Distributed Deep Learning for RIS Aided UAV-D2D Communications in Space-Air-Ground Networks
2023 IEEE/CIC International Conference on Communications in China (ICCC)(2023)
摘要
Due to the flexibility, utility, and the spectrum sharing capability, unmanned aerial vehicle (UAV)-enabled device-to-device (D2D) communications play an important role in the space-airground integrated network (SAGIN). To further improve the communication quality and the system performance, the reconfigurable intelligent surface (RIS) can also be employed. In this paper, we consider a RIS-aided UAV-D2D communication system. A joint optimization problem of the channel selection as well as the transmit power design of D2D users, and the discrete phase configuration of RIS is proposed to maximize the sum rate of D2D users. For efficiently solving this problem, a distributed deep learning (DL) algorithm based on deep neural networks (DNNs) is proposed, which can learn the optimal encoding strategy with only the local channel state information (CSI). Different from the previous way of training DNNs, the penalty terms are added to the loss function when training networks to improve accuracy. Moreover, the proposed DNN networks that have been trained enough to reach a converged state can adaptively derive an optimized strategy for the complex environment of SAGIN with low computational complexity. The simulation results show that the proposed algorithm is able to reach a solution closer to the optimal one than the baseline schemes.
更多查看译文
关键词
Space-air-ground integrated networks,device-to-device communications,reconfigurable intelligent surface,deep learning
AI 理解论文
溯源树
样例
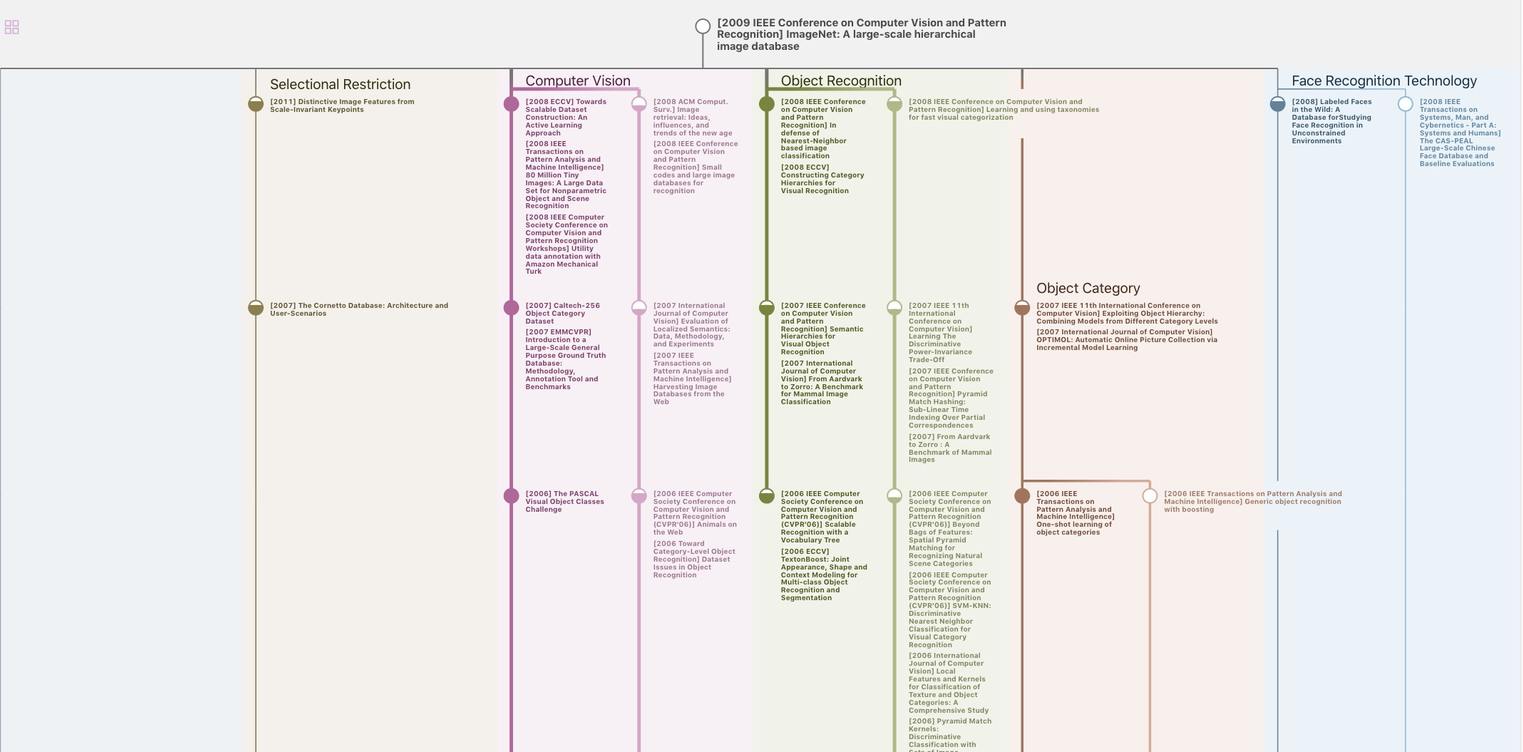
生成溯源树,研究论文发展脉络
Chat Paper
正在生成论文摘要