A Deep Learning-Based No-Reference Quality Metric for High-Definition Images Compressed With HEVC
IEEE Transactions on Broadcasting(2023)
摘要
An accurate no-reference image quality assessment metric for compression artifacts is essential for the broadcasting and streaming industries. Although we have witnessed impressive advances in the capturing, delivery and display technologies, we have not managed to match them with an accurate and perceptual based no-reference image quality metric. In this paper, we propose a unique perceptual based no-reference quality metric for compressed HD frames/images that is based on the DenseNet network architecture. We focus on the effect HEVC (High Efficiency Video Coding) compression artifacts have on the visual quality of a broadcasted and streamed video, as this is a requirement of immense importance for these industries. We chose the Video Multi-Method Assessment Fusion (VMAF) metric as our base measure to map visual quality of HEVC compression artifacts to five visual quality levels. The original VMAF classification was changed to reflect High Definition (HD) resolution images. We trained a DenseNet network to classify compressed images into five visual categories using the dataset generated by the modified VMAF. DenseNet was chosen for its ability to process HD images. Our evaluations have shown that our no-reference metric achieves an impressive average accuracy of 94.13% in classifying the visual quality of compressed images.
更多查看译文
关键词
quality,learning-based,no-reference,high-definition
AI 理解论文
溯源树
样例
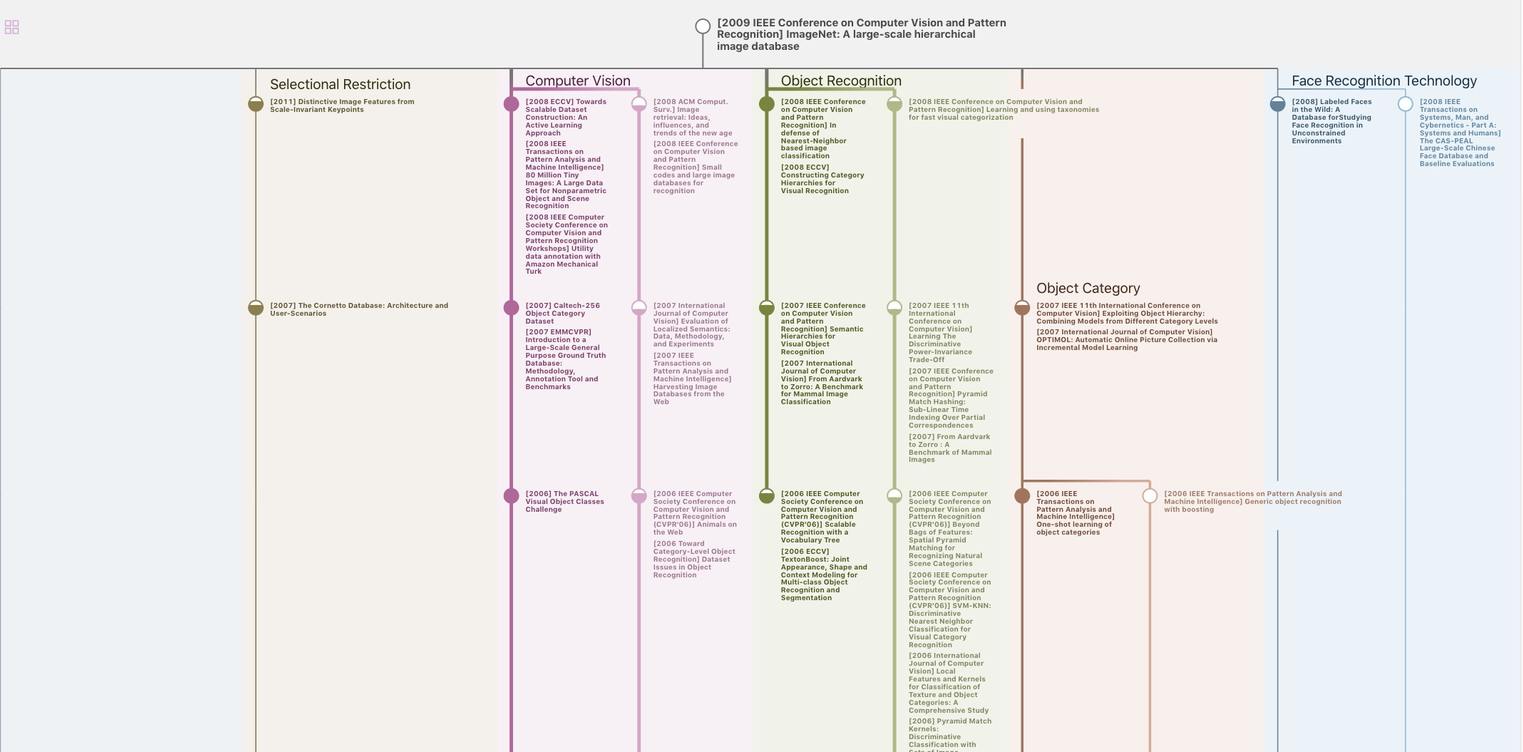
生成溯源树,研究论文发展脉络
Chat Paper
正在生成论文摘要