SAN: Side Adapter Network for Open-Vocabulary Semantic Segmentation
IEEE TRANSACTIONS ON PATTERN ANALYSIS AND MACHINE INTELLIGENCE(2023)
摘要
This article concentrates on open-vocabulary semantic segmentation, where a well optimized model is able to segment arbitrary categories that appear in an image. To achieve this goal, we present a novel framework termed Side Adapter Network, or SAN for short. Our design principles are three-fold: 1) Recent large-scale vision-language models (e.g. CLIP) show promising open-vocabulary image classification capability; it is training-economized to adapt a pre-trained CLIP model to open-vocabulary semantic segmentation. 2) Our SAN model should be both lightweight and effective in order to reduce the inference cost-to achieve this, we fuse the CLIP model's intermediate features to enhance the representation capability of the SAN model, and drive the CLIP model to focus on the informative areas of an image with the aid of the attention biases predicted by a side adapter network. 3) Our approach should empower mainstream segmentation architectures to have the capability of open-vocabulary segmentation-we present P-SAN and R-SAN, to support widely adopted pixel-wise segmentation and region-wise segmentation, respectively. Experimentally, our approach achieves state-of-the-art performance on 5 commonly used benchmarks while having much less trainable parameters and GFLOPs. For instance, our R-SAN outperforms previous best method OvSeg by +2.3 averaged mIoU across all benchmarks while using only 6% of trainable parameters and less than 1% of GFLOPs. In addition, we also conduct a comprehensive analysis of the open-vocabulary semantic segmentation datasets and verify the feasibility of transferring a well optimzied R-SAN model to video segmentation task.
更多查看译文
关键词
Adaptation models,Semantic segmentation,Predictive models,Proposals,Task analysis,Generators,Benchmark testing,Large-scale vision-language model,open-vocabulary semantic segmentation
AI 理解论文
溯源树
样例
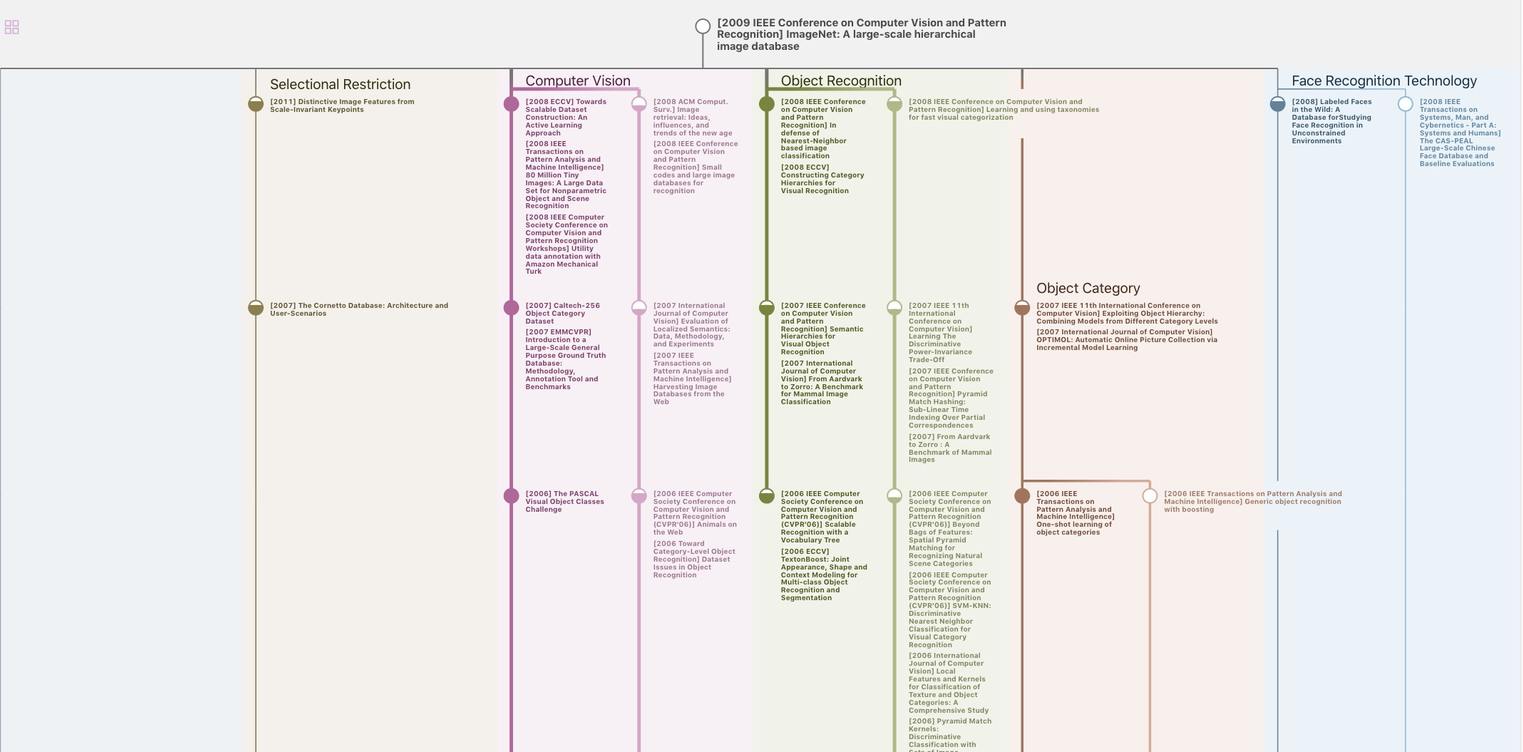
生成溯源树,研究论文发展脉络
Chat Paper
正在生成论文摘要