Explaining grokking through circuit efficiency
CoRR(2023)
摘要
One of the most surprising puzzles in neural network generalisation is grokking: a network with perfect training accuracy but poor generalisation will, upon further training, transition to perfect generalisation. We propose that grokking occurs when the task admits a generalising solution and a memorising solution, where the generalising solution is slower to learn but more efficient, producing larger logits with the same parameter norm. We hypothesise that memorising circuits become more inefficient with larger training datasets while generalising circuits do not, suggesting there is a critical dataset size at which memorisation and generalisation are equally efficient. We make and confirm four novel predictions about grokking, providing significant evidence in favour of our explanation. Most strikingly, we demonstrate two novel and surprising behaviours: ungrokking, in which a network regresses from perfect to low test accuracy, and semi-grokking, in which a network shows delayed generalisation to partial rather than perfect test accuracy.
更多查看译文
关键词
efficiency,circuit
AI 理解论文
溯源树
样例
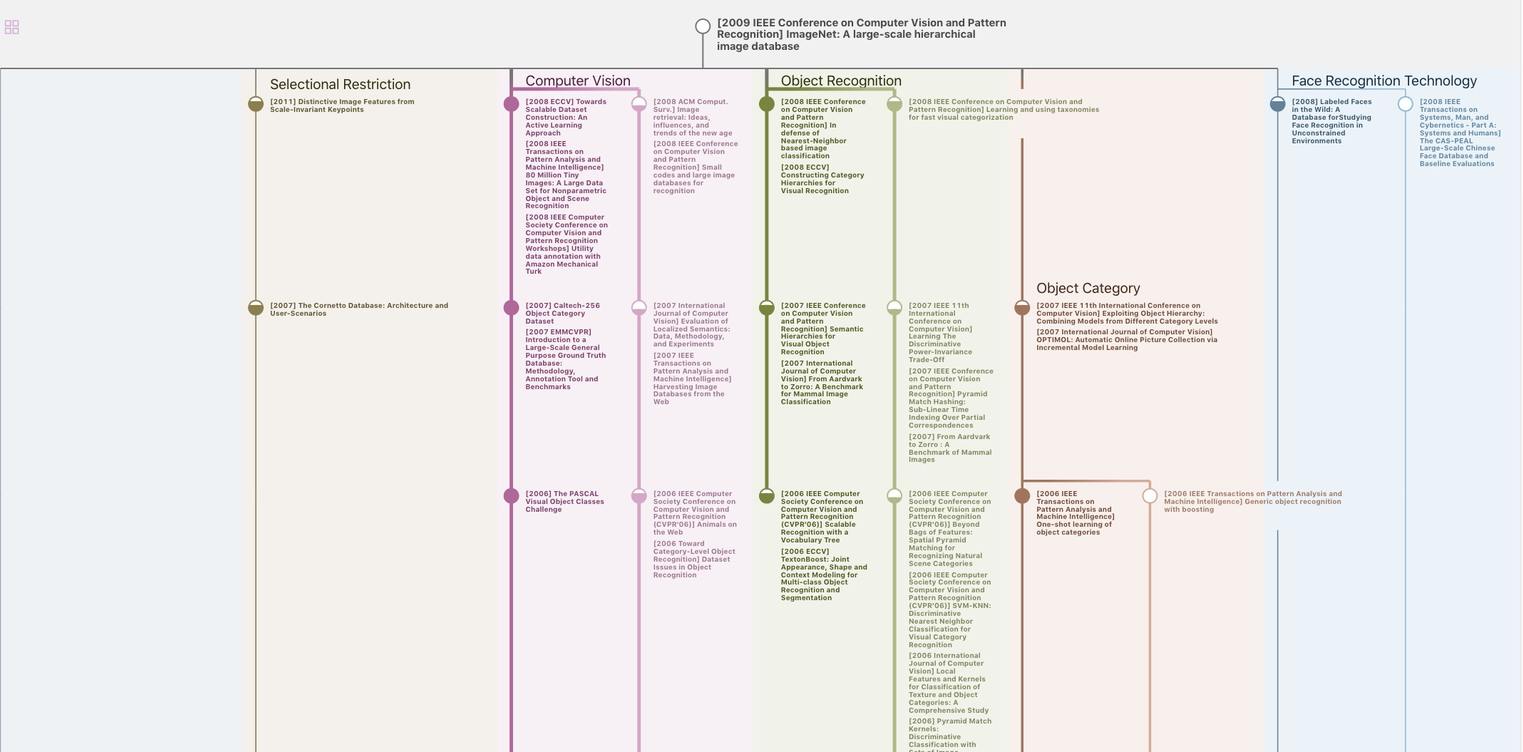
生成溯源树,研究论文发展脉络
Chat Paper
正在生成论文摘要