The impact of electronic health records (EHR) data continuity on prediction model fairness and racial-ethnic disparities
arXiv (Cornell University)(2023)
摘要
Electronic health records (EHR) data have considerable variability in data completeness across sites and patients. Lack of "EHR data-continuity" or "EHR data-discontinuity", defined as "having medical information recorded outside the reach of an EHR system" can lead to a substantial amount of information bias. The objective of this study was to comprehensively evaluate (1) how EHR data-discontinuity introduces data bias, (2) case finding algorithms affect downstream prediction models, and (3) how algorithmic fairness is associated with racial-ethnic disparities. We leveraged our EHRs linked with Medicaid and Medicare claims data in the OneFlorida+ network and used a validated measure (i.e., Mean Proportions of Encounters Captured [MPEC]) to estimate patients' EHR data continuity. We developed a machine learning model for predicting type 2 diabetes (T2D) diagnosis as the use case for this work. We found that using cohorts selected by different levels of EHR data-continuity affects utilities in disease prediction tasks. The prediction models trained on high continuity data will have a worse fit on low continuity data. We also found variations in racial and ethnic disparities in model performances and model fairness in models developed using different degrees of data continuity. Our results suggest that careful evaluation of data continuity is critical to improving the validity of real-world evidence generated by EHR data and health equity.
更多查看译文
关键词
prediction model fairness,electronic health records,data continuity,disparities,ehr,racial-ethnic
AI 理解论文
溯源树
样例
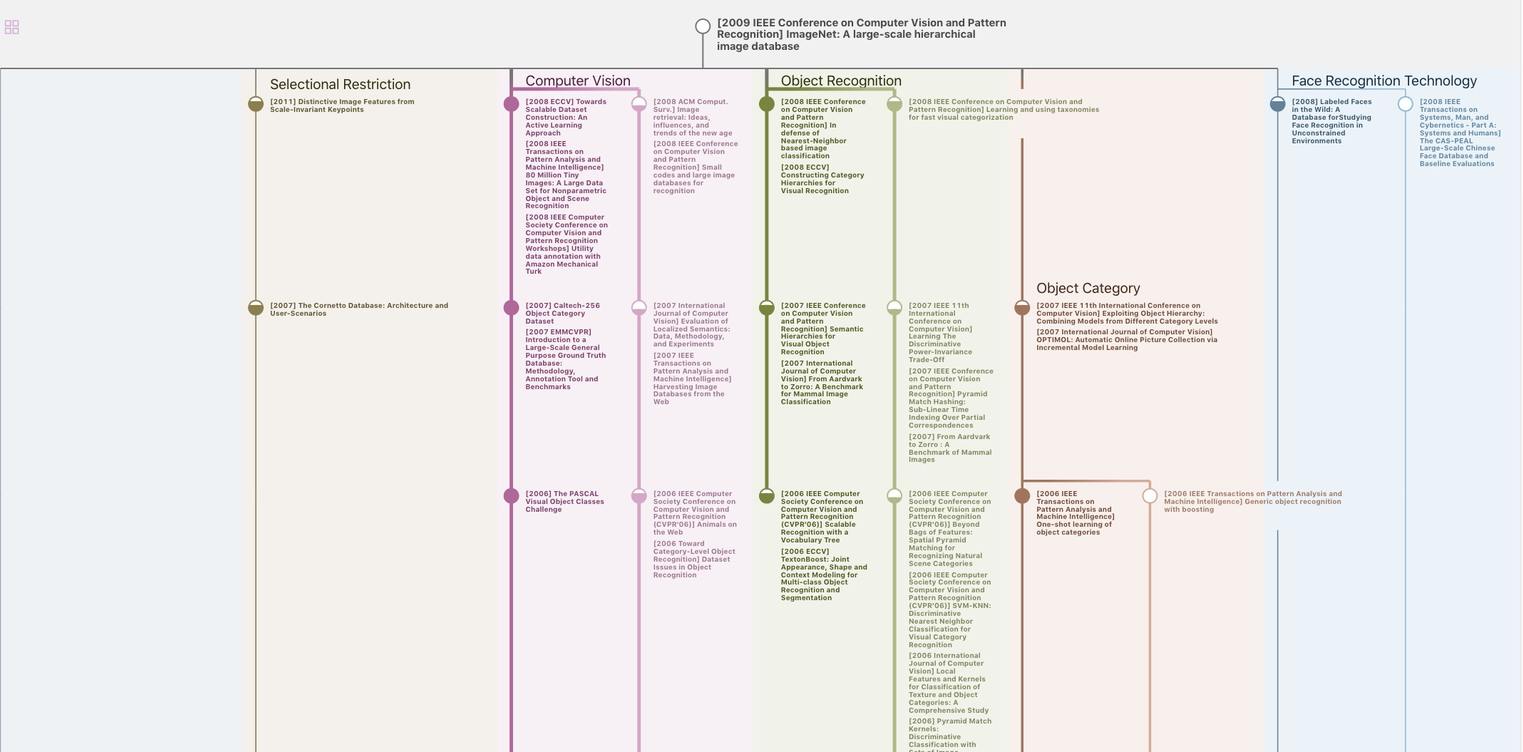
生成溯源树,研究论文发展脉络
Chat Paper
正在生成论文摘要