Categorising the world into local climate zones: towards quantifying labelling uncertainty for machine learning models
JOURNAL OF THE ROYAL STATISTICAL SOCIETY SERIES C-APPLIED STATISTICS(2024)
摘要
Image classification is often prone to labelling uncertainty. To generate suitable training data, images are labelled according to evaluations of human experts. This can result in ambiguities, which will affect subsequent models. In this work, we aim to model the labelling uncertainty in the context of remote sensing and the classification of satellite images. We construct a multinomial mixture model given the evaluations of multiple experts. This is based on the assumption that there is no ambiguity of the image class, but apparently in the experts' opinion about it. The model parameters can be estimated by a stochastic expectation maximisation algorithm. Analysing the estimates gives insights into sources of label uncertainty. Here, we focus on the general class ambiguity, the heterogeneity of experts, and the origin city of the images. The results are relevant for all machine learning applications where image classification is pursued and labelling is subject to humans.
更多查看译文
关键词
expert evaluations,labelling uncertainty,mixture models,multiple labellers,stochastic expectation maximisation
AI 理解论文
溯源树
样例
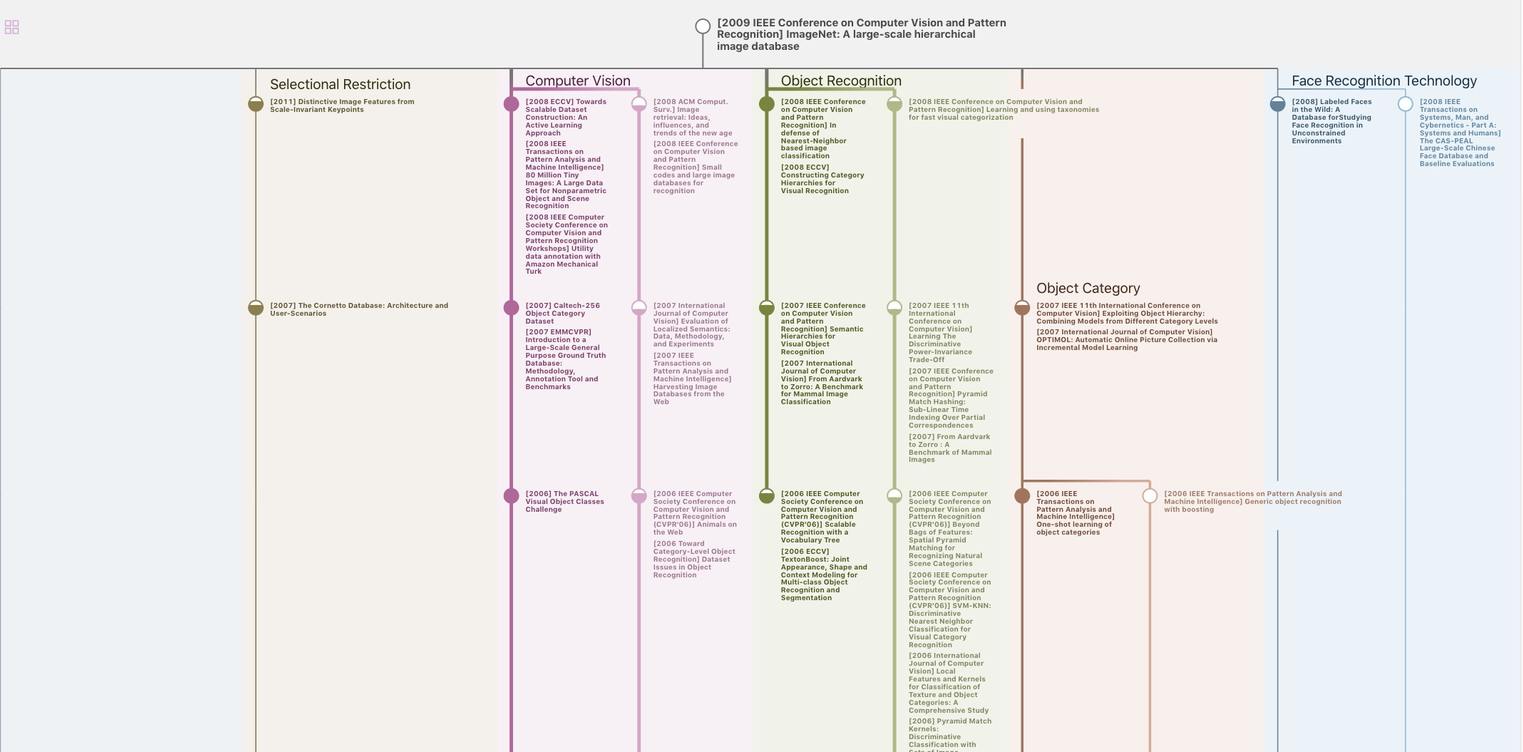
生成溯源树,研究论文发展脉络
Chat Paper
正在生成论文摘要