ReOnto: A Neuro-Symbolic Approach for Biomedical Relation Extraction
MACHINE LEARNING AND KNOWLEDGE DISCOVERY IN DATABASES: RESEARCH TRACK, ECML PKDD 2023, PT IV(2023)
摘要
Relation Extraction (RE) is the task of extracting semantic relationships between entities in a sentence and aligning them to relations defined in a vocabulary, which is generally in the form of a Knowledge Graph (KG) or an ontology. Various approaches have been proposed so far to address this task. However, applying these techniques to biomedical text often yields unsatisfactory results because it is hard to infer relations directly from sentences due to the nature of the biomedical relations. To address these issues, we present a novel technique called ReOnto, that makes use of neuro symbolic knowledge for the RE task. ReOnto employs a graph neural network to acquire the sentence representation and leverages publicly accessible ontologies as prior knowledge to identify the sentential relation between two entities. The approach involves extracting the relation path between the two entities from the ontology. We evaluate the effect of using symbolic knowledge from ontologies with graph neural networks. Experimental results on two public biomedical datasets, BioRel and ADE, show that our method outperforms all the baselines (approximately by 3%).
更多查看译文
关键词
ontology,neuro-symbolic integration,graph neural network,domain knowledge,relation extraction
AI 理解论文
溯源树
样例
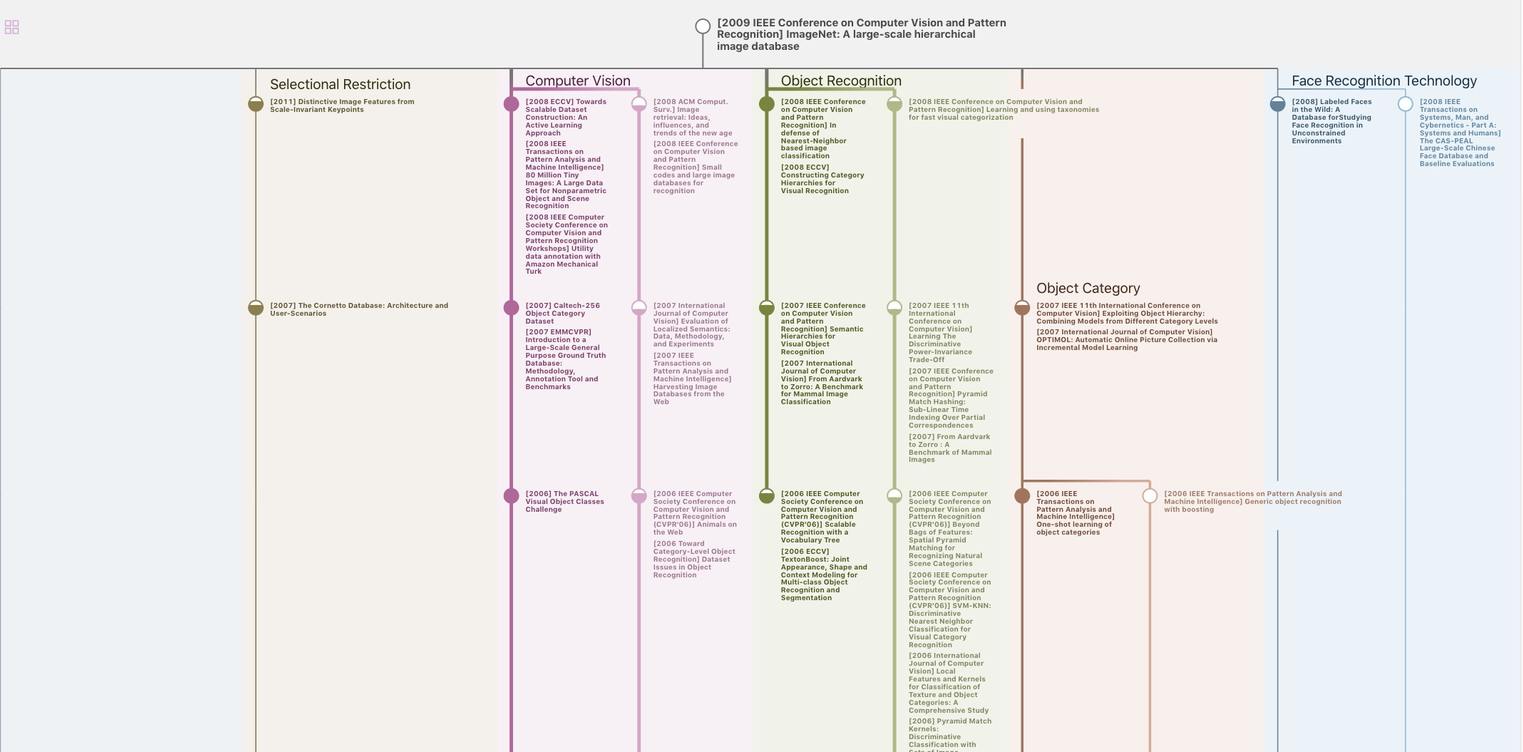
生成溯源树,研究论文发展脉络
Chat Paper
正在生成论文摘要