Distributional Domain -Invariant Preference Matching for Cross -Domain Recommendation
23RD IEEE INTERNATIONAL CONFERENCE ON DATA MINING, ICDM 2023(2023)
摘要
Learning accurate cross -domain preference mappings in the absence of overlapped users/items has presented a persistent challenge in Non-overlapping Cross-domain Recommendation (NOCDR). Despite the efforts made in previous studies to address NOCDR, several limitations still exist. Specifically, 1) while some approaches substitute overlapping users/items with overlapping behaviors, they cannot handle NOCDR scenarios where such auxiliary information is unavailable; 2) often, cross domain preference mapping is modeled by learning deterministic explicit representation matchings between sampled users in two domains. However, this can be biased due to individual preferences and thus fails to incorporate preference continuity and universality of the general population. In light of this, we assume that despite the scattered nature of user behaviors, there exists a consistent latent preference distribution shared among common people. Modeling such distributions further allows us to capture the continuity in user behaviors within each domain and discover preference invariance across domains. To this end, we propose a Distributional domain-invariant Preference Matching method for non-overlapping Cross-Domain Recommendation (DPMCDR). For each domain, we hierarchically approximate a posterior of domain-level preference distribution with empirical evidence derived from user-item interactions. Next, we aim to build distributional implicit matchings between the domain-level preferences of two domains. This process involves mapping them to a shared latent space and seeking a consensus on domain invariant preference by minimizing the distance between their distributional representations therein. In this way, we can identify the alignment of two non-overlapping domains if they exhibit similar patterns of domain-invariant preference. Experiments on real-world datasets demonstrate that DPMCDR outperforms the state-of-the-art approaches with a range of evaluation metrics.
更多查看译文
关键词
Cross-Domain Recommendation,Distributional Preference Matching,Preference Invariance
AI 理解论文
溯源树
样例
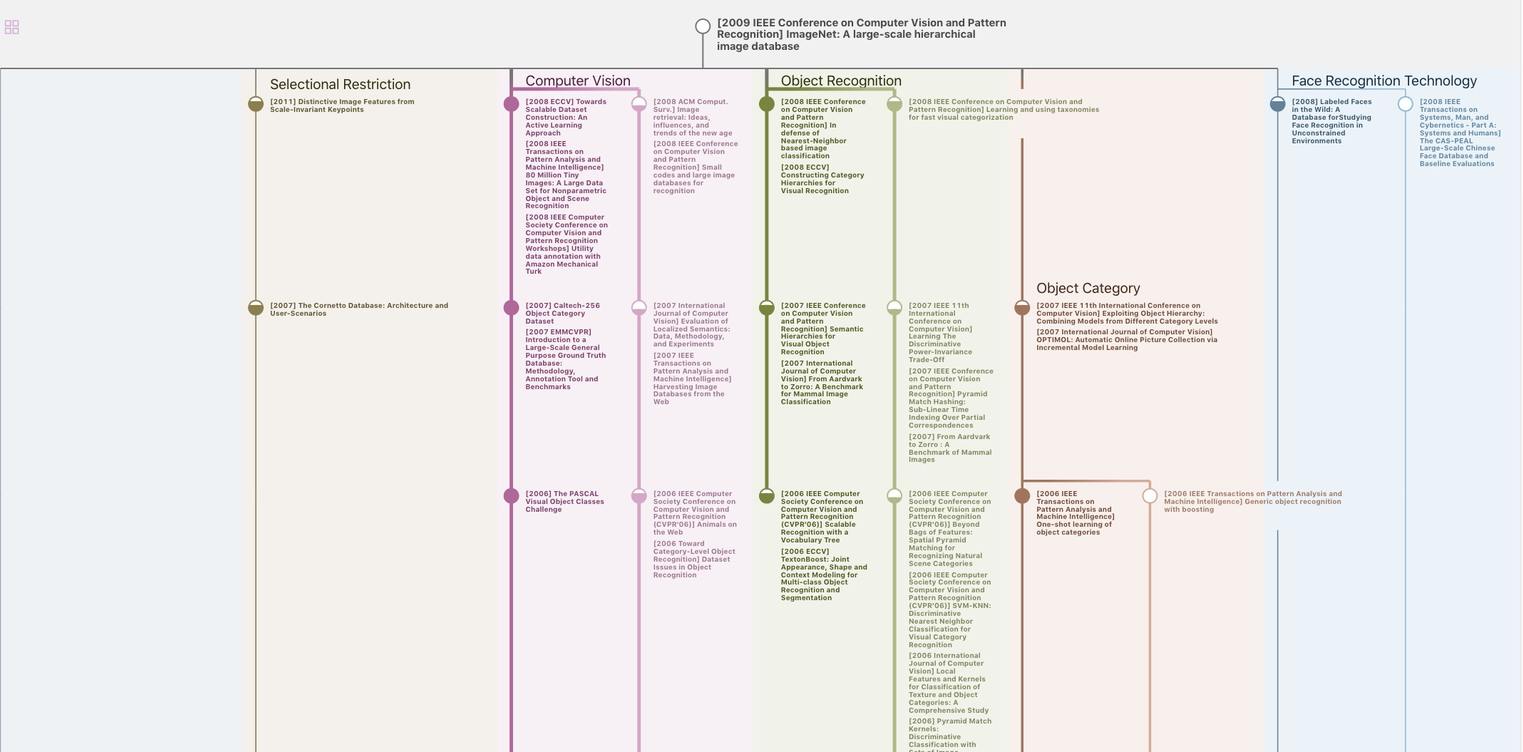
生成溯源树,研究论文发展脉络
Chat Paper
正在生成论文摘要