BDC-Adapter: Brownian Distance Covariance for Better Vision-Language Reasoning
CoRR(2023)
摘要
Large-scale pre-trained Vision-Language Models (VLMs), such as CLIP and ALIGN, have introduced a new paradigm for learning transferable visual representations. Recently, there has been a surge of interest among researchers in developing lightweight fine-tuning techniques to adapt these models to downstream visual tasks. We recognize that current state-of-the-art fine-tuning methods, such as Tip-Adapter, simply consider the covariance between the query image feature and features of support few-shot training samples, which only captures linear relations and potentially instigates a deceptive perception of independence. To address this issue, in this work, we innovatively introduce Brownian Distance Covariance (BDC) to the field of vision-language reasoning. The BDC metric can model all possible relations, providing a robust metric for measuring feature dependence. Based on this, we present a novel method called BDC-Adapter, which integrates BDC prototype similarity reasoning and multi-modal reasoning network prediction to perform classification tasks. Our extensive experimental results show that the proposed BDC-Adapter can freely handle non-linear relations and fully characterize independence, outperforming the current state-of-the-art methods by large margins.
更多查看译文
关键词
brownian distance covariance,bdc-adapter,vision-language
AI 理解论文
溯源树
样例
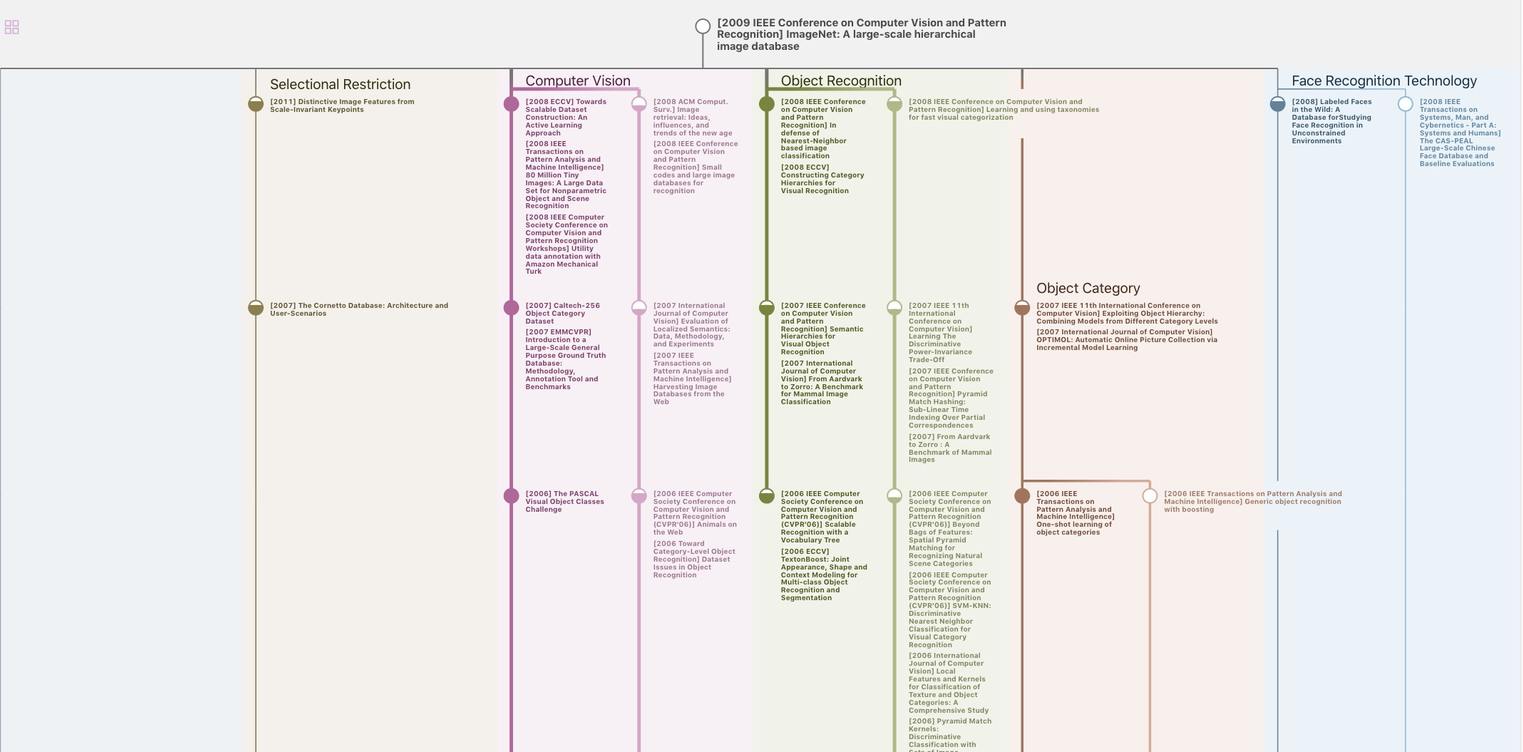
生成溯源树,研究论文发展脉络
Chat Paper
正在生成论文摘要