M2HGCL: Multi-Scale Meta-Path Integrated Heterogeneous Graph Contrastive Learning
CoRR(2023)
摘要
Inspired by the successful application of contrastive learning on graphs, researchers attempt to impose graph contrastive learning approaches on heterogeneous information networks. Orthogonal to homogeneous graphs, the types of nodes and edges in heterogeneous graphs are diverse so that specialized graph contrastive learning methods are required. Most existing methods for heterogeneous graph contrastive learning are implemented by transforming heterogeneous graphs into homogeneous graphs, which may lead to ramifications that the valuable information carried by non-target nodes is undermined thereby exacerbating the performance of contrastive learning models. Additionally, current heterogeneous graph contrastive learning methods are mainly based on initial meta-paths given by the dataset, yet according to our deep-going exploration, we derive empirical conclusions: only initial meta-paths cannot contain sufficiently discriminative information; and various types of meta-paths can effectively promote the performance of heterogeneous graph contrastive learning methods. To this end, we propose a new multi-scale meta-path integrated heterogeneous graph contrastive learning (M2HGCL) model, which discards the conventional heterogeneity-homogeneity transformation and performs the graph contrastive learning in a joint manner. Specifically, we expand the meta-paths and jointly aggregate the direct neighbor information, the initial meta-path neighbor information and the expanded meta-path neighbor information to sufficiently capture discriminative information. A specific positive sampling strategy is further imposed to remedy the intrinsic deficiency of contrastive learning, i.e., the hard negative sample sampling issue. Through extensive experiments on three real-world datasets, we demonstrate that M2HGCL outperforms the current state-of-the-art baseline models.
更多查看译文
关键词
contrastive learning,graph,multi-scale,meta-path
AI 理解论文
溯源树
样例
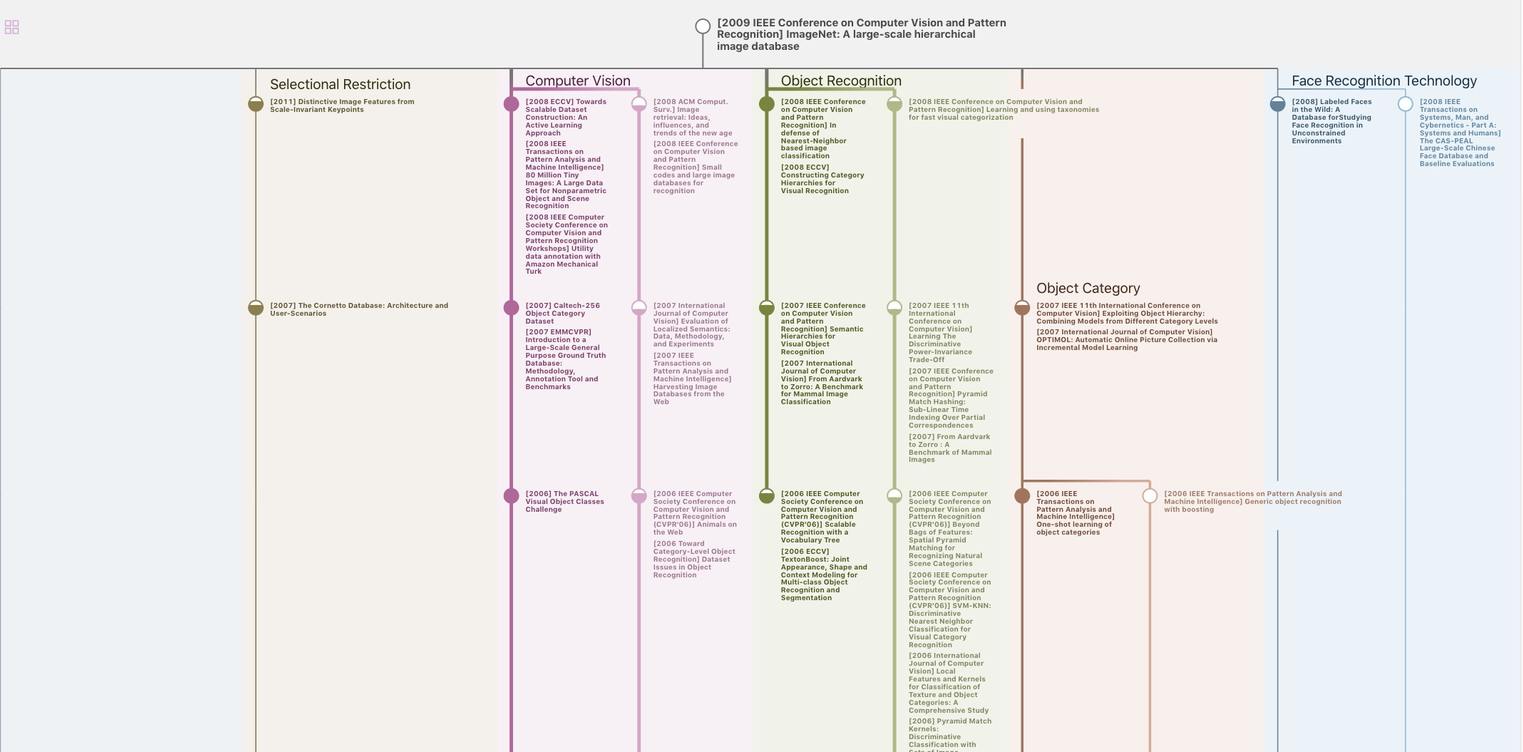
生成溯源树,研究论文发展脉络
Chat Paper
正在生成论文摘要