NTU4DRadLM: 4D Radar-centric Multi-Modal Dataset for Localization and Mapping
CoRR(2023)
摘要
Simultaneous Localization and Mapping (SLAM) is moving towards a robust perception age. However, LiDAR- and visual- SLAM may easily fail in adverse conditions (rain, snow, smoke and fog, etc.). In comparison, SLAM based on 4D Radar, thermal camera and IMU can work robustly. But only a few literature can be found. A major reason is the lack of related datasets, which seriously hinders the research. Even though some datasets are proposed based on 4D radar in past four years, they are mainly designed for object detection, rather than SLAM. Furthermore, they normally do not include thermal camera. Therefore, in this paper, NTU4DRadLM is presented to meet this requirement. The main characteristics are: 1) It is the only dataset that simultaneously includes all 6 sensors: 4D radar, thermal camera, IMU, 3D LiDAR, visual camera and RTK GPS. 2) Specifically designed for SLAM tasks, which provides fine-tuned ground truth odometry and intentionally formulated loop closures. 3) Considered both low-speed robot platform and fast-speed unmanned vehicle platform. 4) Covered structured, unstructured and semi-structured environments. 5) Considered both middle- and large- scale outdoor environments, i.e., the 6 trajectories range from 246m to 6.95km. 6) Comprehensively evaluated three types of SLAM algorithms. Totally, the dataset is around 17.6km, 85mins, 50GB and it will be accessible from this link: https://github.com/junzhang2016/NTU4DRadLM
更多查看译文
关键词
Infrared Imaging,Adverse Conditions,Types Of Algorithms,Lack Of Datasets,Simultaneous Localization And Mapping,Visible Camera,Loop Closure,Vehicle Platform,3D LiDAR,Point Cloud,Transformation Matrix,Unmanned Aerial Vehicles,Gyroscope,Mobile Robot,Intrinsic Parameters,Trajectory Length,Unstructured Environments,Extrinsic Parameters,Planar Features,Large-scale Environments,Graph Optimization,LiDAR Point Clouds
AI 理解论文
溯源树
样例
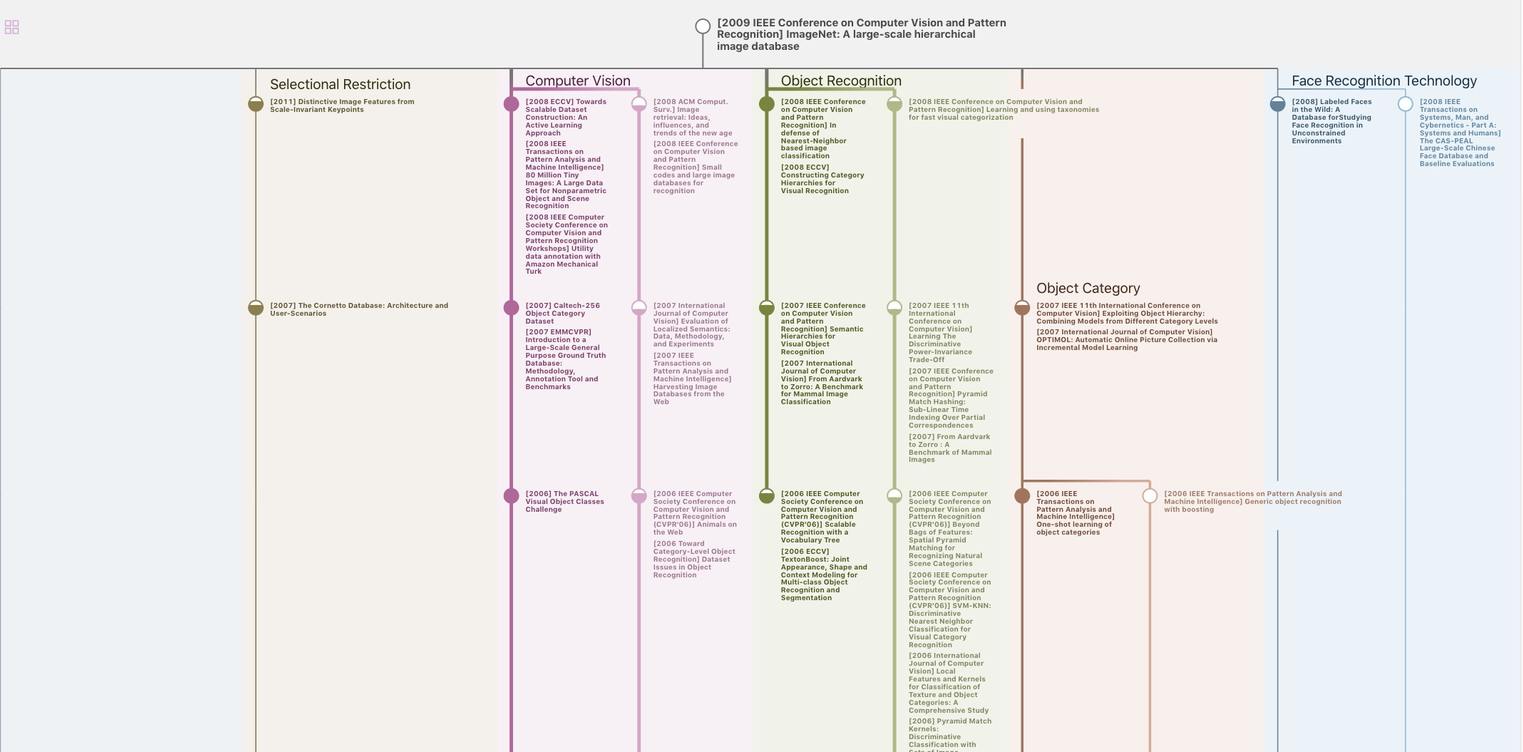
生成溯源树,研究论文发展脉络
Chat Paper
正在生成论文摘要