Network Topology Inference with Sparsity and Laplacian Constraints
CoRR(2023)
摘要
We tackle the network topology inference problem by utilizing Laplacian constrained Gaussian graphical models, which recast the task as estimating a precision matrix in the form of a graph Laplacian. Recent research \cite{ying2020nonconvex} has uncovered the limitations of the widely used $\ell_1$-norm in learning sparse graphs under this model: empirically, the number of nonzero entries in the solution grows with the regularization parameter of the $\ell_1$-norm; theoretically, a large regularization parameter leads to a fully connected (densest) graph. To overcome these challenges, we propose a graph Laplacian estimation method incorporating the $\ell_0$-norm constraint. An efficient gradient projection algorithm is developed to solve the resulting optimization problem, characterized by sparsity and Laplacian constraints. Through numerical experiments with synthetic and financial time-series datasets, we demonstrate the effectiveness of the proposed method in network topology inference.
更多查看译文
关键词
Network topology inference,Graph Laplacian,Gradient projection,Sparsity constraint
AI 理解论文
溯源树
样例
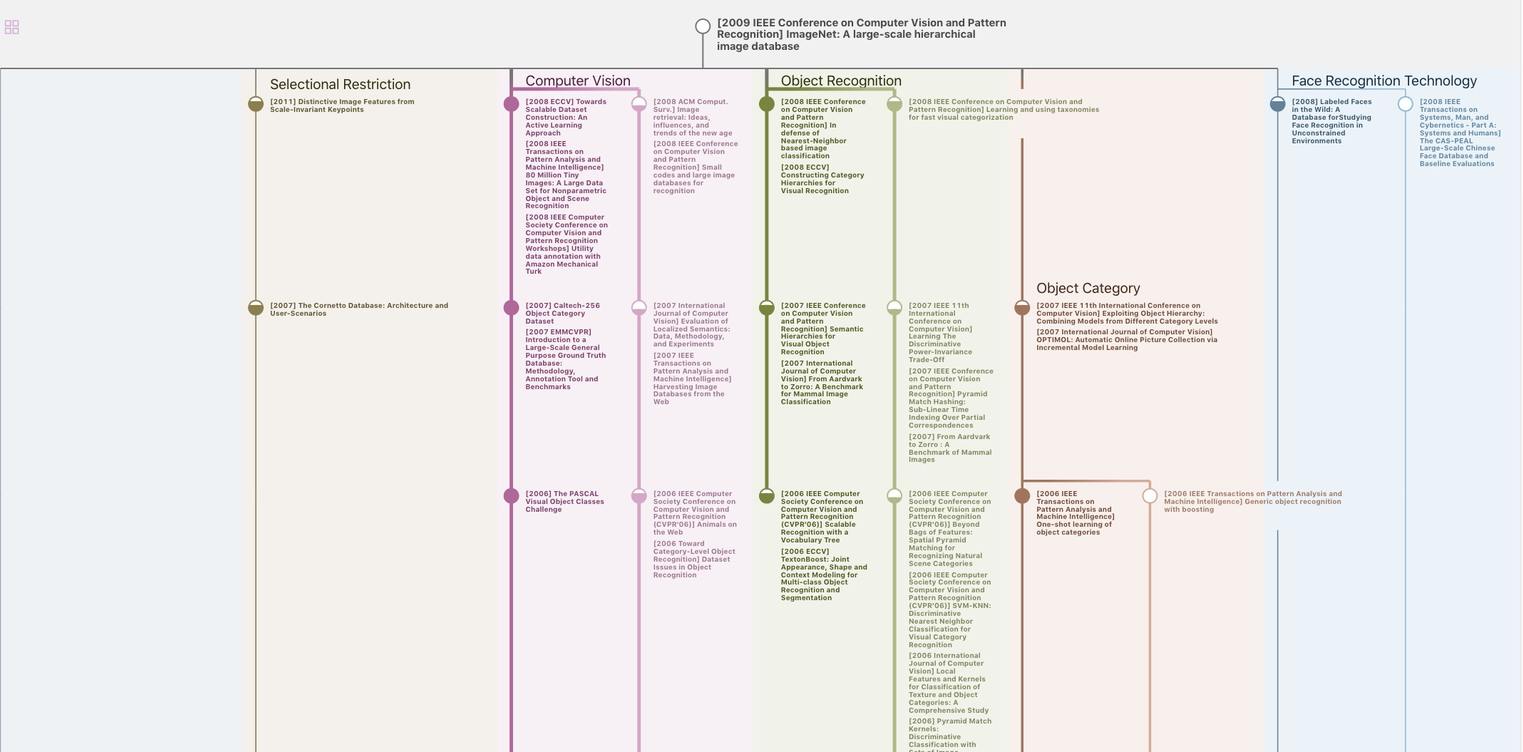
生成溯源树,研究论文发展脉络
Chat Paper
正在生成论文摘要