Full Reference Video Quality Assessment for Machine Learning-Based Video Codecs
CoRR(2023)
摘要
Machine learning-based video codecs have made significant progress in the past few years. A critical area in the development of ML-based video codecs is an accurate evaluation metric that does not require an expensive and slow subjective test. We show that existing evaluation metrics that were designed and trained on DSP-based video codecs are not highly correlated to subjective opinion when used with ML video codecs due to the video artifacts being quite different between ML and video codecs. We provide a new dataset of ML video codec videos that have been accurately labeled for quality. We also propose a new full reference video quality assessment (FRVQA) model that achieves a Pearson Correlation Coefficient (PCC) of 0.99 and a Spearman's Rank Correlation Coefficient (SRCC) of 0.99 at the model level. We make the dataset and FRVQA model open source to help accelerate research in ML video codecs, and so that others can further improve the FRVQA model.
更多查看译文
关键词
quality assessment,video,learning-based
AI 理解论文
溯源树
样例
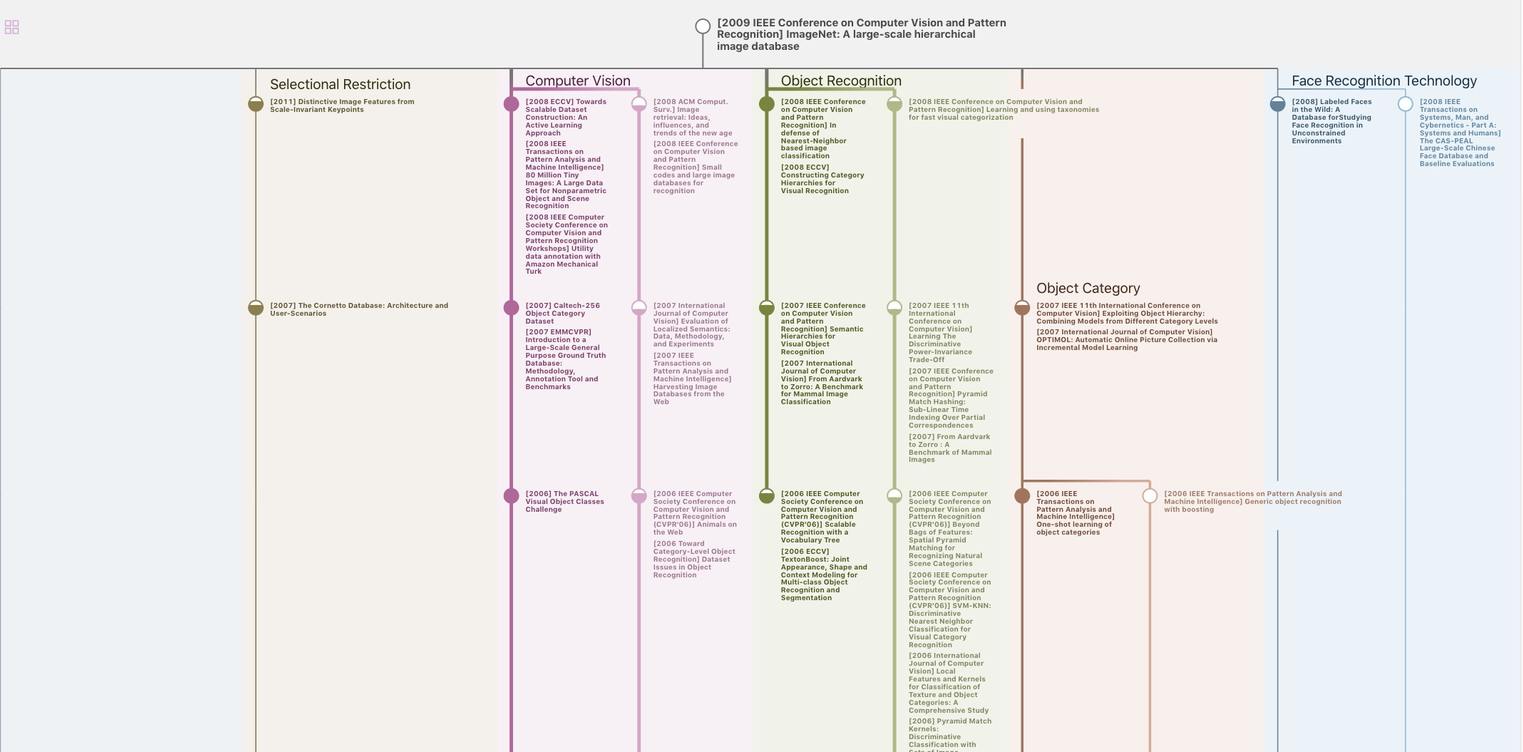
生成溯源树,研究论文发展脉络
Chat Paper
正在生成论文摘要