Affine-Transformation-Invariant Image Classification by Differentiable Arithmetic Distribution Module
CoRR(2023)
摘要
Although Convolutional Neural Networks (CNNs) have achieved promising results in image classification, they still are vulnerable to affine transformations including rotation, translation, flip and shuffle. The drawback motivates us to design a module which can alleviate the impact from different affine transformations. Thus, in this work, we introduce a more robust substitute by incorporating distribution learning techniques, focusing particularly on learning the spatial distribution information of pixels in images. To rectify the issue of non-differentiability of prior distribution learning methods that rely on traditional histograms, we adopt the Kernel Density Estimation (KDE) to formulate differentiable histograms. On this foundation, we present a novel Differentiable Arithmetic Distribution Module (DADM), which is designed to extract the intrinsic probability distributions from images. The proposed approach is able to enhance the model's robustness to affine transformations without sacrificing its feature extraction capabilities, thus bridging the gap between traditional CNNs and distribution-based learning. We validate the effectiveness of the proposed approach through ablation study and comparative experiments with LeNet.
更多查看译文
关键词
arithmetic distribution module,classification,image,affine-transformation-invariant
AI 理解论文
溯源树
样例
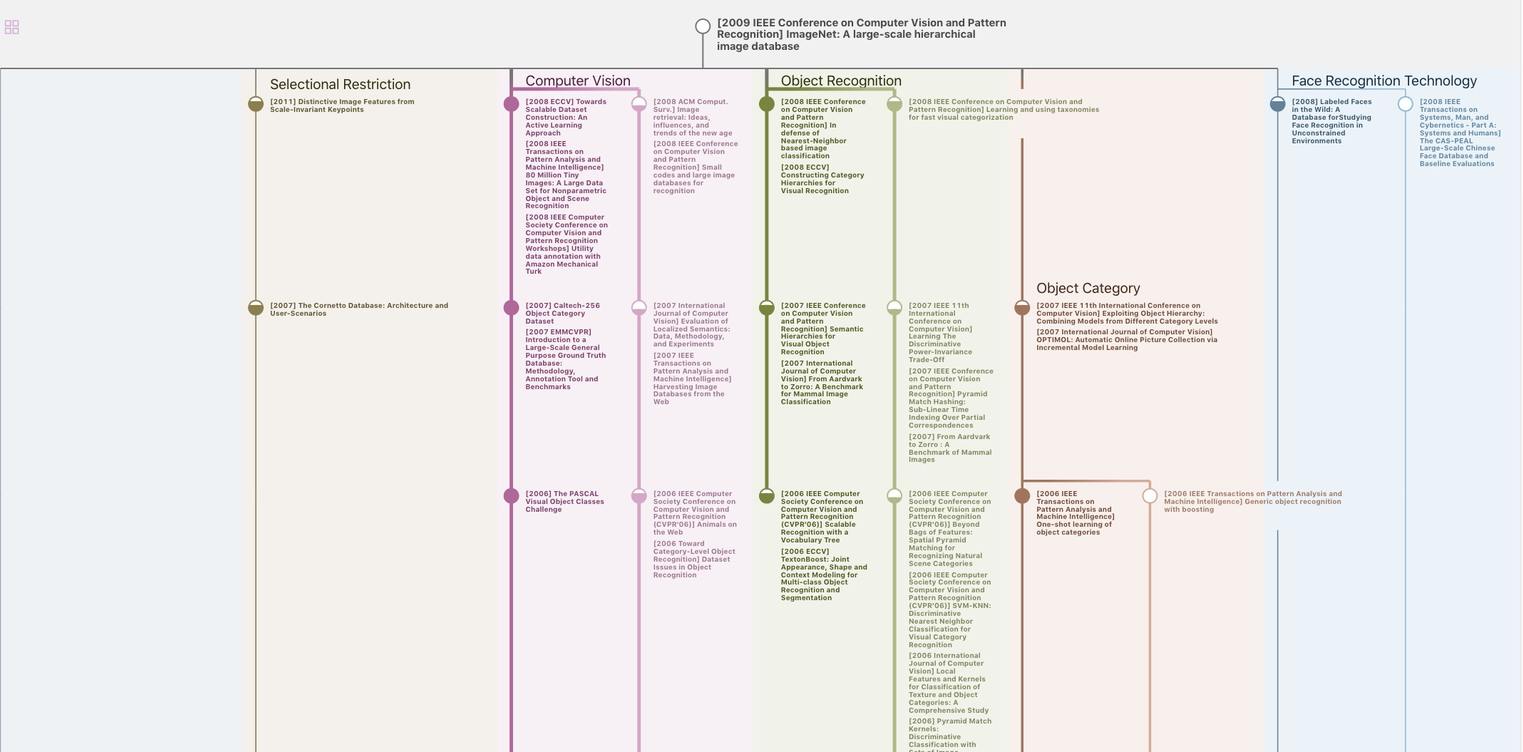
生成溯源树,研究论文发展脉络
Chat Paper
正在生成论文摘要