Information-based Optimal Subdata Selection for Clusterwise Linear Regression
arXiv (Cornell University)(2023)
摘要
Mixture-of-Experts models are commonly used when there exist distinct clusters with different relationships between the independent and dependent variables. Fitting such models for large datasets, however, is computationally virtually impossible. An attractive alternative is to use a subdata selected by ``maximizing" the Fisher information matrix. A major challenge is that no closed-form expression for the Fisher information matrix is available for such models. Focusing on clusterwise linear regression models, a subclass of MoE models, we develop a framework that overcomes this challenge. We prove that the proposed subdata selection approach is asymptotically optimal, i.e., no other method is statistically more efficient than the proposed one when the full data size is large.
更多查看译文
关键词
optimal subdata selection,clusterwise linear
AI 理解论文
溯源树
样例
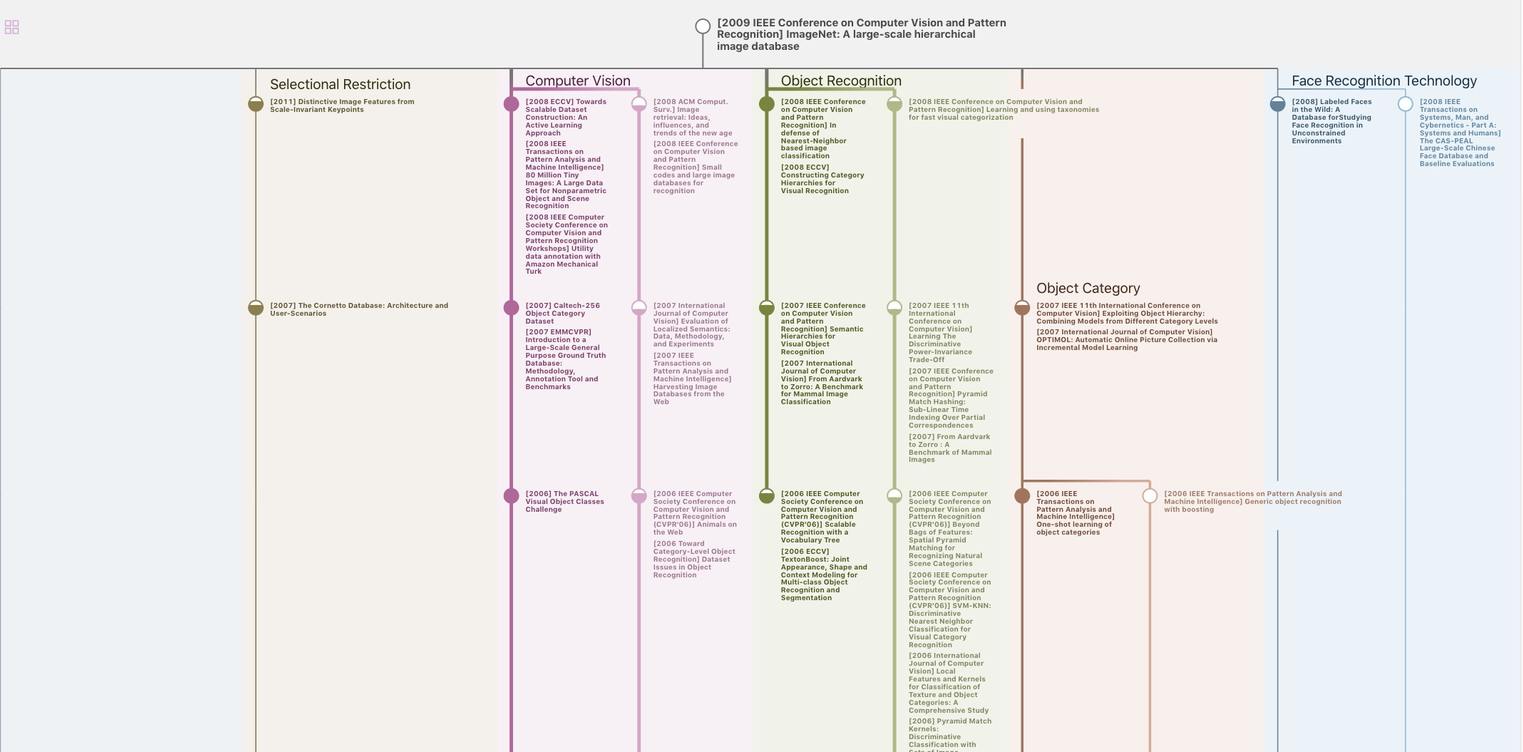
生成溯源树,研究论文发展脉络
Chat Paper
正在生成论文摘要