LEHA: A novel lightweight efficient and highly accurate lane departure warning system
Multimedia Tools and Applications(2024)
摘要
As one of the most indispensable means of transportation in modern society, vehicles guarantee our daily commuting and logistics transportation. However, with the increasing number of vehicles, vehicles have also caused increasingly serious traffic safety problems while providing convenience to our lives. One of the most common of these is traffic accidents caused by vehicle yaw due to driver distraction. As a potential solution to this problem, lane departure warning systems (LDWS) focus on detecting and determining whether the vehicle is deviating from the driveway, considered an essential part of autonomous driving technology, and have received significant attention in recent years. A large number of different types of LDWS systems have been developed, especially in recent years, with the development of artificial intelligence technology, many methods based on deep learning and machine vision have been proposed. However, it is well known that due to the complexity of the network structure in deep learning-based object detection algorithms, the operation of such methods relies on a large amount of computing power support. However, due to the limitation of the overall energy supply of the vehicle, it is usually unable to support computing power similar to the laboratory level. Therefore, how to realize efficient lane departure warnings under the condition of limited computing power is a critical problem to be solved. Accordingly, in this paper, we propose a novel lightweight LDWS model. Different from deep learning methods of LDWS, our LDWS model LEHA can achieve high accuracy and efficiency by relying only on simple hardware. The proposed LEHA consists of three modules: the image processing module, the lane detection module, and the lane departure recognition module. The image pre-processing module is applied to pre-process the original road image, which can improve the accuracy and efficiency of the following lane detection module. After obtaining the processed image, lane detection begins to detect and label the lanes. Finally, lane departure recognition is used to calculate the deviation distance and direction to determine whether the warning should be initiated. To evaluate the performance of LEHA, we compare our method with other state-of-the-art LDWS models in terms of detection accuracy and processing time under ideal and non-ideal lane conditions based on the KITTI dataset. The experimental results demonstrate that our LEHA outperforms state-of-the-art techniques.
更多查看译文
关键词
Land departure warning system,Image preprocessing,Lane detection,Lane departure recognition
AI 理解论文
溯源树
样例
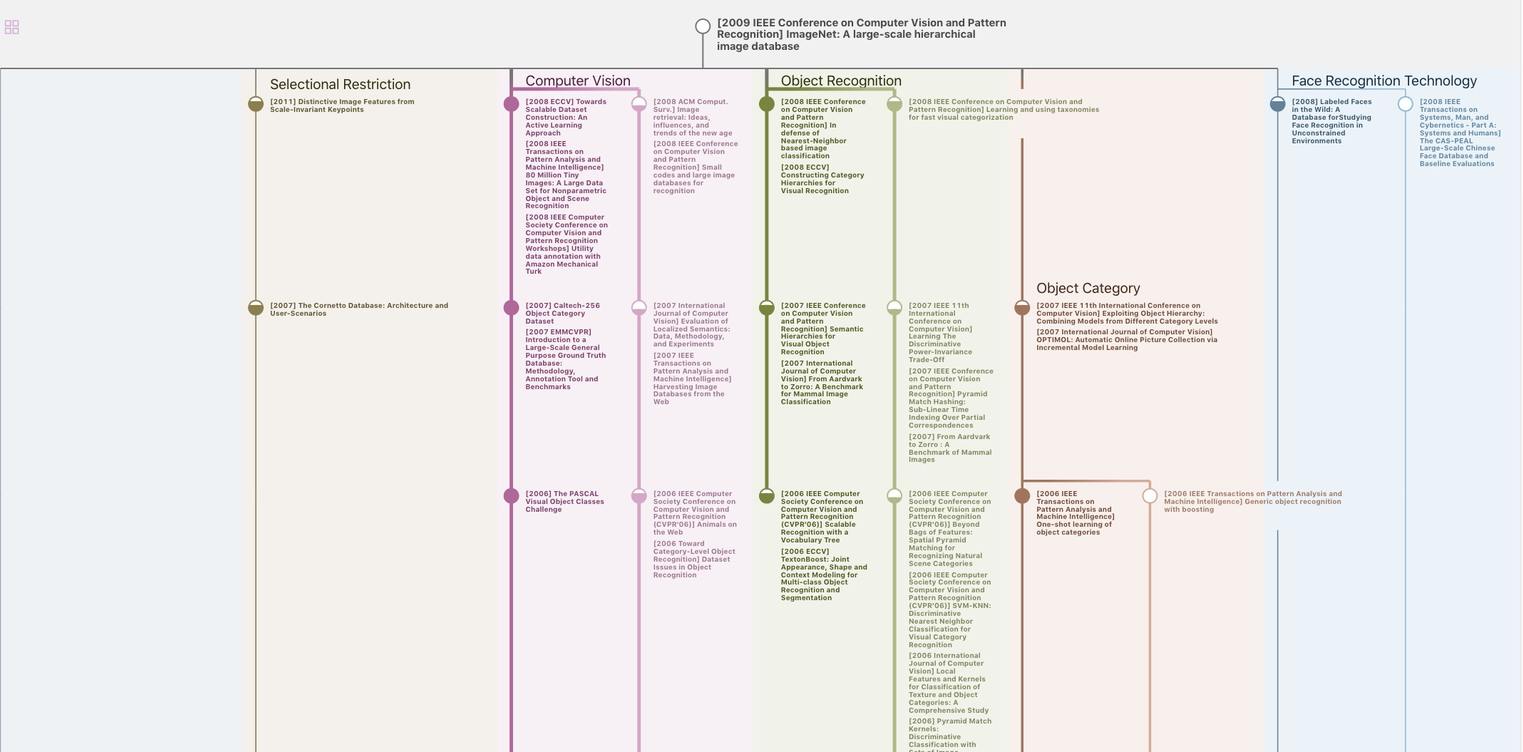
生成溯源树,研究论文发展脉络
Chat Paper
正在生成论文摘要