Deep learning–based segmentation of whole-body fetal MRI and fetal weight estimation: assessing performance, repeatability, and reproducibility
European Radiology(2024)
摘要
Objectives To develop a deep-learning method for whole-body fetal segmentation based on MRI; to assess the method’s repeatability, reproducibility, and accuracy; to create an MRI-based normal fetal weight growth chart; and to assess the sensitivity to detect fetuses with growth restriction (FGR). Methods Retrospective data of 348 fetuses with gestational age (GA) of 19–39 weeks were included: 249 normal appropriate for GA (AGA), 19 FGR, and 80 Other (having various imaging abnormalities). A fetal whole-body segmentation model with a quality estimation module was developed and evaluated in 169 cases. The method was evaluated for its repeatability (repeated scans within the same scanner, n = 22), reproducibility (different scanners, n = 6), and accuracy (compared with birth weight, n = 7). A normal MRI-based growth chart was derived. Results The method achieved a Dice = 0.973, absolute volume difference ratio (VDR) = 1.8
更多查看译文
关键词
Fetal weight,Magnetic resonance imaging,Deep learning,Growth chart,Fetal growth restriction
AI 理解论文
溯源树
样例
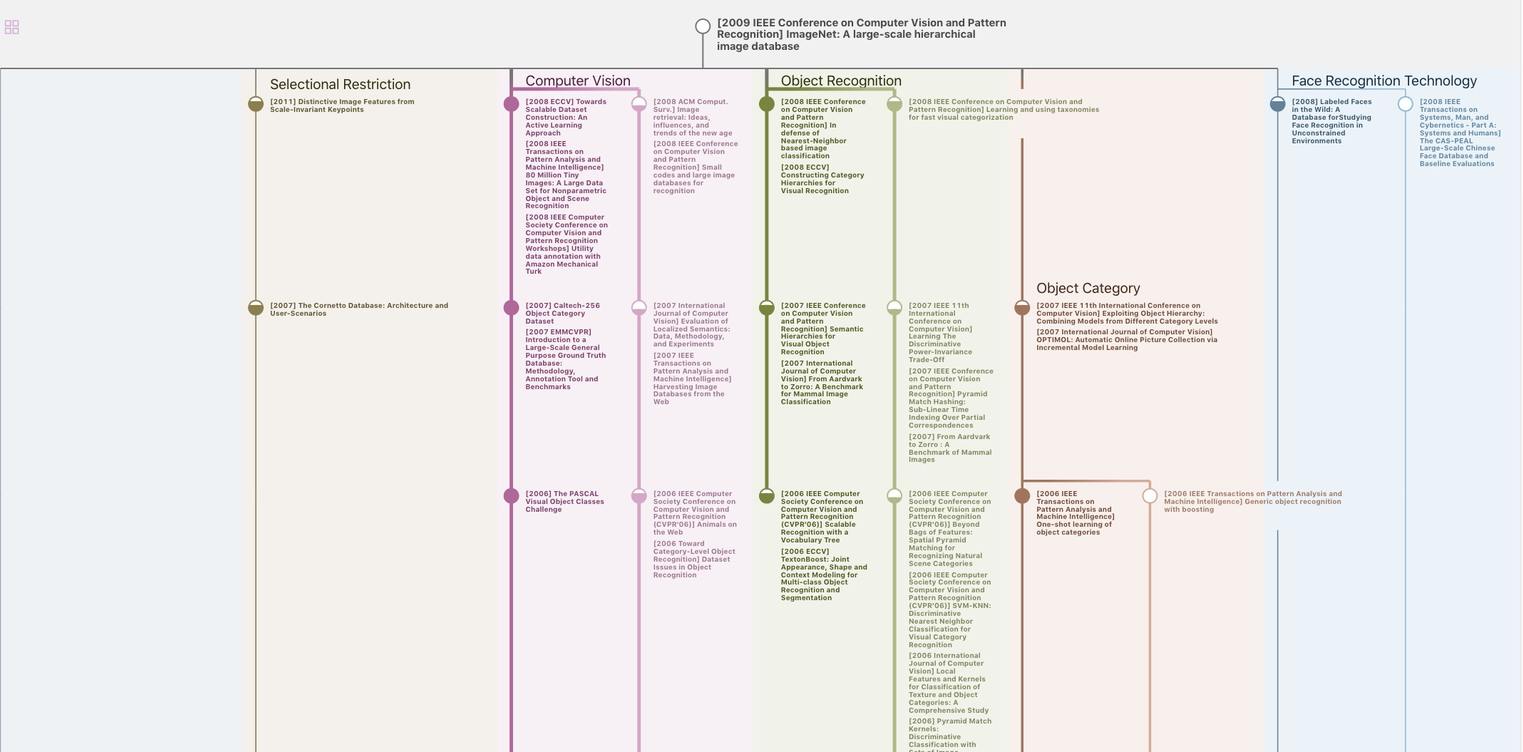
生成溯源树,研究论文发展脉络
Chat Paper
正在生成论文摘要