Stochastic Gradient Descent and Anomaly of Variance-Flatness Relation in Artificial Neural Networks
Chinese Physics Letters(2023)
摘要
Stochastic gradient descent(SGD),a widely used algorithm in deep-learning neural networks,has attracted continuing research interests for the theoretical principles behind its success.A recent work reported an anomaly(inverse)relation between the variance of neural weights and the landscape flatness of the loss function driven under SGD[Feng Y and Tu Y Proc.Natl.Acad.Sci.USA 118 e2015617118(2021)].To investigate this seeming violation of statistical physics principle,the properties of SGD near fixed points are analyzed with a dynamic decomposition method.Our approach recovers the true"energy"function under which the universal Boltzmann distribution holds.It differs from the cost function in general and resolves the paradox raised by the the anomaly.The study bridges the gap between the classical statistical mechanics and the emerging discipline of artificial intelligence,with potential for better algorithms to the latter.
更多查看译文
关键词
stochastic gradient descent,artificial neural networks,neural networks,variance-flatness
AI 理解论文
溯源树
样例
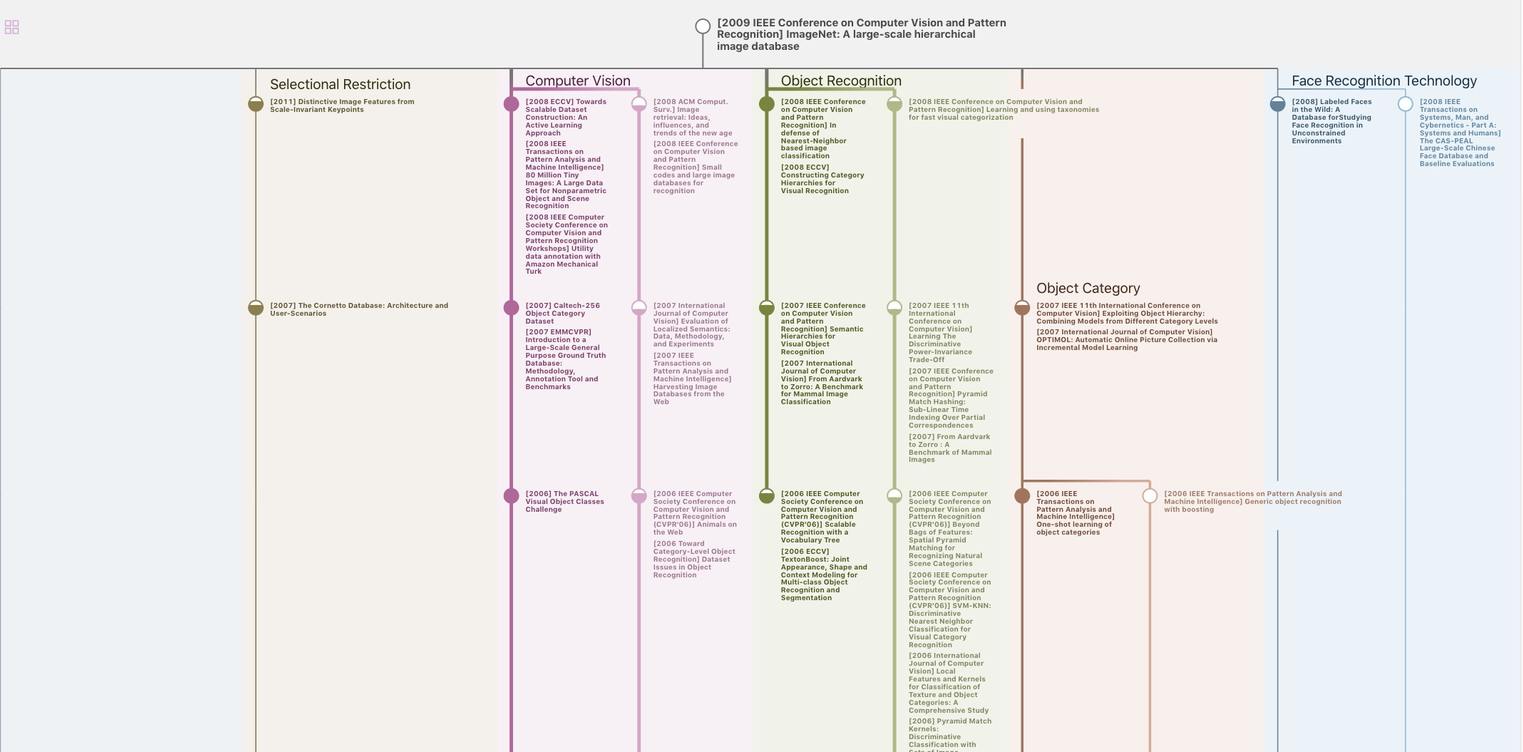
生成溯源树,研究论文发展脉络
Chat Paper
正在生成论文摘要