Subjectivity in Unsupervised Machine Learning Model Selection
CoRR(2023)
摘要
Model selection is a necessary step in unsupervised machine learning. Despite numerous criteria and metrics, model selection remains subjective. A high degree of subjectivity may lead to questions about repeatability and reproducibility of various machine learning studies and doubts about the robustness of models deployed in the real world. Yet, the impact of modelers' preferences on model selection outcomes remains largely unexplored. This study uses the Hidden Markov Model as an example to investigate the subjectivity involved in model selection. We asked 33 participants and three Large Language Models (LLMs) to make model selections in three scenarios. Results revealed variability and inconsistencies in both the participants' and the LLMs' choices, especially when different criteria and metrics disagree. Sources of subjectivity include varying opinions on the importance of different criteria and metrics, differing views on how parsimonious a model should be, and how the size of a dataset should influence model selection. The results underscore the importance of developing a more standardized way to document subjective choices made in model selection processes.
更多查看译文
关键词
subjectivity,machine learning,selection
AI 理解论文
溯源树
样例
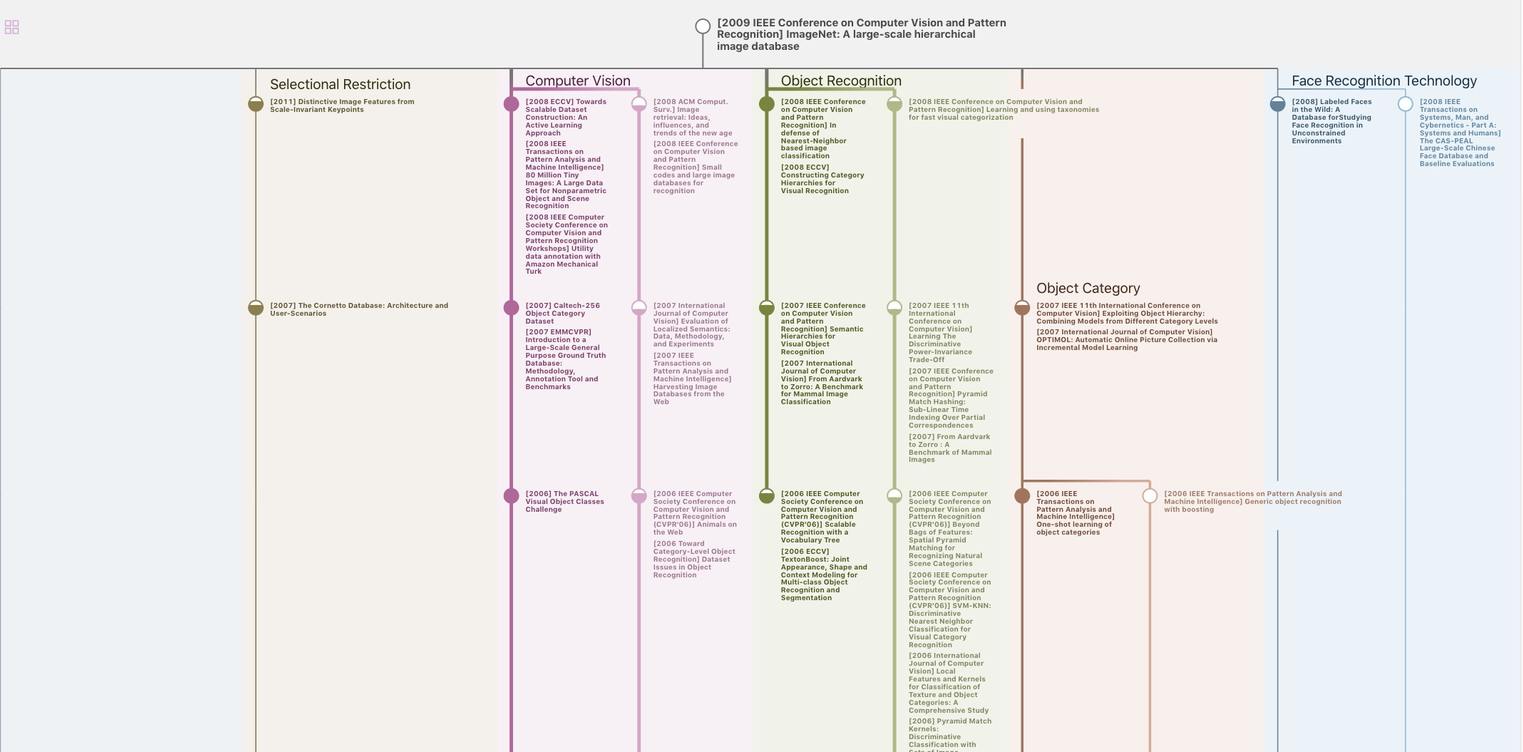
生成溯源树,研究论文发展脉络
Chat Paper
正在生成论文摘要