Edge-Assisted Lightweight Region-of-Interest Extraction and Transmission for Vehicle Perception
GLOBECOM 2023 - 2023 IEEE Global Communications Conference(2023)
摘要
To enhance on-road environmental perception for autonomous driving, accurate and real-time analytics on high-resolution video frames generated from on-board cameras be-comes crucial. In this paper, we design a lightweight object location method based on class activation mapping (CAM) to rapidly capture the region of interest (RoI) boxes that contain driving safety related objects from on-board cameras, which can not only improve the inference accuracy of vision tasks, but also reduce the amount of transmitted data. Considering the limited on-board computation resources, the RoI boxes extracted from the raw image are offloaded to the edge for further processing. Considering both the dynamics of vehicle-to-edge communications and the limited edge resources, we propose an adaptive RoI box offloading algorithm to ensure prompt and accurate inference by adjusting the down-sampling rate of each box. Extensive experimental results on four high-resolution video streams demonstrate that our approach can effectively improve the overall accuracy by up to 16% and reduce the transmission demand by up to 49%, compared with other benchmarks.
更多查看译文
关键词
Computational Resources,Raw Images,Video Frames,Object Location,Vision Tasks,Task Accuracy,Inference Accuracy,Limited Computational Resources,Class Activation Maps,High-resolution Video,Onboard Camera,Convolutional Neural Network,Feature Maps,Object Detection,Long Short-term Memory,Resource Consumption,Mean Accuracy,Convolutional Neural Network Model,Redundant Information,Region Of Interest Extraction,Edge Server,Raw Frames,Lightweight Convolutional Neural Network,Target Object,Frames Per Second,Region Proposal,Autonomous Vehicles,Video Analysis,Inference Results
AI 理解论文
溯源树
样例
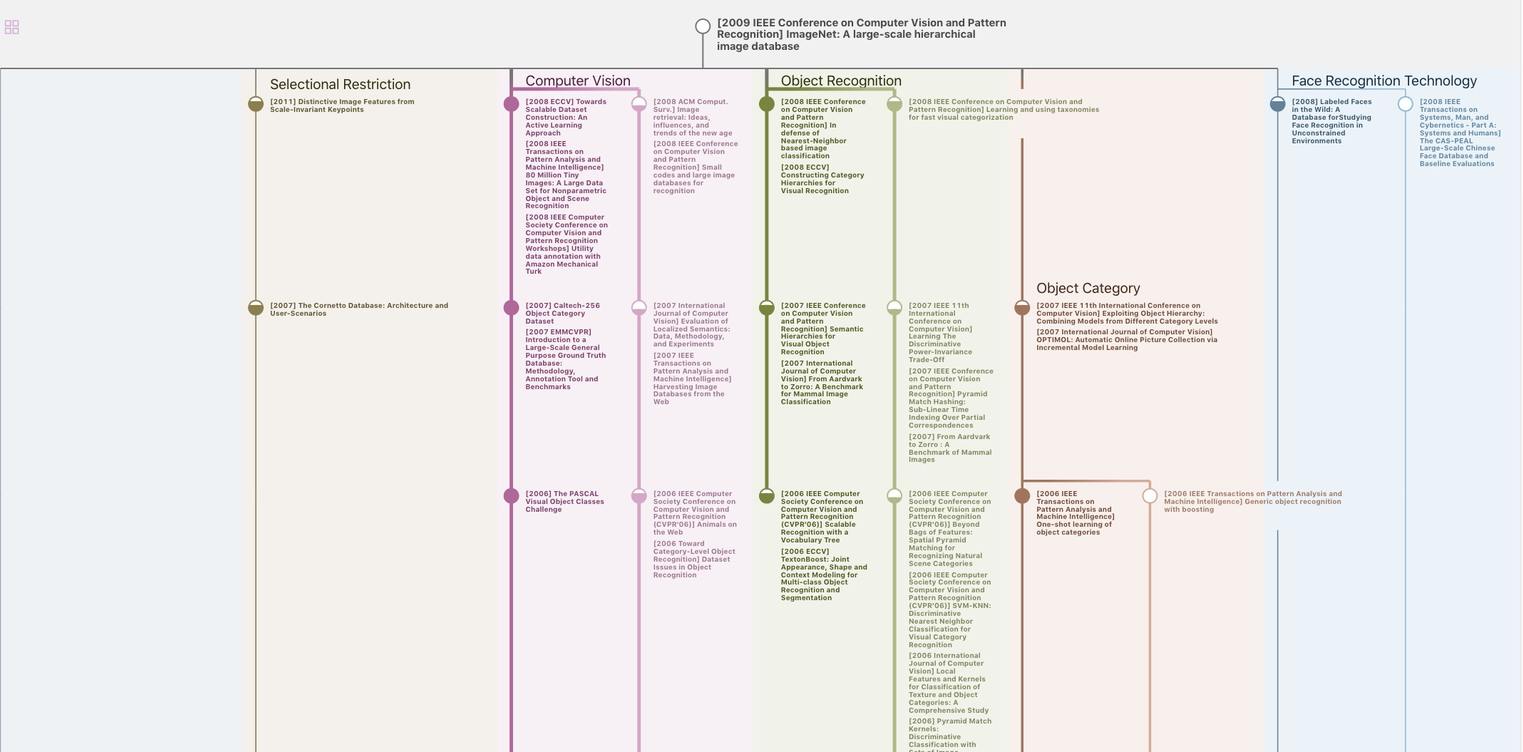
生成溯源树,研究论文发展脉络
Chat Paper
正在生成论文摘要