Graph fusion prediction of autism based on attentional mechanisms.
Journal of biomedical informatics(2023)
摘要
Autism spectrum disorder (ASD) is a pervasive developmental disorder, and the earlier detection and timely intervention for treatment positively affect the prognosis of patients. Deep learning algorithms based on graph structure have achieved good results in autism prediction in recent years. However, there are problems with standardized operations in extracting features and combining neighborhood node features with the structure of the graph dependent, which limits the generalization ability of the trained model to other graph structures. In this paper, we propose a graph fusion autism prediction model based on attentional mechanisms(AGF) to address the above problems. The AGF model represents the overall population (patients or healthy controls) as a sparse graph, where nodes are subjects, and non-imaging features are integrated as edge weights. Different weights can be defined for different nodes in the neighborhood through the attention mechanism without relying on prior knowledge of the graph structure. The model is also able to dynamically fuse multiple sparse graphs obtained from different non-imaging features by way of training weight assignment. Its performance is also compared with several other models (e.g., S-AGF, GCN, etc.), and the results show that it has superior prediction accuracy compared to the baseline model. The results show that this improvement of graph fusion works better on the ABIDE databases, and the classification accuracy can reach 73.9%. The datasets and source code are freely available at https://github.com/chengyu-github1012/Graph-Fusion.git. Strengths and limitations of this study: graph fusion; disease prediction; noise.
更多查看译文
AI 理解论文
溯源树
样例
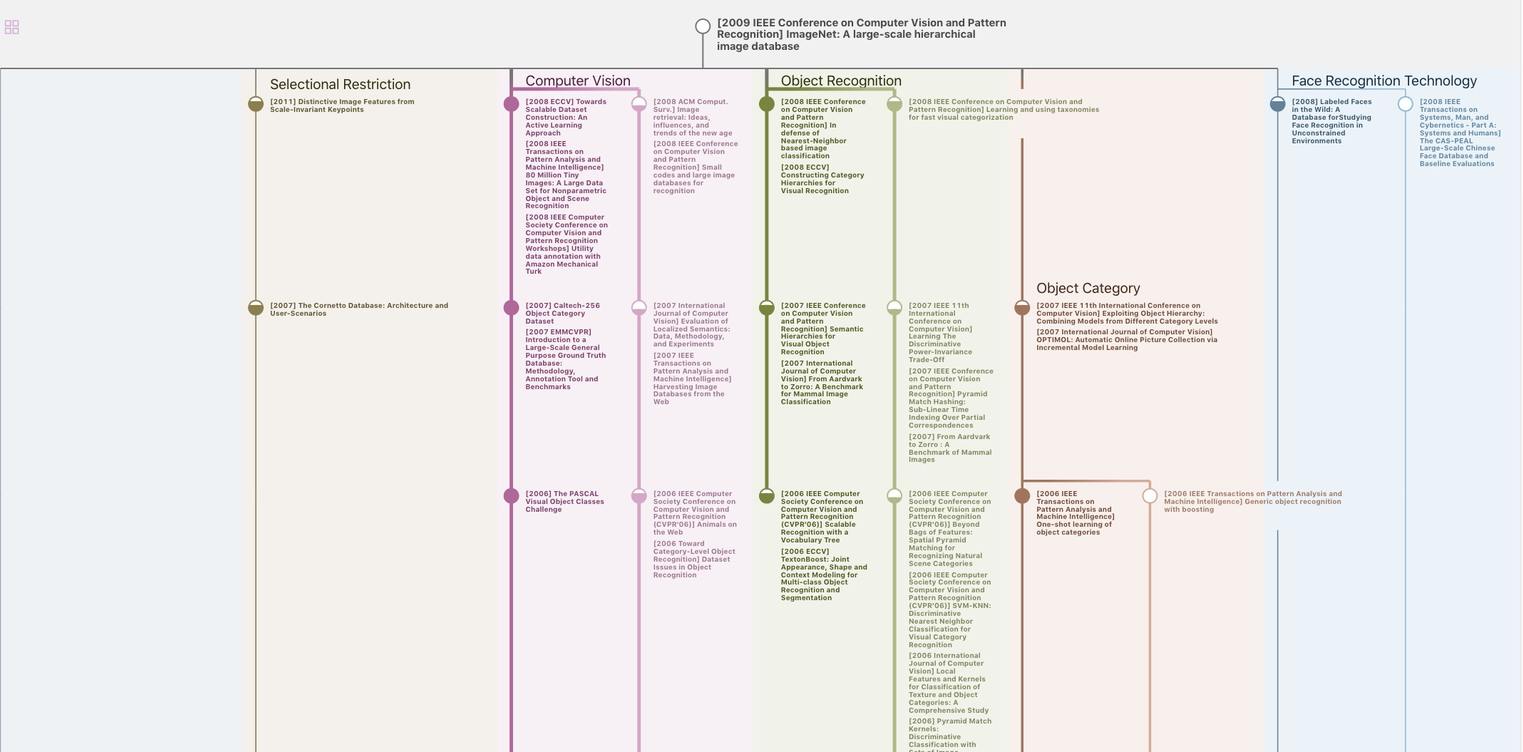
生成溯源树,研究论文发展脉络
Chat Paper
正在生成论文摘要