#InsTag: Instruction Tagging for Analyzing Supervised Fine-tuning of Large Language Models.
CoRR(2023)
摘要
Foundation language models obtain the instruction-following ability through supervised fine-tuning (SFT). Diversity and complexity are considered critical factors of a successful SFT dataset, while their definitions remain obscure and lack quantitative analyses. In this work, we propose InsTag, an open-set fine-grained tagger, to tag samples within SFT datasets based on semantics and intentions and define instruction diversity and complexity regarding tags. We obtain 6.6K tags to describe comprehensive user queries. Then we analyze popular open-sourced SFT datasets and find that the model ability grows with more diverse and complex data. Based on this observation, we propose a data selector based on InsTag to select 6K diverse and complex samples from open-source datasets and fine-tune models on InsTag-selected data. The resulting models, TagLM, outperform open-source models based on considerably larger SFT data evaluated by MT-Bench, echoing the importance of query diversity and complexity. We open-source InsTag in https://github.com/OFA-Sys/InsTag.
更多查看译文
关键词
Data Analysis,Supervised Fine-tuning for Human Alignment,Large Language Model
AI 理解论文
溯源树
样例
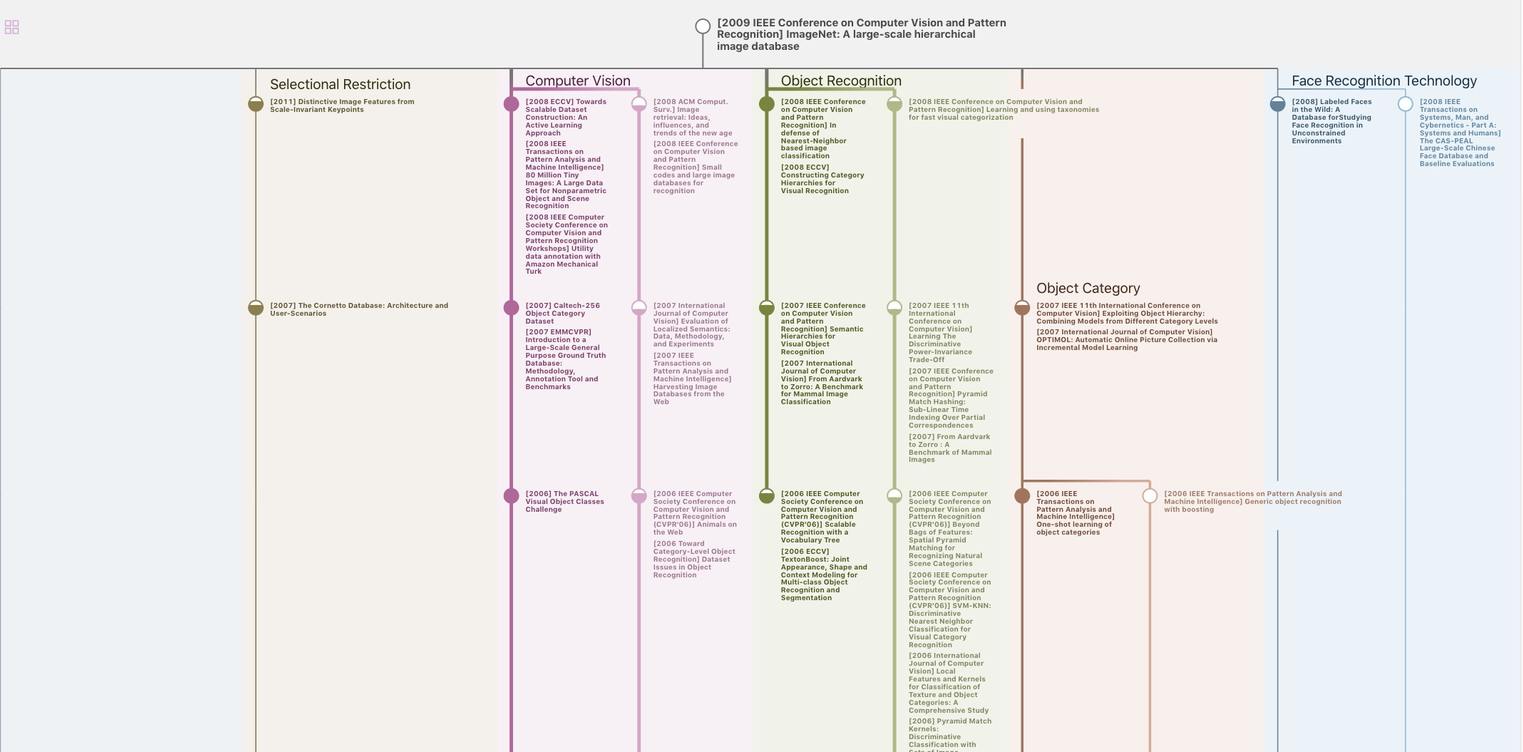
生成溯源树,研究论文发展脉络
Chat Paper
正在生成论文摘要