A Learnable Group-Tube Transform Induced Tensor Nuclear Norm and Its Application for Tensor Completion
SIAM JOURNAL ON IMAGING SCIENCES(2023)
摘要
The transform-based tensor nuclear norm (TNN) methods have shown good recovery results for tensor completion. However, the TNN methods are based on the single-tube transforms in which transforms are applied to each tube independently. The performance of the single-tube transform -based TNN methods is not good for recovery of missing tubes in multidimensional images (e.g., all the observations are missing in a pixel location of multispectral images). The main aim of this paper is to address this issue by proposing and developing a learnable group-tube transform-based TNN (GTNN) method that can effectively explore the correlation of neighboring tubes by leveraging a learnable group-tube transform. The proposed learnable group-tube transform is a separable three-dimensional transform that consists of a one-dimensional spectral/temporal transform (i.e., single-tube transform) and a two-dimensional spatial transform. Such group-tube transform can effectively explore the correlation of neighboring tubes. Based on the elaborately designed low-rank metric GTNN, we suggest a low-rank tensor completion model. To solve this highly nonconvex model, we design an efficient multiblock proximal alternating minimization algorithm and establish the convergence guarantee. A variety of numerical experiments on real-world multidimensional imaging data including traffic speed data, color images, videos, and multispectral images collectively manifest that the GTNN method outperforms some state-of-the-art TNN methods especially when the observations along tubes are missing.
更多查看译文
关键词
tensor completion,group-tube transform,proximal alternating minimization algorithm,tensor nuclear norm
AI 理解论文
溯源树
样例
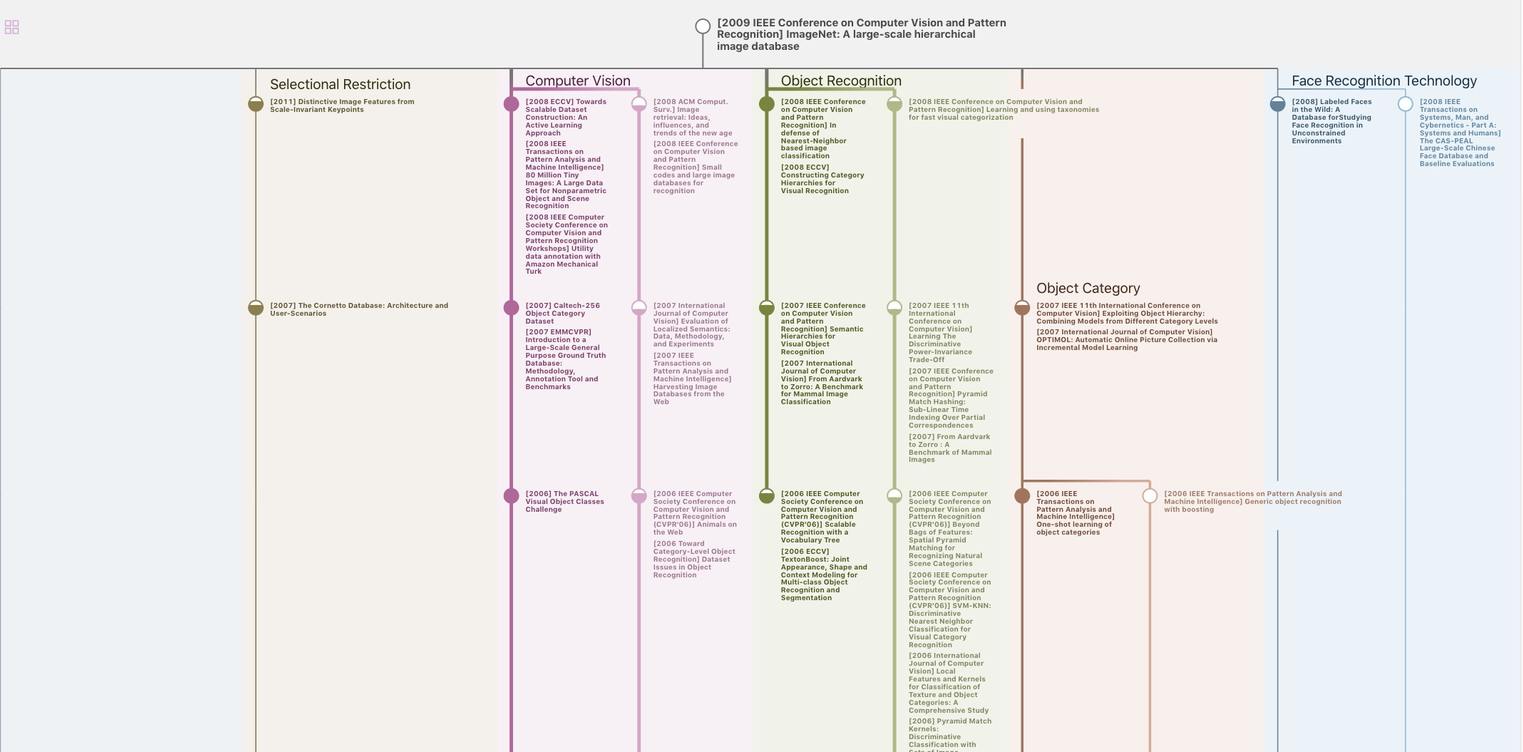
生成溯源树,研究论文发展脉络
Chat Paper
正在生成论文摘要