S3Net: Superpixel-Guided Self-Supervised Learning Network for Multitemporal Image Change Detection
IEEE Geosci. Remote. Sens. Lett.(2023)
摘要
Deep learning (DL) has recently achieved outstanding performance in change detection of multitemporal images. However, most existing DL-based change detection methods still suffer from the problem of insufficient labeled training samples. To overcome this limitation, an unsupervised superpixel-guided self-supervised learning network (S3Net) is proposed for detecting changes occurred on the land surface. By performing principal component analysis on two input images, a triple-channel pseudocolor image containing the main information of both the images is first generated, which is used for superpixel segmentation to produce homogeneous image objects. Then, a Siamese network composing of two identical subnetworks with shared weight based on transfer learning is trained for pretext task in a self-supervised learning way, aiming to obtain multiscale object-level spatial feature difference images. On this basis, a high-quality difference image is generated by incorporating the pixel-level and object-level difference information using a simple weighted fusion strategy, which can be analyzed by thresholding to produce the final binary change map. The experimental results on four real-world datasets from different sensors show that the proposed approach can obtain superior performance in comparison to several state-of-the-art change detection methods, which further demonstrates its effectiveness and practicability. We make our data and code publicly available (https://github.com/OMEGA-RS/S3Net_CD).
更多查看译文
关键词
Change detection,multitemporal images,self-supervised learning,superpixel segmentation,transfer learning
AI 理解论文
溯源树
样例
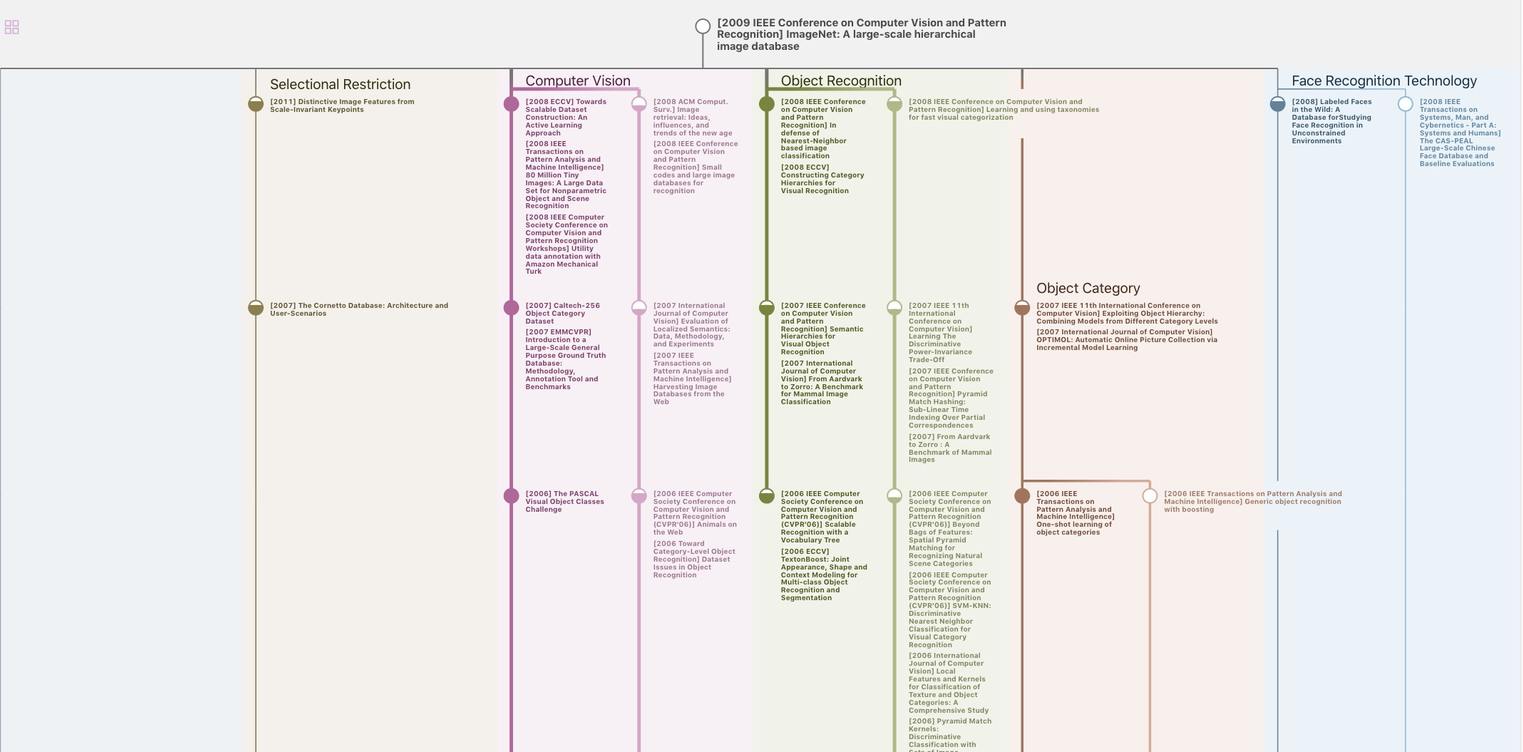
生成溯源树,研究论文发展脉络
Chat Paper
正在生成论文摘要