Low-resource Multilingual Neural Translation Using Linguistic Feature-based Relevance Mechanisms.
ACM Trans. Asian Low Resour. Lang. Inf. Process.(2023)
摘要
This article investigates approaches to effectively harness source-side linguistic features for low-resource multilingual neural machine translation (MNMT). Previous works focus on using various features of a word such as lemma, part-of-speech tag, dependency label, and so on, to improve translation quality in a low-resource scenario. However, these studies deal with bilingual translation and do not focus on using features in multilingual training setups. Our work focuses on this particular point and experiments with low-resource multilingual models incorporating source-side linguistic features. Although techniques for integrating features into an NMT model such as concatenation and feature relevance perform quite well in bilingual settings, they do not work well in multilingual settings. To remedy this, we propose the use of dummy features and language indicator features in MNMT models. Experiments are conducted on English to Asian language translation on a multilingual, multi-parallel corpus spanning English and eight Asian languages where for each language pair, the training data size does not exceed 20,000 parallel sentences. After establishing strong bilingual baselines using feature relevance mechanisms and multilingual baselines without any features, we show that our proposed dummy features and language indicator features, in combination with feature relevance mechanisms, yield significant improvements in BLEU points for all language pairs. We then analyze our models from the perspectives of model sizes, the impact of individual linguistic features, validation perplexity computed during training, visualization of the activations of the relevance mechanisms, and exhaustive tuning of hyperparameters. We also report preliminary results for multilingual multi-way models using linguistic features.
更多查看译文
关键词
Linguistic features,morphology,neural networks
AI 理解论文
溯源树
样例
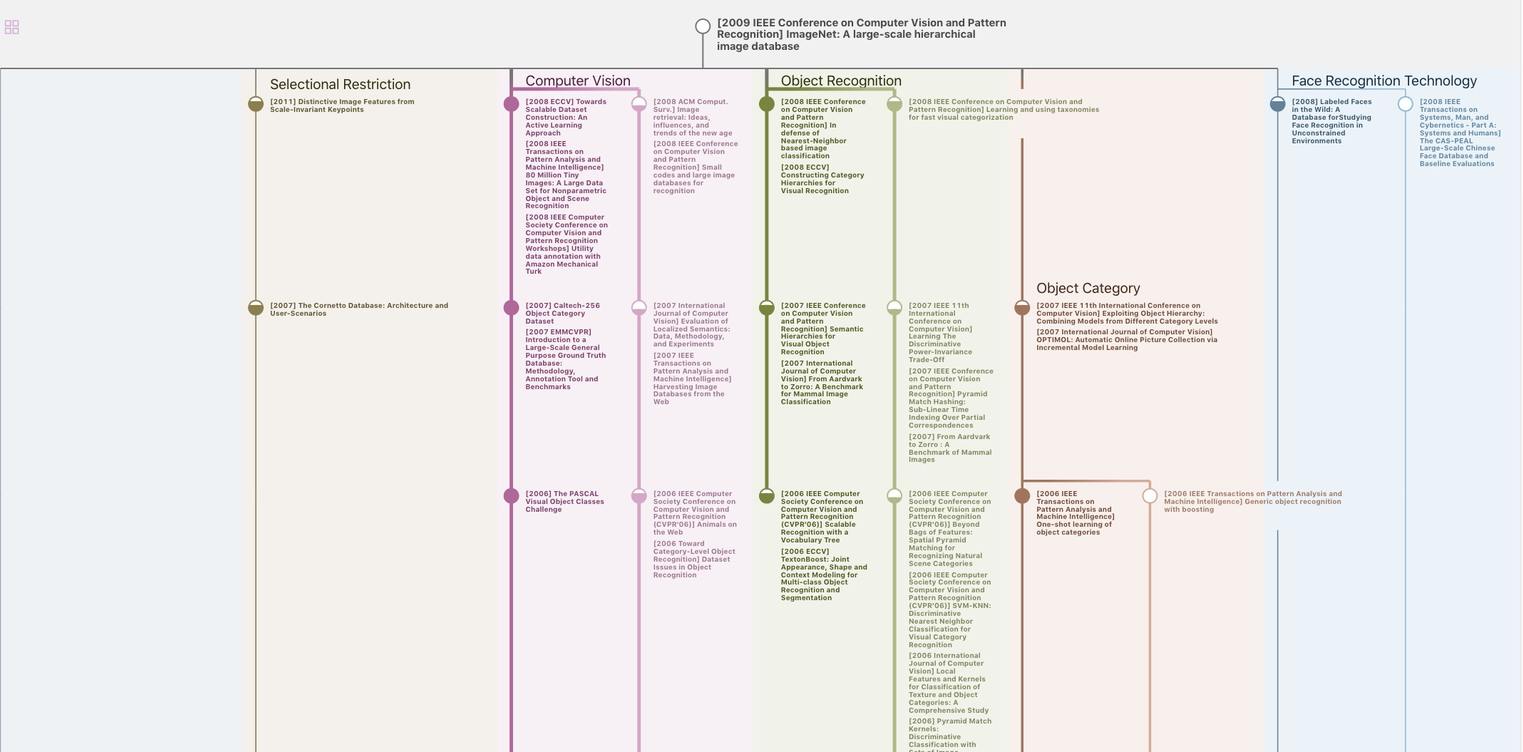
生成溯源树,研究论文发展脉络
Chat Paper
正在生成论文摘要