VirFace: A Semi-Supervised Method for Enhancing Face Recognition via Unlabeled Shallow Data.
IEEE Trans. Inf. Forensics Secur.(2023)
摘要
The semi-supervised face recognition problem has become a popular research topic in recent years. However, one common and important situation, in which the unlabeled data is shallow, has rarely been considered in most existing works. In this paper, shallow data means there are only few images per identity. In the unlabeled shallow situation, the existing semi-supervised face recognition methods generally do not work well. Thus, how to effectively utilize the unlabeled shallow face data for improving face recognition performance is an important issue. In this paper, we propose a novel semi-supervised face recognition method, namely VirFace
$^{\infty} $
, to enhance the face recognition performance effectively with the unlabeled shallow data. VirFace
$^{\infty} $
consists of VirClass and VirDistribution components. In VirClass, we inject the unlabeled data as virtual classes into the feature space to enlarge the inter-class distance. In VirDistribution, we predict the distribution of each virtual class, namely virtual distribution, and then enhance the inter-class discriminativeness by enlarging the distances between the labeled features and the virtual distributions. To the best of our knowledge, we are among the first to tackle the face recognition problem on unlabeled shallow face data. Extensive experiments demonstrate the superiority of our proposed method.
更多查看译文
关键词
Face recognition, unlabeled shallow data, semi supervised learning, VirFace(8), VirClass, VirDistribution
AI 理解论文
溯源树
样例
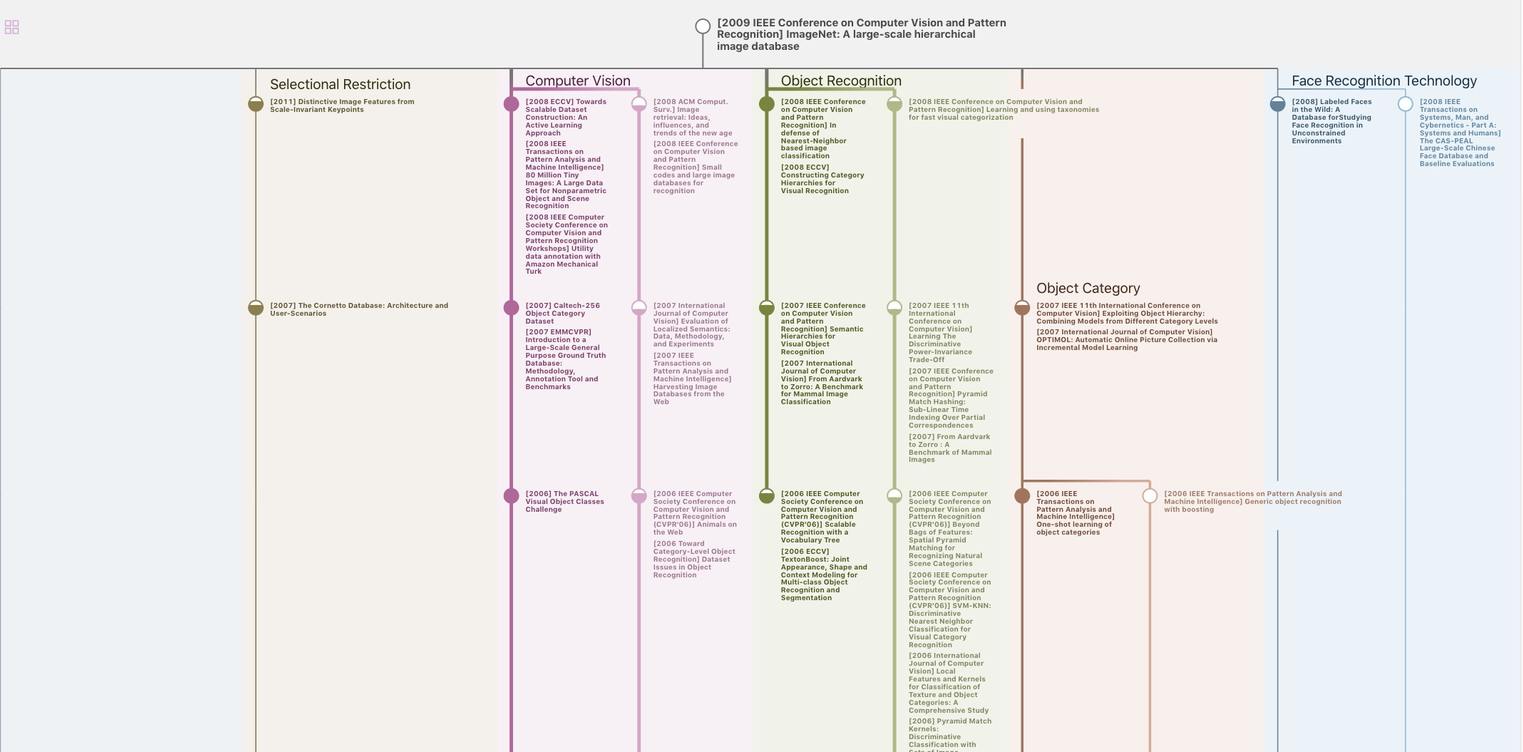
生成溯源树,研究论文发展脉络
Chat Paper
正在生成论文摘要