Deep-Reinforcement-Learning-Assisted Client Selection in Nonorthogonal-Multiple-Access-Based Federated Learning.
IEEE Internet Things J.(2023)
摘要
To reap the benefit of big data generated by the massive number of Internet of Things (IoT) devices while preserving data privacy, federated learning (FL) has been proposed to enable IoT devices to train machine learning models locally. That is, instead of sharing the local data sets, different clients in terms of IoT devices only need to upload their local models to a centralized FL server. Client selection in FL is critical to maximize the number of qualified clients, who can successfully upload their local models to the FL server before the predefined deadline. Normally, client selection is coupled with wireless resource management owing to the fact that different clients need to share the same spectrum to upload their local models. The existing solutions of joint optimizing client selection and resource management are designed based on frequency-division multiple access (FDMA) or time-division multiple access (TDMA), which do not consider the dynamics of the clients and lead to low bandwidth utilization. In this article, we propose the Nonorthogonal-Multiple-Access (NOMA)-based resource allocation for client selection in FL to dynamically and jointly optimize client selection for each global iteration as well as the transmission power of each selected client in each time slot within a global iteration. We design the deep-reinforcement-learn-based client selection in NOMA-based federated learning (DREAM-FL) algorithm to solve the problem. Extensive simulations are conducted to demonstrate that DREAM-FL can select more qualified clients and has higher model accuracy than FDMA and TDMA-based solutions.
更多查看译文
关键词
Client selection,deep-reinforcement-learn,federated learning (FL),Nonorthogonal-Multiple-Access (NOMA)
AI 理解论文
溯源树
样例
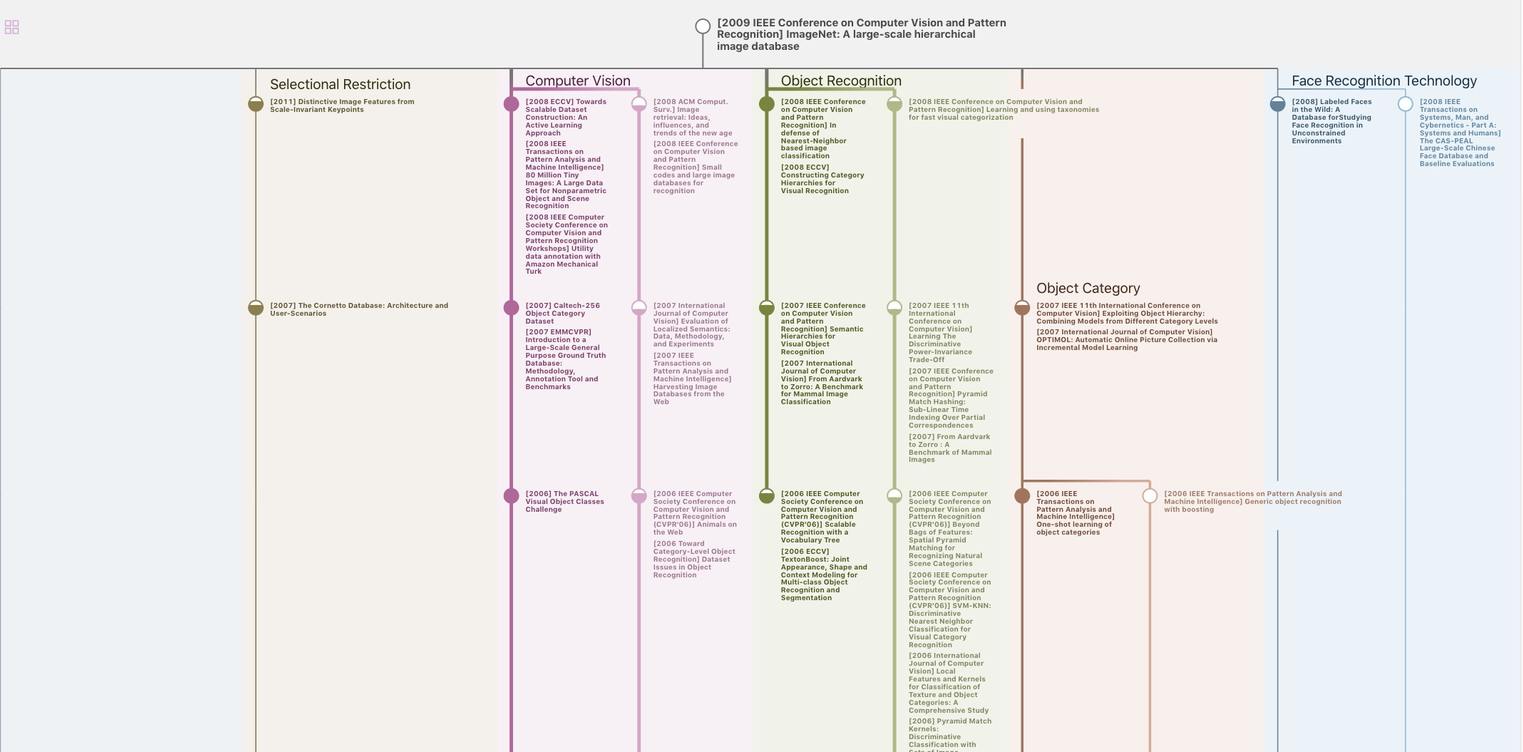
生成溯源树,研究论文发展脉络
Chat Paper
正在生成论文摘要