A Robust Spectral Clustering Algorithm for Sub-Gaussian Mixture Models with Outliers.
Oper. Res.(2023)
摘要
Traditional clustering algorithms such as k-means and vanilla spectral clustering are known to deteriorate significantly in the presence of outliers. Several previous works in literature have proposed robust variants of these algorithms; however, they do not provide any theoretical guarantees. Extending previous clustering literature on Gaussian mixture models, in their paper “A Robust Spectral Clustering Algorithm for Sub-Gaussian Mixture Models with Outliers,” Prateek R. Srivastava, Purnamrita Sarkar, and Grani A. Hanasusanto developed a new spectral clustering algorithm and provided error bounds for the algorithm under a general sub-Gaussian mixture model setting with outliers. Surprisingly, their derived error bound matches with the best-known bound for semidefinite programs under the same setting without outliers. Numerical experiments on a variety of simulated and real-world data sets further demonstrate that their algorithm is less sensitive to outliers compared with other state-of-the-art algorithms. We consider the problem of clustering data sets in the presence of arbitrary outliers. Traditional clustering algorithms such as k -means and spectral clustering are known to perform poorly for data sets contaminated with even a small number of outliers. In this paper, we develop a provably robust spectral clustering algorithm that applies a simple rounding scheme to denoise a Gaussian kernel matrix built from the data points and uses vanilla spectral clustering to recover the cluster labels of data points. We analyze the performance of our algorithm under the assumption that the “good” data points are generated from a mixture of sub-Gaussians (we term these “inliers”), whereas the outlier points can come from any arbitrary probability distribution. For this general class of models, we show that the misclassification error decays at an exponential rate in the signal-to-noise ratio, provided the number of outliers is a small fraction of the inlier points. Surprisingly, this derived error bound matches with the best-known bound for semidefinite programs (SDPs) under the same setting without outliers. We conduct extensive experiments on a variety of simulated and real-world data sets to demonstrate that our algorithm is less sensitive to outliers compared with other state-of-the-art algorithms proposed in the literature. Funding: G. A. Hanasusanto was supported by the National Science Foundation Grants NSF ECCS-1752125 and NSF CCF-2153606. P. Sarkar gratefully acknowledges support from the National Science Foundation Grants NSF DMS-1713082, NSF HDR-1934932 and NSF 2019844. Supplemental Material: The online appendix is available at https://doi.org/10.1287/opre.2022.2317 .
更多查看译文
关键词
Machine Learning and Data Science,spectral clustering,sub-Gaussian mixture models,kernel methods,semidefinite programming,outlier detection,asymptotic analysis
AI 理解论文
溯源树
样例
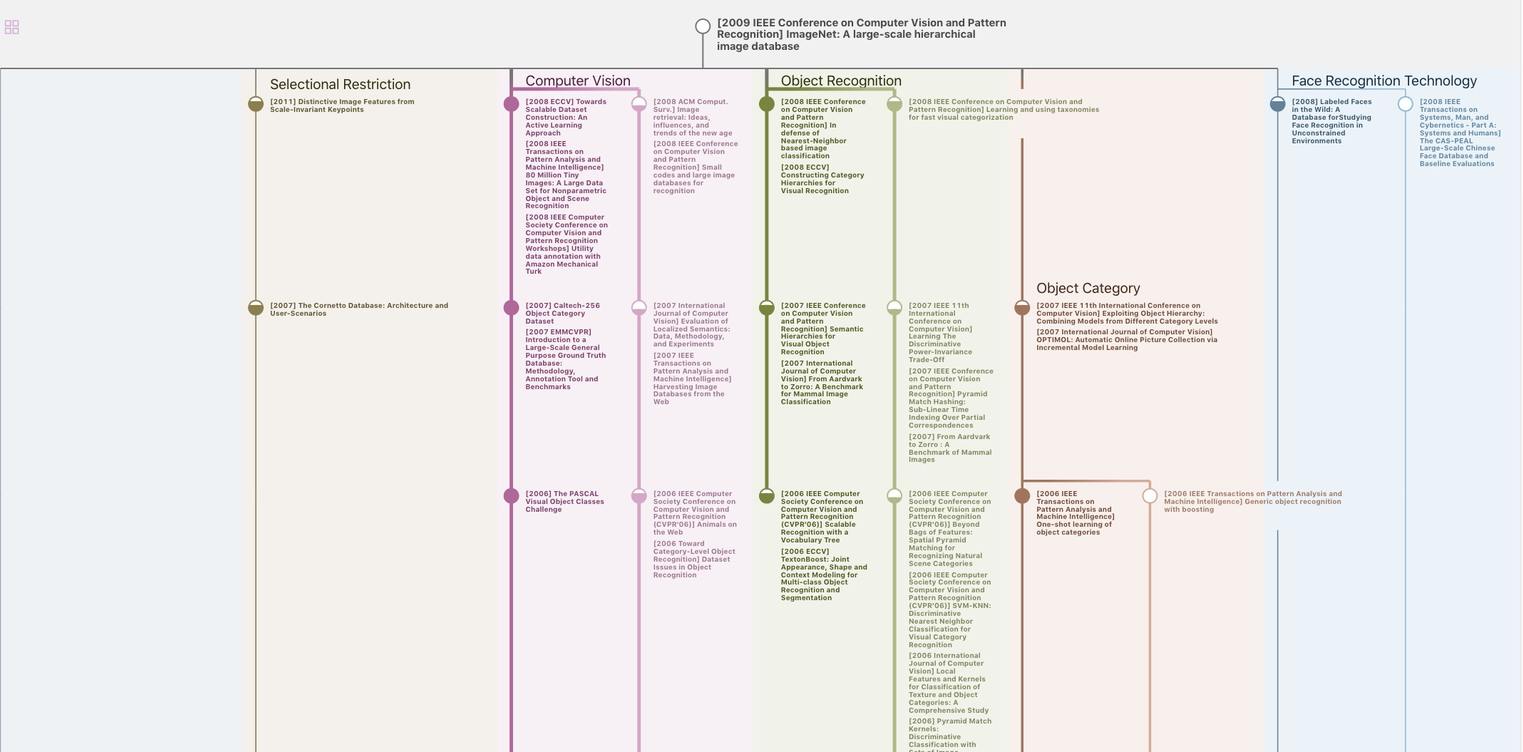
生成溯源树,研究论文发展脉络
Chat Paper
正在生成论文摘要