Optimistic Gittins Indices.
Oper. Res.(2022)
摘要
We propose a tightening sequence of optimistic approximations to the Gittins index in “Optimistic Gittins Indices.” We show that the use of these approximations in concert with the use of an increasing discount factor appears to offer a compelling alternative to state-of-the-art index schemes proposed for the Bayesian multiarmed bandit problem. We prove that the use of these optimistic indices constitutes a regret optimal algorithm. Perhaps more interestingly, the use of even the loosest of these approximations appears to offer substantial performance improvements over state-of-the-art alternatives while incurring little to no additional computational overhead relative to the simplest of these alternatives. Recent years have seen a resurgence of interest in Bayesian algorithms for the multiarmed bandit (MAB) problem, such as Thompson sampling. These algorithms seek to exploit prior information on arm biases. The empirically observed performance of these algorithms makes them a compelling alternative to their frequentist counterparts. Nonetheless, there appears to be a wide range in empirical performance among such Bayesian algorithms. These algorithms also vary substantially in their design (as opposed to being variations on a theme). In contrast, if one cared about Bayesian regret discounted over an infinite horizon at a fixed, prespecified rate, the celebrated Gittins index theorem offers an optimal algorithm. Unfortunately, the Gittins analysis does not appear to carry over to minimizing Bayesian regret over all sufficiently large horizons and computing a Gittins index is onerous relative to essentially any incumbent index scheme for the Bayesian MAB problem. The present paper proposes a tightening sequence of optimistic approximations to the Gittins index. We show that the use of these approximations in concert with the use of an increasing discount factor appears to offer a compelling alternative to state-of-the-art index schemes proposed for the Bayesian MAB problem in recent years. We prove that these optimistic indices constitute a regret optimal algorithm, in the sense of meeting the Lai-Robbins lower bound, including matching constants. Perhaps more interestingly, the use of even the loosest of these approximations appears to offer substantial performance improvements over state-of-the-art alternatives (including Thompson sampling, information direct sampling, and the Bayes UCB algorithm) while incurring little to no additional computational overhead relative to the simplest of these alternatives. Funding: Both authors were partially supported by NSG Grant CMMI 1727239.
更多查看译文
关键词
Decision Analysis,multiarmed bandits,Gittins index,online learning
AI 理解论文
溯源树
样例
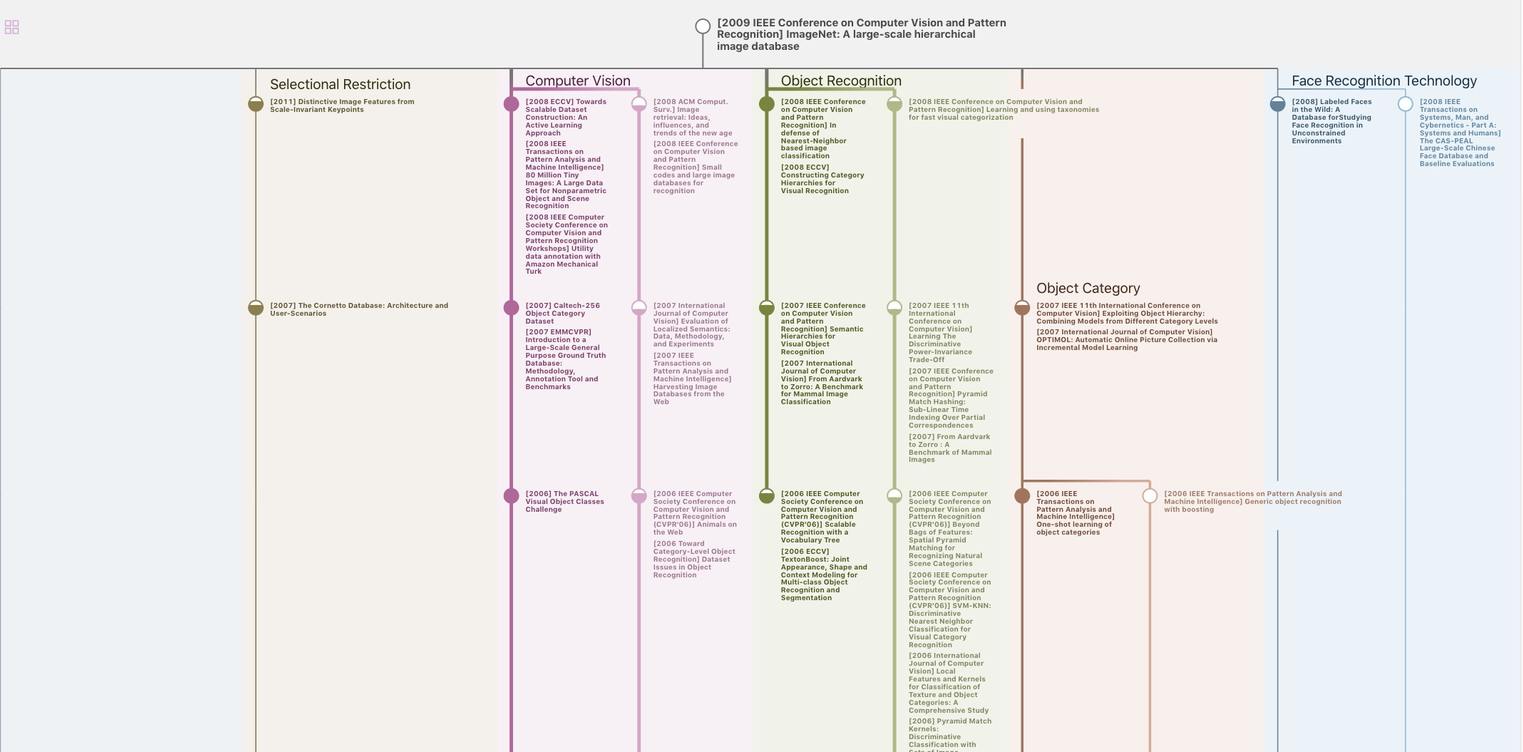
生成溯源树,研究论文发展脉络
Chat Paper
正在生成论文摘要