Contrastive learning with semantic consistency constraint.
Image Vis. Comput.(2023)
摘要
Contrastive representation learning (CL) can be viewed as an anchor-based learning paradigm that learns rep-resentations by maximizing the similarity between an anchor and positive samples while reducing the similarity with negative samples. A randomly adopted data augmentation strategy generates positive and negative samples, resulting in semantic inconsistency in the learning process. The randomness may introduce additional distur-bances to the original sample, thereby reversing the sample identity. Also, the negative sample demarcation strategy makes the negative samples containing semantically similar samples to the anchors, called false negative samples. Therefore, CL's maximization and reduction process cause distractors to be incorporated into the learned feature representation. In this paper, we propose a novel Semantic Consistency Regularization (SCR) method to alleviate this problem. Specifically, we introduce a new regularization item, pairwise subspace dis-tance, to constrain the consistency of distributions across different views. Furthermore, we propose a divide-and-conquer strategy to ensure that the proposed SCR is well-suited for large mini-batch cases. Empirically, results across multiple benchmark mini and large datasets demonstrate that SCR outperforms state-of-the-art methods. Codes are available at https://github.com/PaulGHJ/SCR.git.
更多查看译文
关键词
Representation learning, Contrastive learning, Semantic consistency
AI 理解论文
溯源树
样例
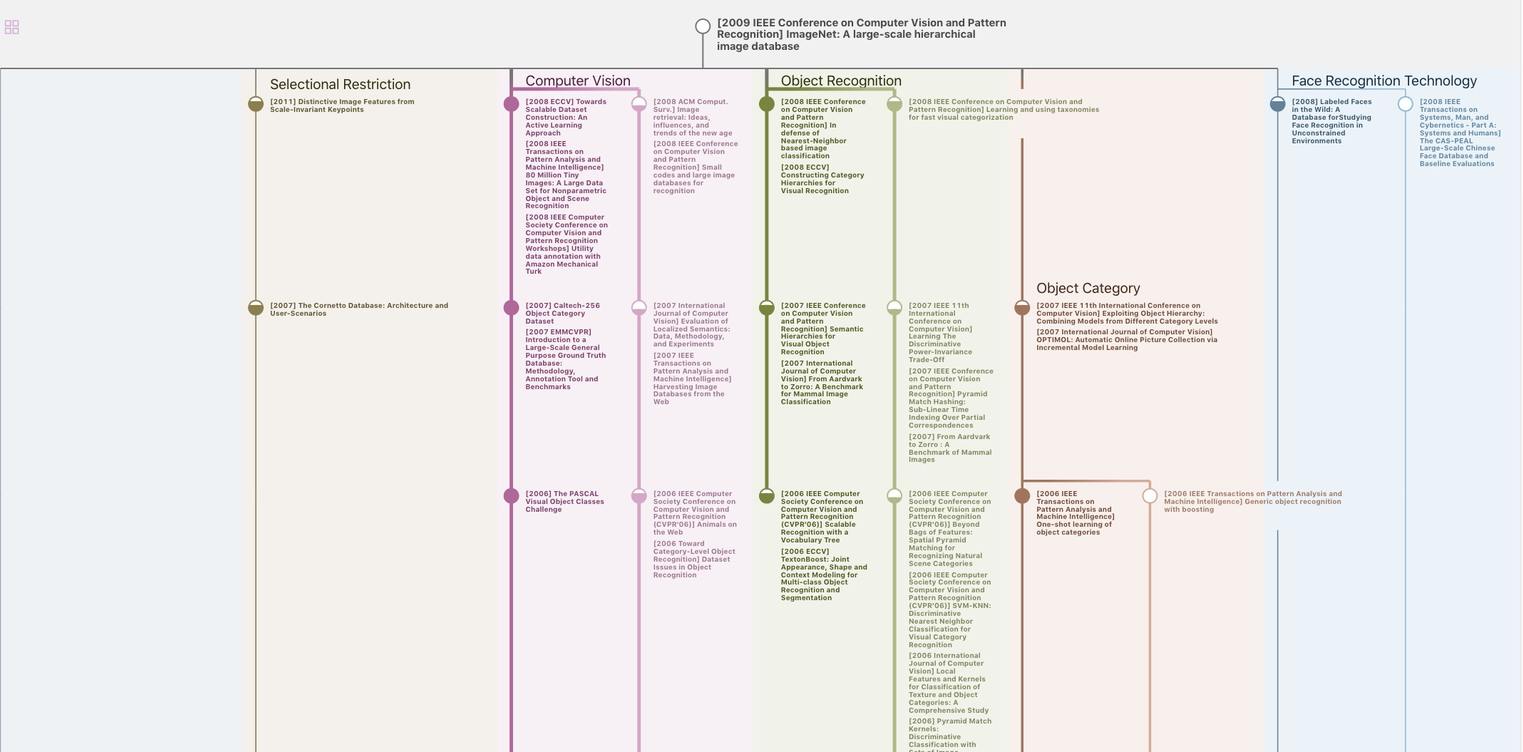
生成溯源树,研究论文发展脉络
Chat Paper
正在生成论文摘要