Optimizing Numerical Weather Prediction Model Performance Using Machine Learning Techniques
IEEE Access(2023)
摘要
Weather forecasting primarily uses numerical weather prediction models that use weather observation data, including temperature and humidity, to predict future weather. The Korea Meteorological Administration (KMA) has adopted the GloSea6 numerical weather prediction model from the UK for weather forecasting. Besides utilizing these models for real-time weather forecasts, supercomputers are essential for running them for research purposes. However, owing to the limited supercomputer resources, many researchers have faced difficulties running the models. To address this issue, the KMA has developed a low-resolution model called Low GloSea6, which can be run on small and medium-sized servers in research institutions, but Low GloSea6 still uses numerous computer resources, especially in the I/O load. As I/O load can cause performance degradation for models with high data I/O, model I/O optimization is essential, but trial-and-error optimization by users is inefficient. Therefore, this study presents a machine learning-based approach to optimize the hardware and software parameters of the Low GloSea6 research environment. The proposed method comprised two steps. First, performance data were collected using profiling tools to obtain hardware platform parameters and Low GloSea6 internal parameters under various settings. Second, a machine learning model was trained using the collected data to determine the optimal hardware platform parameters and Low GloSea6 internal parameters for new research environments. The machine-learning model successfully predicted the optimal parameter combinations in different research environments, exhibiting a high degree of accuracy compared to the actual parameter combinations. In particular, the predicted model execution time based on the parameter combination showed a significant outcome with an error rate of only 16% compared to the actual execution time. Overall, this optimization method holds the potential to improve the performance of other high-performance computing scientific applications.
更多查看译文
关键词
Scientific application,GloSea6,machine learning,I/O optimization,profiling
AI 理解论文
溯源树
样例
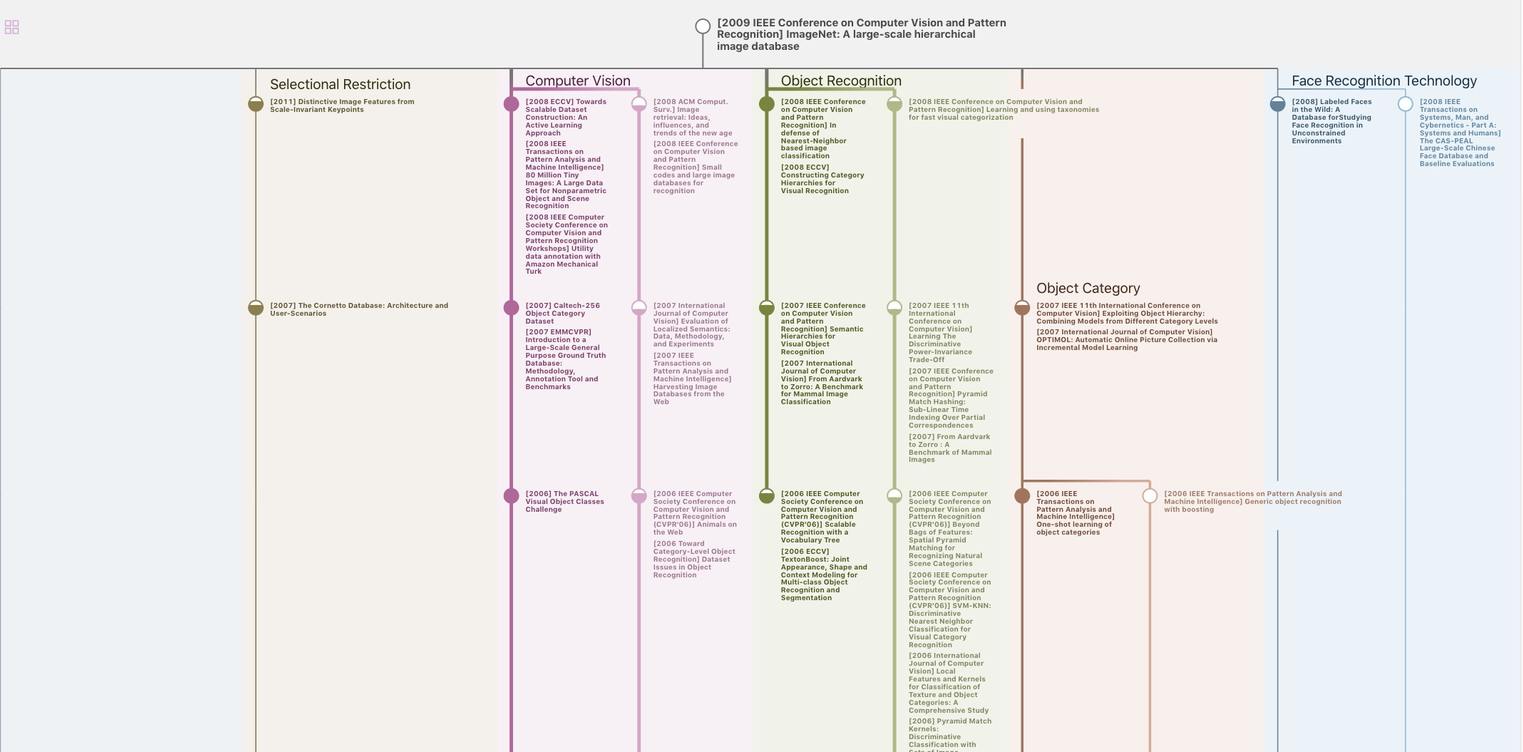
生成溯源树,研究论文发展脉络
Chat Paper
正在生成论文摘要