Predicting the Future Popularity of Academic Publications Using Deep Learning by Considering It as Temporal Citation Networks
IEEE Access(2023)
摘要
One of the key goals of Informetrics is to identify citation-based popular articles among so many other aspects, such as determining popular research topics, identifying influential scholars, and predicting hot trends in science. These can be achieved by applying network science approaches to scientific networks and formulating the problem as a popular (most-cited) node ranking task. To rank the papers based on their future citation gain. In this work a deep learning based framework is proposed. Which helps in automatic node level feature extraction and can make node level prediction in dynamic graphs such as citation networks. To achieve this we have learned global ranking preserve d dimensional node embedding. We have only considered temporal features, which makes it suitable for generalisation to other networks. Although our model can consider node level explicit features also. Further we have given novel cost function which can be easily solve ranking problem for dynamic graphs using probabilistic regression method. Which can be easily optimised. Another novelty of our work is that our model can be trained using different snapshots of the graph and different time. Further trained model can be used to make future prediction. The proposed model has been tested on an arXiv paper citation network using six standard information retrieval-based metrics. The results show that our proposed model outperforms, on average, other state-of-the-art static models as well as dynamic node ranking models. The outcome of this research study leads to informed data-driven decision-making in science, such as the allocation and distribution of research funds and investment in strategic research centers. When considering past time window size as 10 months and making prediction after 10 months our proposed model's performance on various ranking based evaluation metrics are as follows: AUC-0.974, Kendal's rank correlation tau-0.455, Precision- 0.643, Novelty-0.0456, Temporal novelty-0.375 and on NDCG-0.949. Our model is able to make long term trend prediction with just training on short time window.
更多查看译文
关键词
Citation prediction,citation networks,node ranking,deep learning,temporal networks,and popularity prediction
AI 理解论文
溯源树
样例
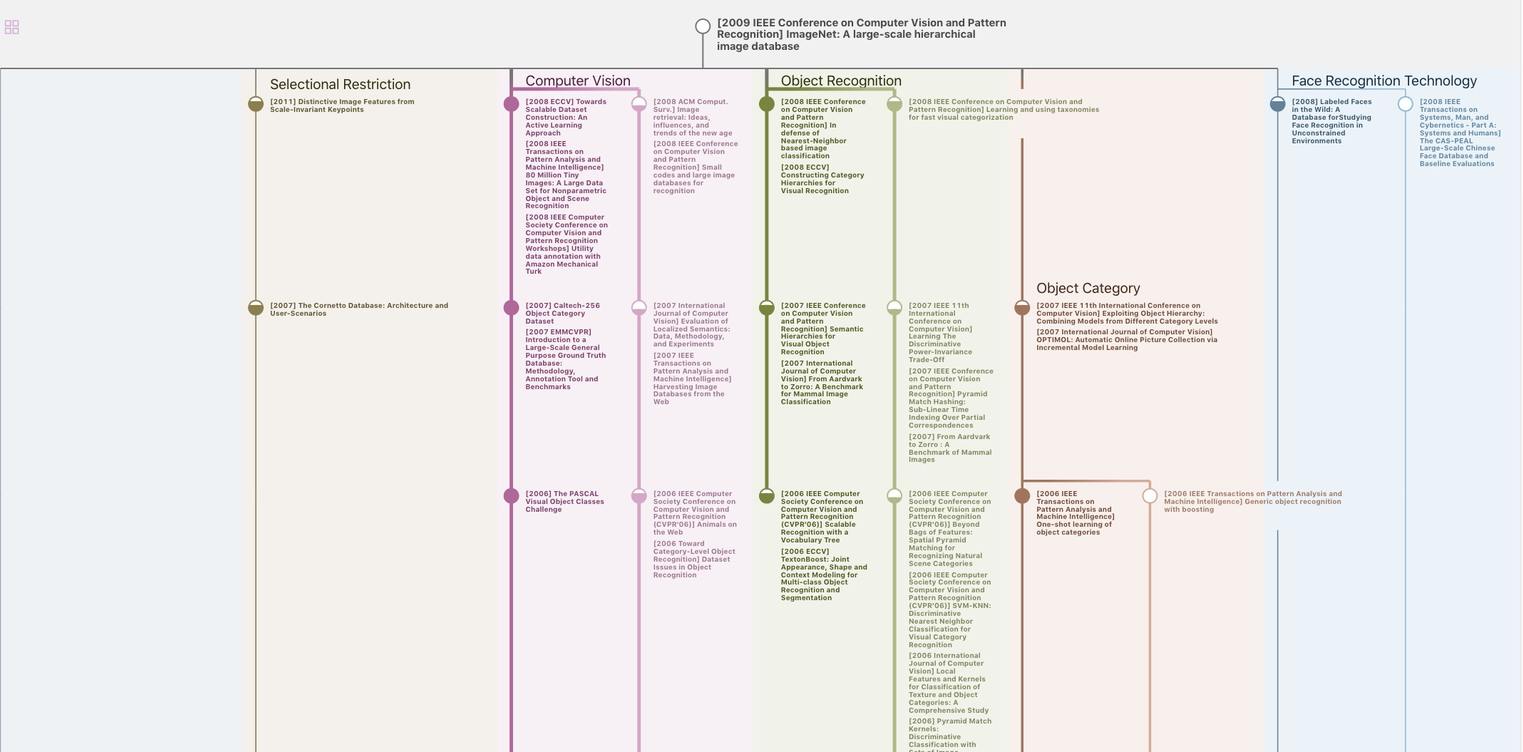
生成溯源树,研究论文发展脉络
Chat Paper
正在生成论文摘要