An Inferential Commonsense-Driven Framework for Predicting Political Bias in News Headlines.
IEEE Access(2023)
摘要
Identifying political bias in news headlines holds significant importance as it influences the dissemination and consumption of news stories. However, employing conventional methods to do so poses a formidable challenge, as the short headline text is often complex and lacks sufficient syntactic and semantic context. Existing approaches fail to acknowledge the potential of commonsense reasoning in facilitating text comprehension, although it has been shown to aid numerous downstream applications. To this end, to facilitate comprehension and compensate for the lack of context, we propose leveraging inferential commonsense knowledge to simplify, interpret, and explain events that are not explicitly stated in headlines. Furthermore, to fully utilise its potential and deal with the unnecessary noise it may introduce, we present a method for emphasising significant inferences. Using this knowledge, we introduce a novel framework, IC-BAIT, short for Inferential Commonsense aware BiAs IdenTifier, which is a flexible neural network framework designed to enhance political bias prediction in news headlines. We also present two bias-annotated datasets: MediaBias and GoodNews. Experiments on both datasets demonstrate that IC-BAIT significantly enhances the performance of the baseline models used in the framework. Experiments on the datasets show that IC-BAIT improves the baseline models in terms of accuracy (2.0-10.0%), macro-averaged F-1 (2.2-22.2%), Jaccard-score (up to 15.1%), and micro-averaged F-1 (up to 18.6%). Our in-depth qualitative analysis reveals the scenarios in which the selected knowledge is beneficial and when it is detrimental. Datasets and scripts are available at https://github.com/Swati17293/IC-BAIT.
更多查看译文
关键词
political bias,commonsense-driven
AI 理解论文
溯源树
样例
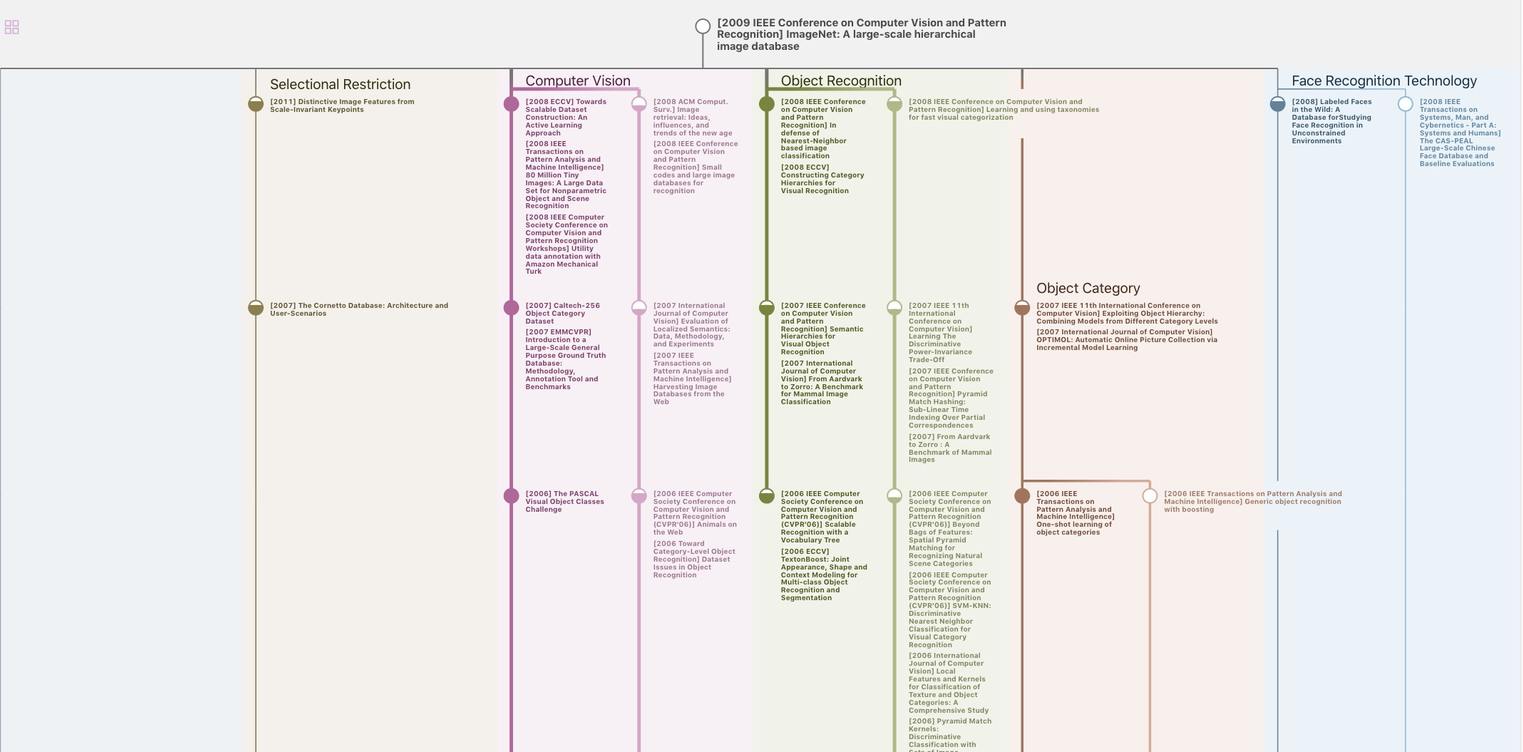
生成溯源树,研究论文发展脉络
Chat Paper
正在生成论文摘要