Design and Implementation of a Lightweight Deep CNN-Based Plant Biometric Authentication System.
IEEE Access(2023)
摘要
The wide application of personal biometric information such as face, fingerprint, iris, and voiceprint has simultaneously created many new ethical and legal issues, including the fraudulent use of biometrics. A non-human biometric system is demanded as an alternative, which features no human private information and can be replaced or renewed from time to time. The main objective of this study is to identify wood or leaf biometric patterns and verify their identities by building their respective datasets. On this basis, a plant biometric feature-based recognition system and authentication application were developed and implemented by employing a deep convolutional neural network (CNN) architecture to learn the embedding model using a distance-based triplet-loss similarity metric. We used two kinds of small datasets based on wood and leaves, which are Spruce Cross-Section (SCS) dataset and Collinsonia Canadensis Leaf Abaxial Surface (CCLAS) dataset. A series of artificial augmentations have been integrated into training to mimic the changes in the images during the usage of keys in real-world scenarios. The final results achieve accuracy values of 97.56% (validation set) and 96.06% (test set) on the Spruce Cross-Section (SCS) dataset and 99.11% (validation set) and 98.61% (test set) on the Collinsonia Canadensis Leaf Abaxial Surface (CCLAS) dataset, indicating the high reliability of this non-human biometric authentication system.
更多查看译文
关键词
plant,cnn-based
AI 理解论文
溯源树
样例
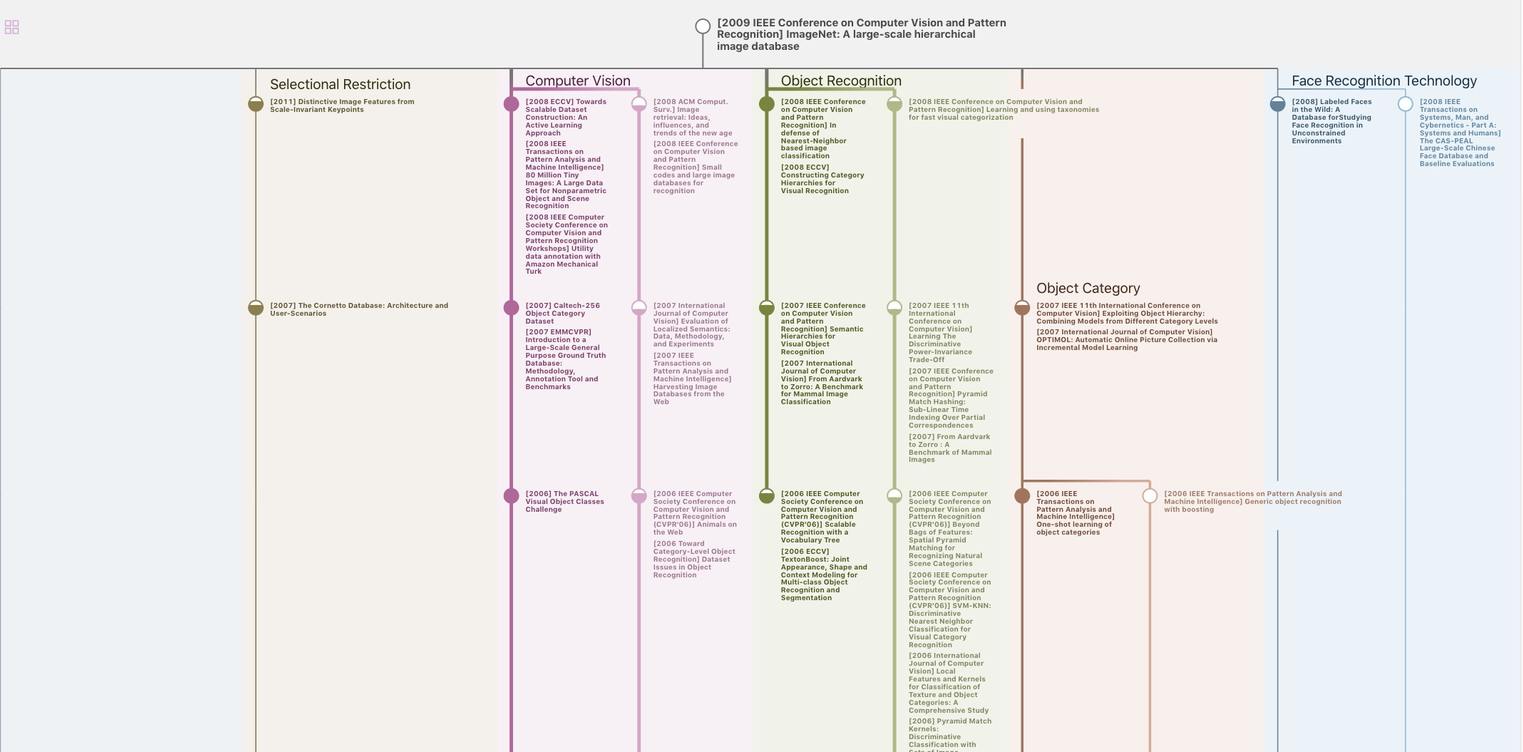
生成溯源树,研究论文发展脉络
Chat Paper
正在生成论文摘要