Cyberattack Graph Modeling for Visual Analytics.
IEEE Access(2023)
摘要
Cybersecurity research demands continuous monitoring of the dynamic threat landscape to detect novel attacks. Researchers and security professionals often deploy honeypot networks to intercept and examine real attack data. However, due to the volume and variety of the collected data, it is very challenging for security analysts to investigate the attacks, compare their characteristics and infer their potential connections. To this end, we propose a novel graph-based cyberattack model for storing, analyzing, and visualizing honeynet-captured attacks as the main contribution of our work. Our model enables attack graph analysis and presents the attack data analogous to the Cyber Kill Chain framework to enable intuitive visualizations. We construct the attack graph by decomposing the intercepted attacks into a set of unique entities (represented as nodes) and actions (represented as edges) and merge them into a global attack graph. We develop a user-centric, interactive attack analysis and visualization tool that leverages the proposed model to aid the heuristic cyberattack investigation. We describe the design and technical implementation of the developed model and visual-interactive tool in detail. Finally, we demonstrate the developed tools and validate the model in an analysis of real-world attack data captured on our own distributed honeypot platform. We use the attack model and (sub)graph visualizations to depict attack topologies, identify recurring attackers, and quantify detected malware types. We also leverage graph data science algorithms to uncover and rank malware distribution networks, reveal hidden links between the attackers, and cluster the attack entities to identify potential botnets.
更多查看译文
关键词
Attack modeling,attacker links,big data,botnet detection,cyberattack,cybersecurity
AI 理解论文
溯源树
样例
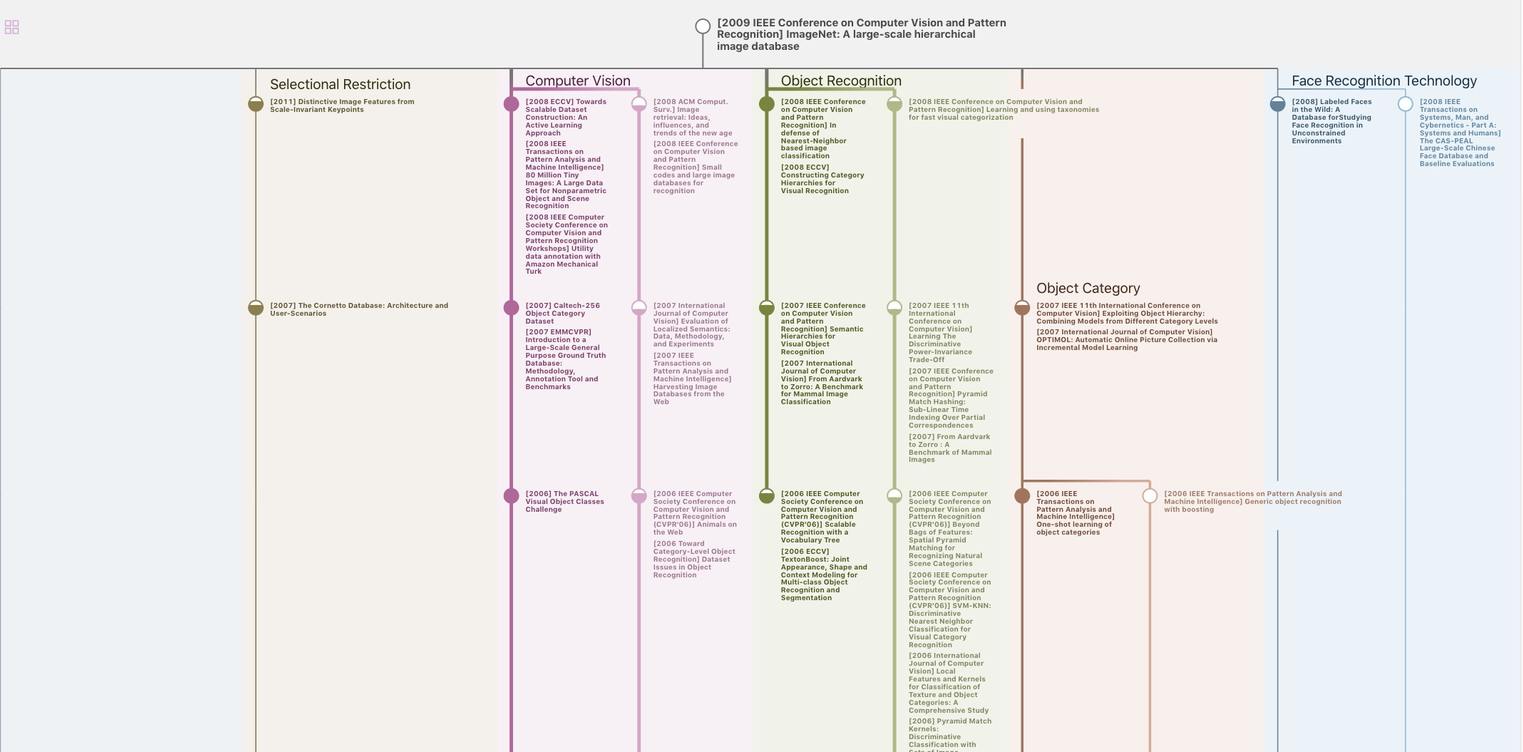
生成溯源树,研究论文发展脉络
Chat Paper
正在生成论文摘要