SalCor: A Hierarchical Saliency-Driven Segmentation Model With Local Correntropy for Medical Images
IEEE Access(2023)
摘要
In image segmentation, noise and nonuniform intensity can lead to performance degradation in existing models, particularly when dealing with shadow artifacts. This study proposes a hierarchical saliency-driven segmentation model with local correntropy (SalCor) to address this problem, incorporating saliency information with local correntropy-based K-means clustering to formulate an energy function. This approach enables it to extract objects with complex backgrounds effectively regardless of noise and intensity inhomogeneity. An adaptive weight function is introduced to dynamically adjust the intensities of the energy functions (external and internal) based on the image information, resulting in enhanced model resilience to contour initialization and improved robustness. The SalCor model can handle noise robustly by leveraging the local correntropy-based K-means clustering. The proposed approach is evaluated on synthetic and real images, including medical images, such as brain and mammogram magnetic resonance imaging (MRI) and coronavirus disease 2019 (COVID-19) computed tomography images, and is compared with state-of-the-art models. The statistical analysis confirms the SalCor model's exceptional precision and efficiency. These outcomes indicate that SalCor holds great potential for detecting brain tumors and mammogram tumors in MRIs and early diagnosis of COVID-19.
更多查看译文
关键词
Active contours,brain magnetic resonance imaging (MRI),coronavirus disease 2019 (COVID-19),image segmentation,level set,mammogram,medical image,saliency
AI 理解论文
溯源树
样例
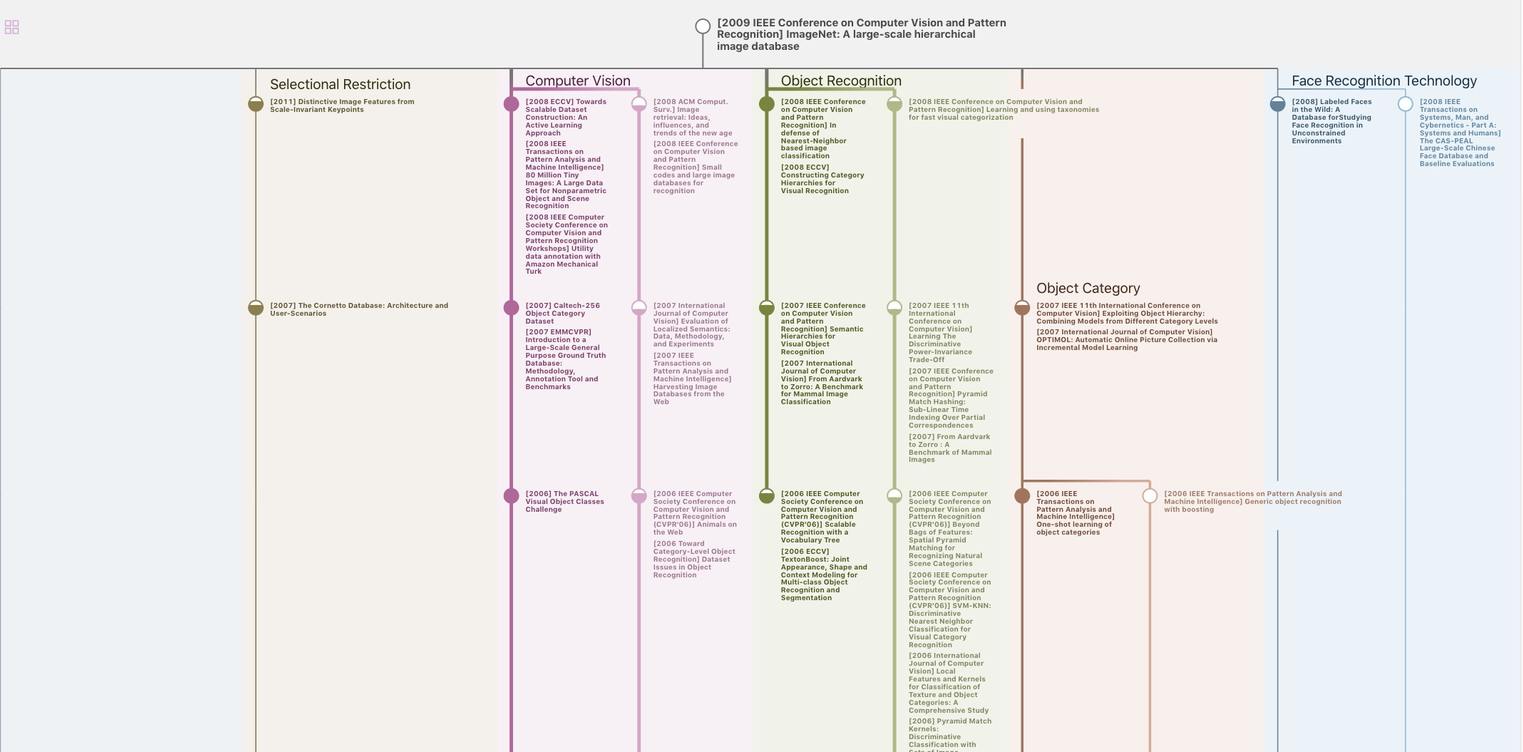
生成溯源树,研究论文发展脉络
Chat Paper
正在生成论文摘要