Learning-Based Adaptive Sliding-Window RLNC for High Bandwidth-Delay Product Networks
IEEE Access(2023)
摘要
Sliding-window random linear network coding (RLNC) is a good fit for achieving low in-order delivery delay in future-generation networks characterized by lossy links. In high bandwidth-delay product networks, however, the issue of integrating RLNC with transmission control protocol (TCP) flow and congestion control poses a significant challenge. In this paper, we propose an innovative reinforcement learning (RL) framework that addresses this issue by decoupling the RLNC sliding window from TCP and dynamically adjusting it to enhance network performance in terms of goodput, in-order delivery delay, and decoding complexity. By employing RL, we enable autonomous decision-making for adjusting the sliding window of RLNC, which operates independently of TCP. This decoupling allows RLNC to adapt dynamically to the varying conditions of the network, without prior knowledge of its characteristics. By leveraging the benefits of RLNC and TCP separately, we achieve more efficient and effective utilization of network resources. The results highlight significant improvements in goodput, in-order delivery delay, and decoding complexity. These improvements are crucial because in network coding, there is always a trade-off between goodput, delay, and decoding complexity, and minimizing this trade-off is very challenging. Using RL and decoupling of RLNC sliding window from TCP, we address this challenge and minimize the trade-off significantly. Goodput is improved by up to 11%, the in-order delivery delay is reduced by a factor of 9%, and coding complexity shows an improvement of up to 45% compared to the state-of-the-art.
更多查看译文
关键词
High BDP networks,in-order delay,reinforcement learning,RLNC,TCP sliding window
AI 理解论文
溯源树
样例
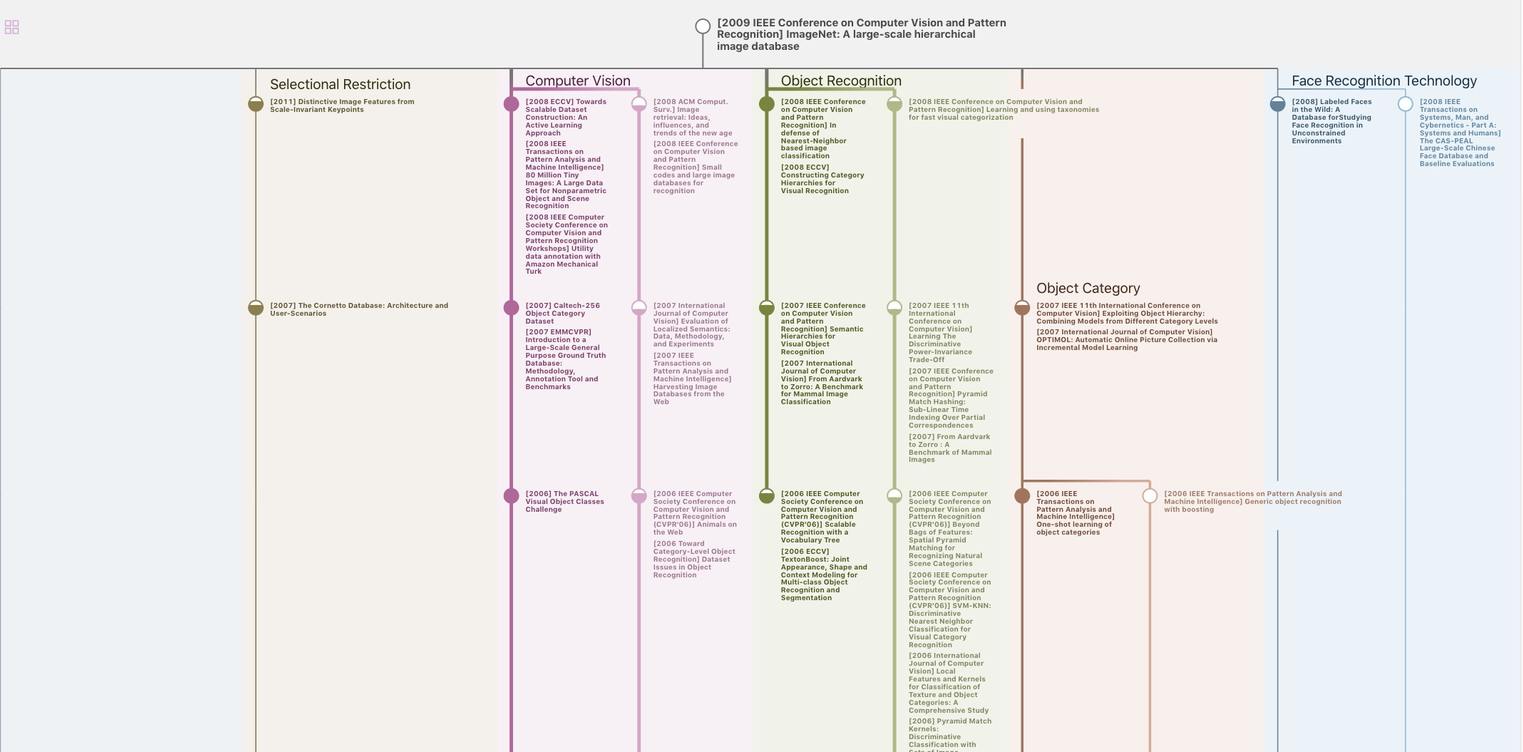
生成溯源树,研究论文发展脉络
Chat Paper
正在生成论文摘要