Early prediction of ransomware API calls behaviour based on GRU-TCN in healthcare IoT
Connect. Sci.(2023)
摘要
The healthcare industry is collecting considerable patient and medical data by using Internet of Things (IoT) devices. Consequently, ransomware attacks to encrypt healthcare systems or leak such data have increased recently. Many studies are aiming to predict ransomware behaviours early to protect the healthcare IoT environment from such attacks. However, previous studies analysed ransomware behaviours for long periods of time, and systems would already get infected and encrypted meanwhile. To avoid this problem, this study proposes an early prediction scheme of ransomware behaviour (EPS-Ran) to reduce the likelihood of systems being infected during behavioural analysis. EPS-Ran analyses behaviours for 30 s to extract the opcode and API calls sequence. The extracted behaviour features are entered into a hybrid deep learning model that combines the bidirectional gated recurrent unit (Bi-GRU) model and the temporal convolutional network (TCN) model to predict a future 90 s API calls sequence. The MAE, MSE, and RMSE of the prediction performance of EPS-Ran were measured to be 0.3438, 0.5648, and 0.6342, respectively. EPS-Ran predicted ransomware behaviours early with a low error rate even when the analysis time was reduced from 120 s to 30 s.
更多查看译文
关键词
Healthcare internet of things (IoT),ransomware,behaviour analysis,early prediction,deep learning,>
AI 理解论文
溯源树
样例
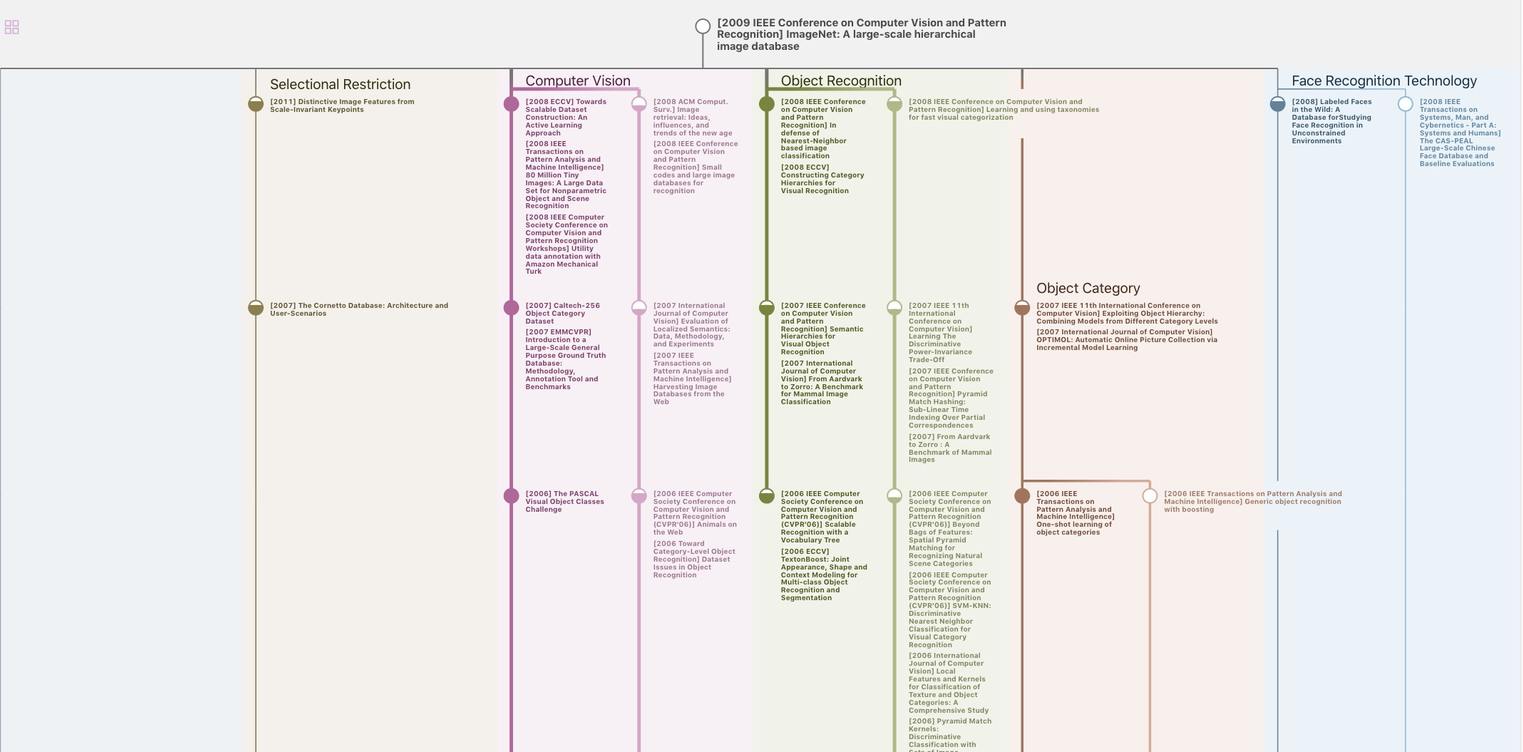
生成溯源树,研究论文发展脉络
Chat Paper
正在生成论文摘要