Patch-based camera-aware person-to-group learning and group similarity strategy for unsupervised group re-identification
Neurocomputing(2023)
摘要
In real life, people often go together in groups, which extends to an important but less researched task of computer vision: group re-identification. Differing from the related task of person re-identification, group reidentification involves unique challenges: self-occlusion, layout changes, membership changes, and the lack of labeled data. To solve these problems, we propose a patch-based camera-aware person-to-group unsupervised learning network. For the problem of high labeling costs, we train an unsupervised feature extraction network to extract the features of group members. Moreover, the feature extraction network is based on image patches, which solves the problem of group member alignment caused by occlusion within the group. To handle the large intra-ID variance resulting from changes in camera views, we add camera information to the model and propose the multi-grained camera-aware loss. For the problems of layout changes and membership changes, we adopt a person-to-group strategy. Specifically, we propose a novel group distance metric, named Modified Hausdorff Distance, to exploit the information of all the group members. Experimental results on two public datasets demonstrate the superiority of our approach.
更多查看译文
关键词
Group re-identification,Patch-based,Unsupervised,Camera-aware,Modified hausdorff distance
AI 理解论文
溯源树
样例
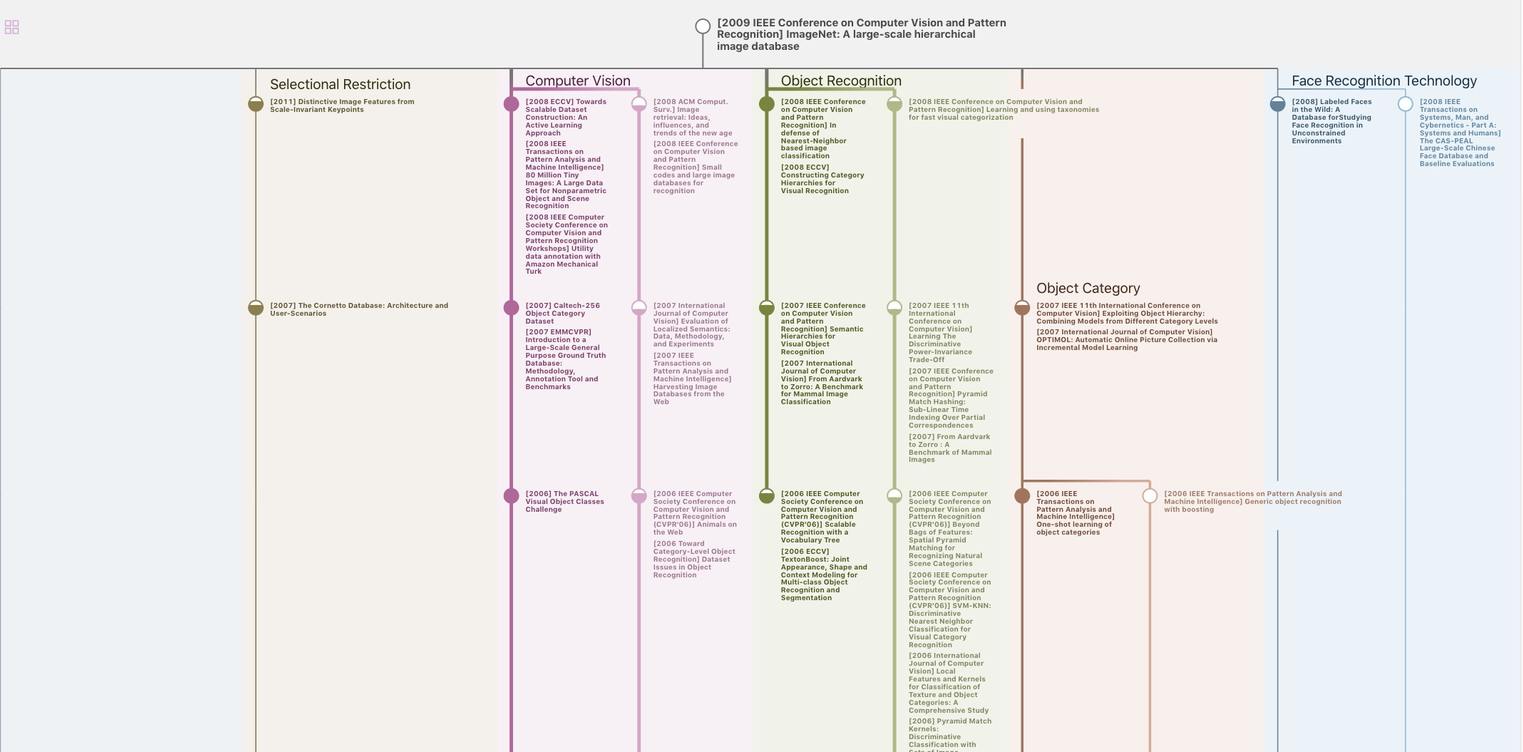
生成溯源树,研究论文发展脉络
Chat Paper
正在生成论文摘要