Consensus and Diffusion for First-Order Distributed Optimization Over Multi-Hop Network.
IEEE Access(2023)
摘要
Distributed optimization is a powerful paradigm to solve various problems in machine learning over networked systems. Existing first-order optimization methods perform cheap gradient descent by exchanging information per iteration only with single-hop neighbours in a network. However, in many agent networks such as sensor and robotic networks, it is prevalent that each agent can interact with other agents over multi-hop communication. Therefore, distributed optimization method over multi-hop networks is an important but overlooked topic that clearly needs to be developed. Motivated by this observation, in this paper, we apply multi-hop transmission to the outstanding distributed gradient descent (DGD) method and propose two typical versions (i.e., consensus and diffusion) of multi-hop DGD method, which we call CM-DGD and DM-DGD, respectively. Theoretically, we present the convergence guarantee of the proposed methods under mild assumptions. Moreover, we confirm that multi-hop strategy results in higher probability to improve the spectral gap of the underlying network, which has been shown to be a critical factor improving performance of distributed optimizations, thus achieves better convergence metrics. Experimentally, two distributed machine learning problems are picked to verify the theoretical analysis and show the effectiveness of CM-DGD and DM-DGD by using synthesized and real data sets.
更多查看译文
关键词
optimization,diffusion,first-order,multi-hop
AI 理解论文
溯源树
样例
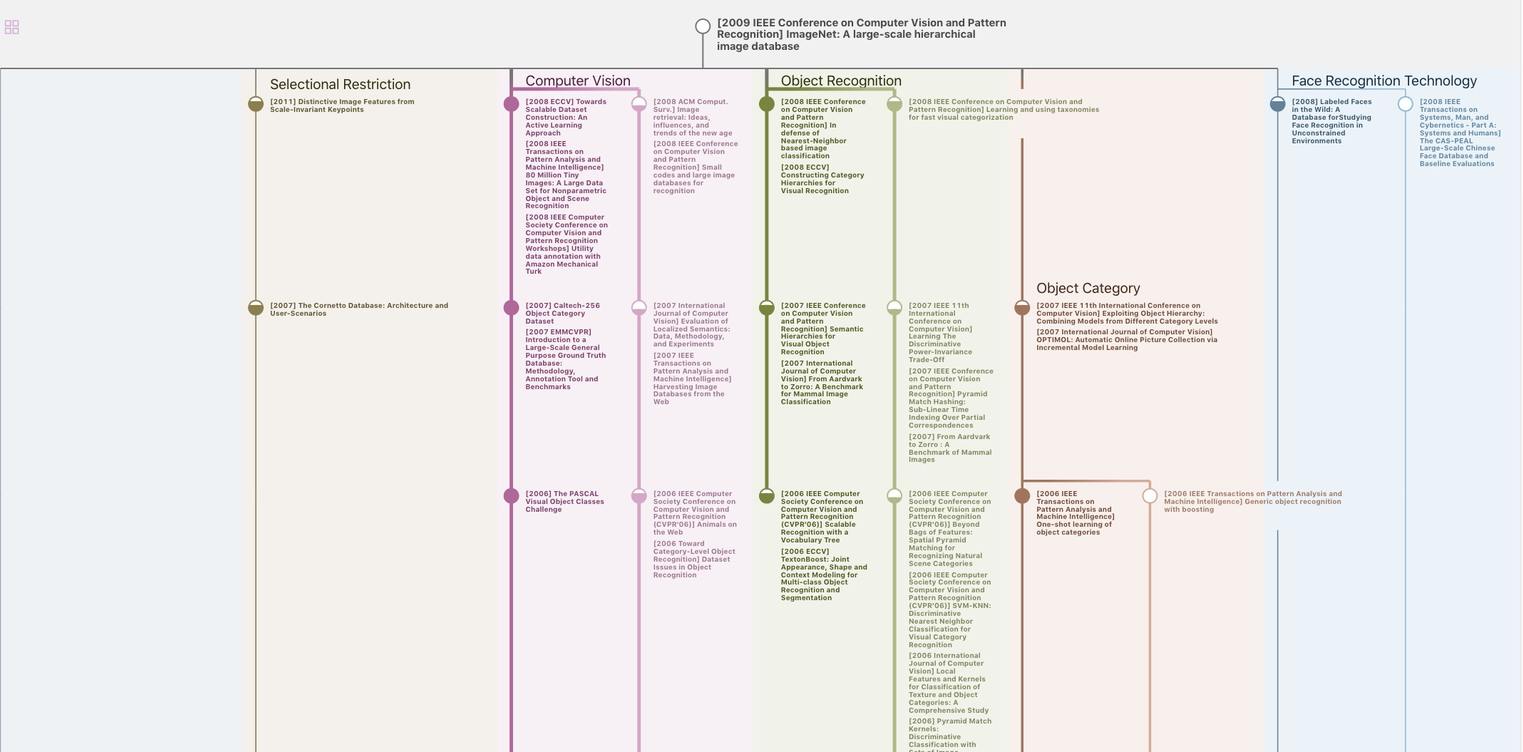
生成溯源树,研究论文发展脉络
Chat Paper
正在生成论文摘要