Adaptive transfer learning for PINN.
J. Comput. Phys.(2023)
摘要
Physics-informed neural networks (PINNs) have shown great potential in solving computa-tional physics problems with sparse, noisy, unstructured, and multi-fidelity data. However, the training of PINN remains a challenge, and PINN is not robust to deal with some com-plex problems, such as the sharp local gradient in broad computational domains, etc. Transfer learning techniques can provide fast and accurate training for PINN through in-telligent initialization, but the previous researches are much less effective when dealing with transfer learning cases with a large range of parameter variation, which also suffers from the same drawbacks. This manuscript develops the concept of the minimum energy path for PINN and proposes an adaptive transfer learning for PINN (AtPINN). The Partial Differential Equations (PDEs) parameters are initialized by the source parameters and up-dated adaptively to the target parameters during the training process, which can guide the optimization of PINN from the source to the target task. This process is essentially per-formed along a designed low-loss path, which is no barrier in the energy landscape of neural networks. Consequently, the stability of the training process is guaranteed. AtPINN is utilized to achieve transfer learning cases with a large range of parameter variation for solving five complex problems. The results demonstrate that AtPINN has promising po-tential for extending the application of PINN. Besides, three transfer learning cases with different ranges of parameter variation are analyzed through visualization. Furthermore, results also show that the idea of adaptive transfer learning can be a particular optimiza-tion strategy to directly solve problems without intelligent initialization. & COPY; 2023 Elsevier Inc. All rights reserved.
更多查看译文
关键词
Physical-informed neural networks, Transfer learning, Adaptive learning, Non-convex problem, The minimum energy path
AI 理解论文
溯源树
样例
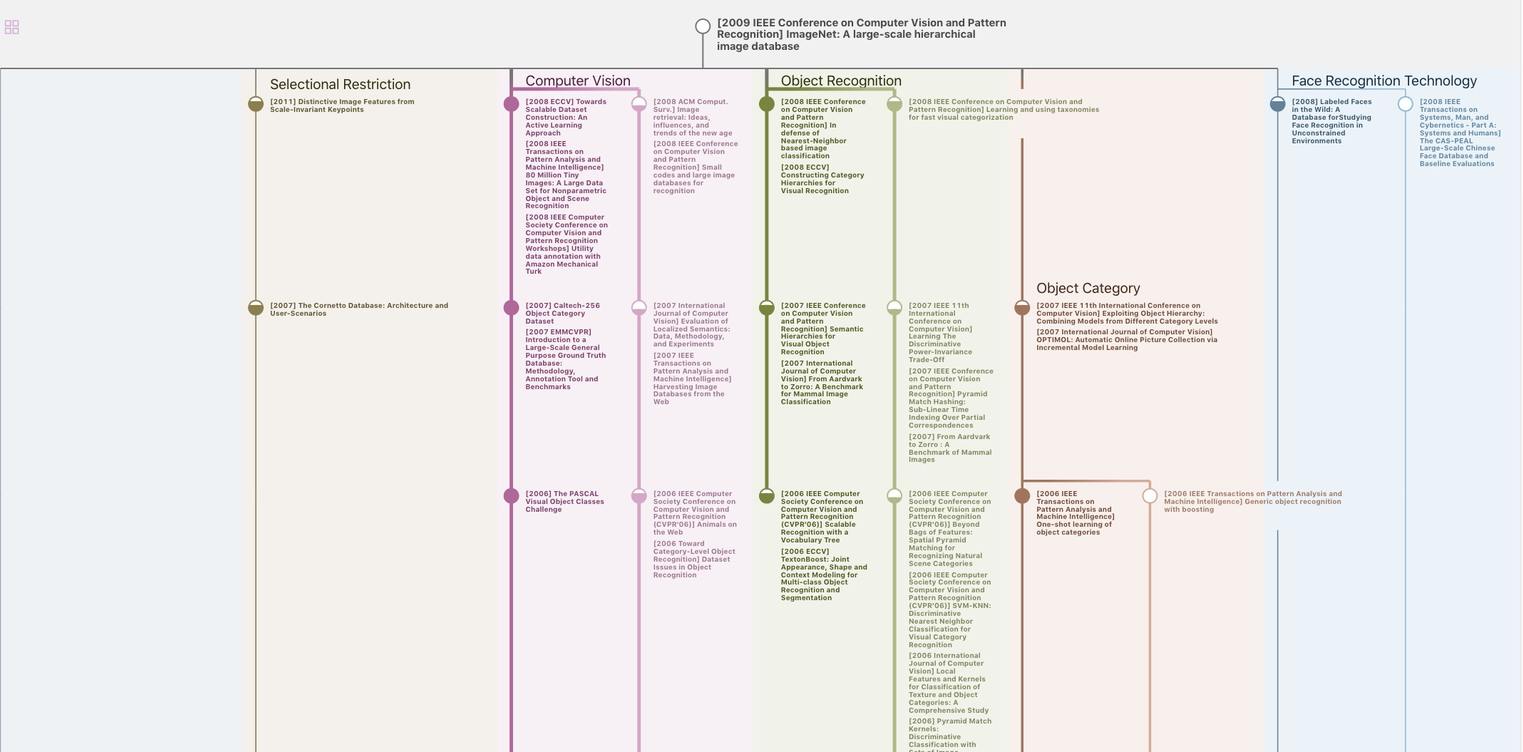
生成溯源树,研究论文发展脉络
Chat Paper
正在生成论文摘要