Bearing fault classification using TKEO statistical features and artificial intelligence.
J. Intell. Fuzzy Syst.(2023)
摘要
The study introduces a novel approach to classify faulty bearings using a combination of the Teager-Kaiser Energy Operator (TKEO) and Artificial Intelligence. The TKEO signal is used for statistical feature extraction to distinguish between healthy and abnormal bearings and two datasets were used to evaluate the proposed method. Total 11 statistical features were extracted from the raw and processed signals using the TKEO operator. The obtained feature set was used as input for various machine learning algorithms, and their performance was compared. Additionally, statistical features were calculated using the Hilbert Transform and compared to the proposed method. The study found that when the TKEO features were used as input for the classifier in the acoustic signal, the CART model achieved the highest accuracy of 99.62% compared to the raw and Hilbert transform signal features. In the case of vibration signals, the TKEO signal feature outperformed the raw signal feature with 100% accuracy for all artificial intelligence models. The proposed methodology revealed that using TKEO signal features as input significantly enhanced the classification accuracy.
更多查看译文
关键词
Statistical feature, hilbert transform, TKEO, artificial intelligence, CART
AI 理解论文
溯源树
样例
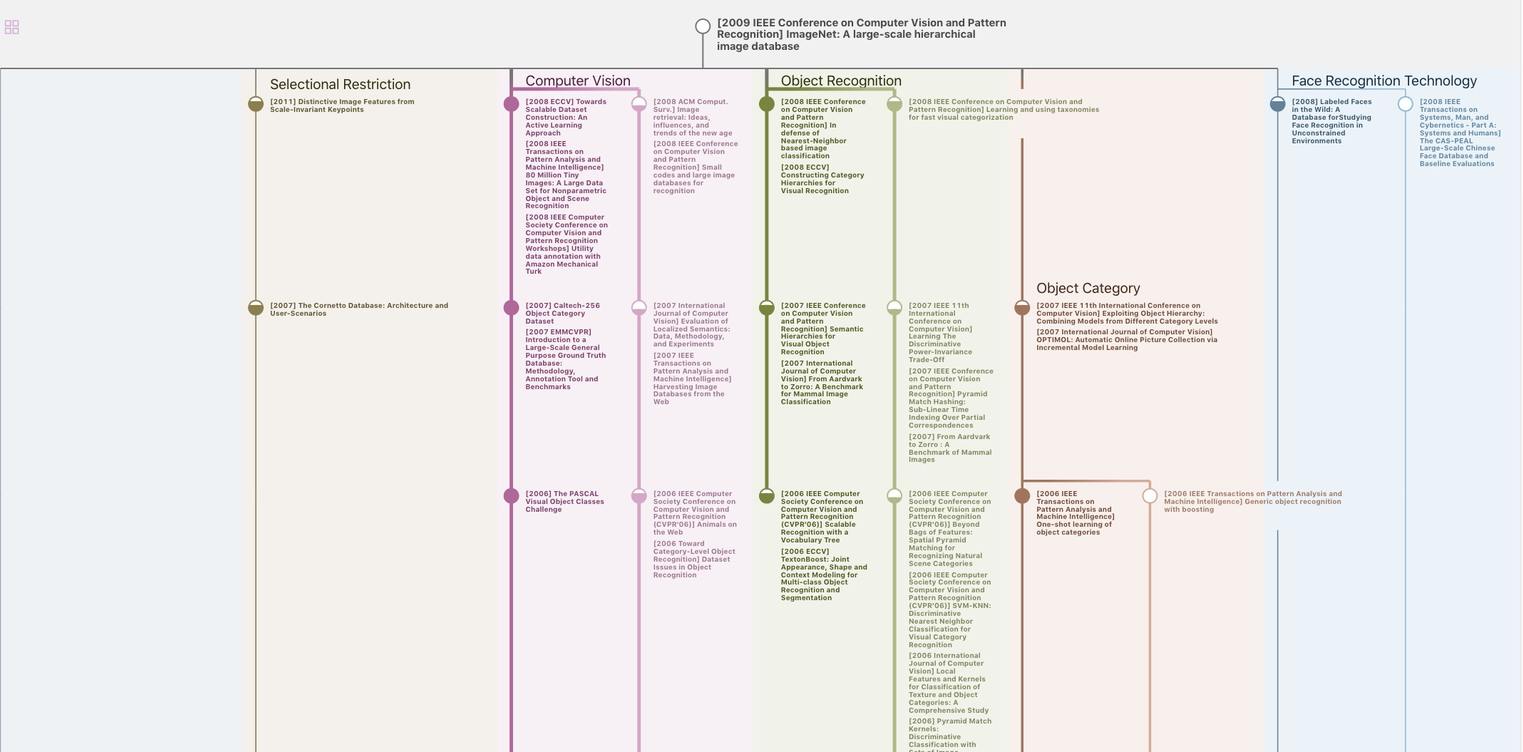
生成溯源树,研究论文发展脉络
Chat Paper
正在生成论文摘要