An approach for analysing the impact of data integration on complex network diffusion models.
J. Complex Networks(2023)
摘要
Complex networks are a powerful way to reason about systems with non-trivial patterns of interaction. The increased attention in this research area is accelerated by the increasing availability of complex network data sets, with data often being reused as secondary data sources. Typically, multiple data sources are combined to create a larger, fuller picture of these complex networks and in doing so scientists have to make sometimes subjective decisions about how these sources should be integrated. These seemingly trivial decisions can sometimes have significant impact on both the resultant integrated networks and any downstream network models executed on them. We highlight the importance of this impact in online social networks and dark networks, two use-cases where data are regularly combined from multiple sources due to challenges in measurement or overlap of networks. We present a method for systematically testing how different, realistic data integration approaches can alter both the networks themselves and network models run on them, as well as an associated Python package (NIDMod) that implements this method. A number of experiments show the effectiveness of our method in identifying the impact of different data integration setups on network diffusion models.
更多查看译文
关键词
diffusion,data integration,impact
AI 理解论文
溯源树
样例
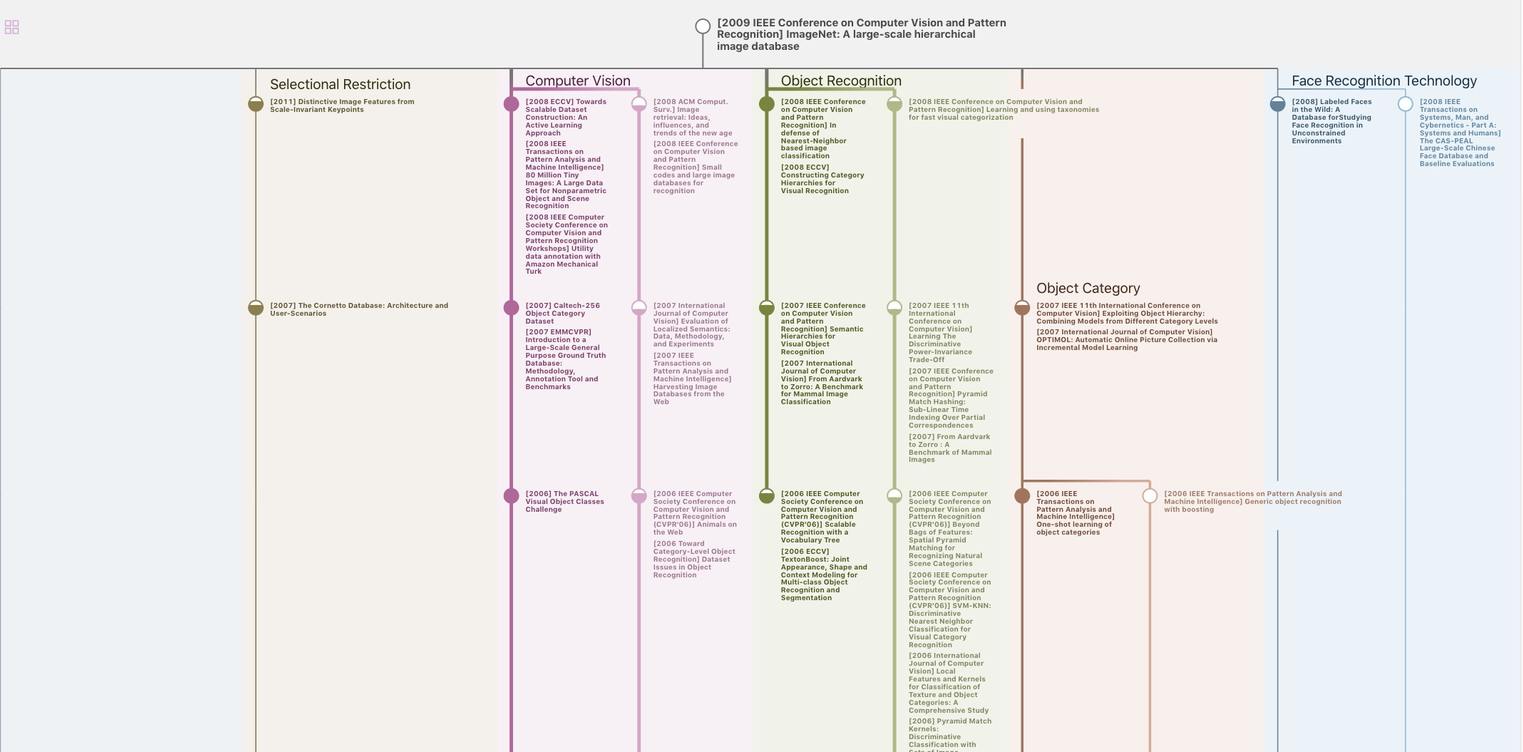
生成溯源树,研究论文发展脉络
Chat Paper
正在生成论文摘要