Lottery Ticket Search on Untrained Models with Applied Lottery Sample Selection.
Mach. Learn. Knowl. Extr.(2023)
摘要
In this paper, we present a new approach to improve tabular datasets by applying the lottery ticket hypothesis to tabular neural networks. Prior approaches were required to train the original large-sized model to find these lottery tickets. In this paper we eliminate the need to train the original model and discover lottery tickets using networks a fraction of the model's size. Moreover, we show that we can remove up to 95% of the training dataset to discover lottery tickets, while still maintaining similar accuracy. The approach uses a genetic algorithm (GA) to train candidate pruned models by encoding the nodes of the original model for selection measured by performance and weight metrics. We found that the search process does not require a large portion of the training data, but when the final pruned model is selected it can be retrained on the full dataset, even if it is often not required. We propose a lottery sample hypothesis similar to the lottery ticket hypotheses where a subsample of lottery samples of the training set can train a model with equivalent performance to the original dataset. We show that the combination of finding lottery samples alongside lottery tickets can allow for faster searches and greater accuracy.
更多查看译文
关键词
selection,untrained models,search,sample
AI 理解论文
溯源树
样例
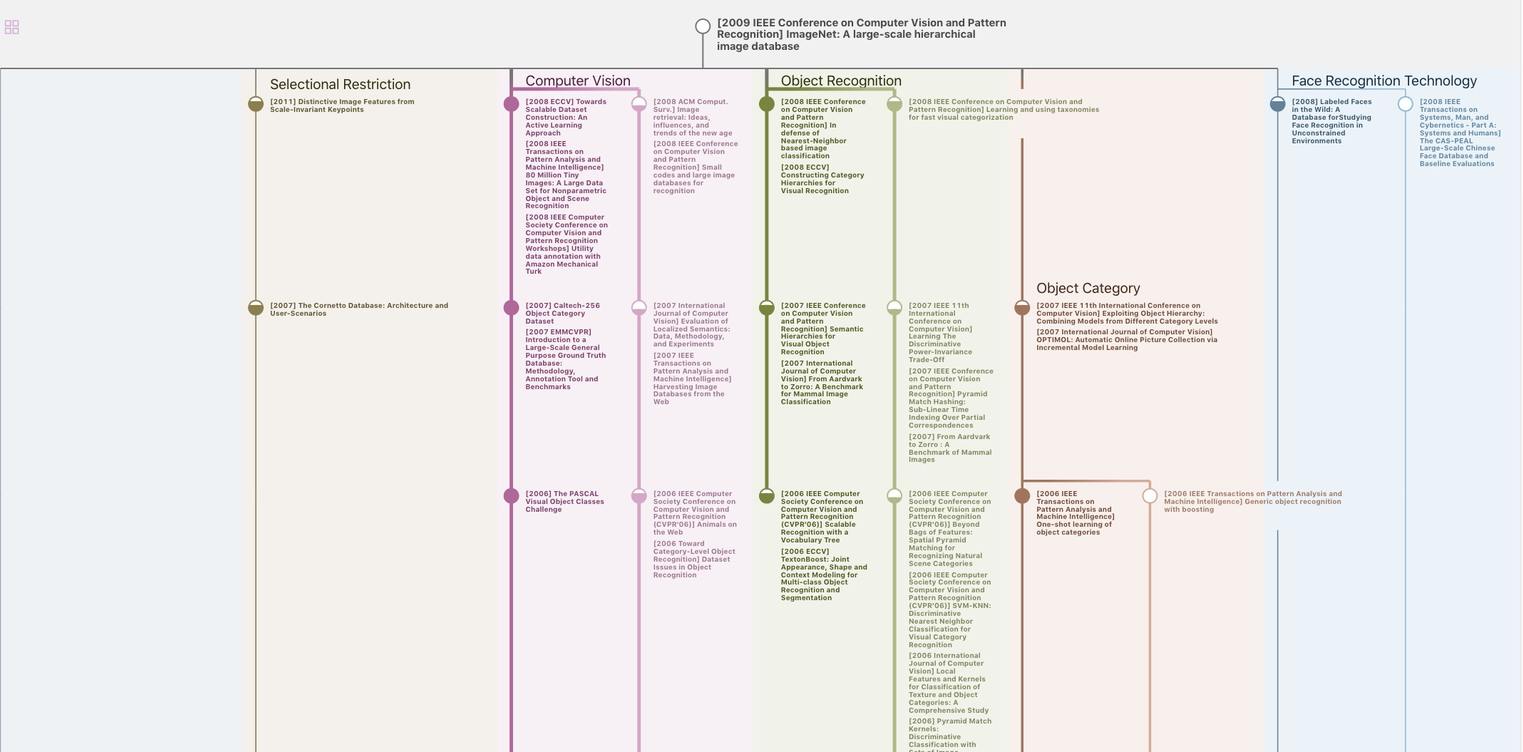
生成溯源树,研究论文发展脉络
Chat Paper
正在生成论文摘要