Yolo-MSAPF: Multiscale Alignment Fusion With Parallel Feature Filtering Model for High Accuracy Weld Defect Detection.
IEEE Trans. Instrum. Meas.(2023)
摘要
This work aims to improve the low accuracy caused by interference information during real-time weld surface detection. First, a weld surface dataset with 7580 pictures containing eight types of defects was established. Second, an improved detection model named Yolo-MSAPF was designed based on Yolo-v5 model and verified by the self-established database. Finally, a real-time detection system was built to analyze the performance of the detection model in an industrial environment. The design principle of the Yolo-MSAPF is to eliminate interference information but enhance necessary features in each scale as much as possible by multiscale alignment fusion (MSAF) with parallel features filtering (PFF) modules (i.e., MSAPF strategy). For the MSAF, not only the accuracy but the richness of fused features is guaranteed by aligning features at one level to fuse all other scales. After that, the fused features in each scale were individually filtered out in parallel spatial and channel in the PFF module. The results show that rate for the images with missing detection for eight types of defects sharply drops from 21.47% to 1.68% when the MSAPF strategy is used. Moreover, it is worth noting that the mAP@0.5 of the Yolo-MSAPF model reaches 95.3%, which is similar to the Yolo-v7 model, while the number of parameters is reduced by 30.1%, compared with the baseline model. Additionally, the great ability to screen out unqualified weld is also verified in the industrial environment. Soon, code and dataset will be available at https://github.com/Luckycat518/Yolo-MSAPF.
更多查看译文
关键词
multiscale alignment fusion,parallel feature filtering model,yolo-msapf
AI 理解论文
溯源树
样例
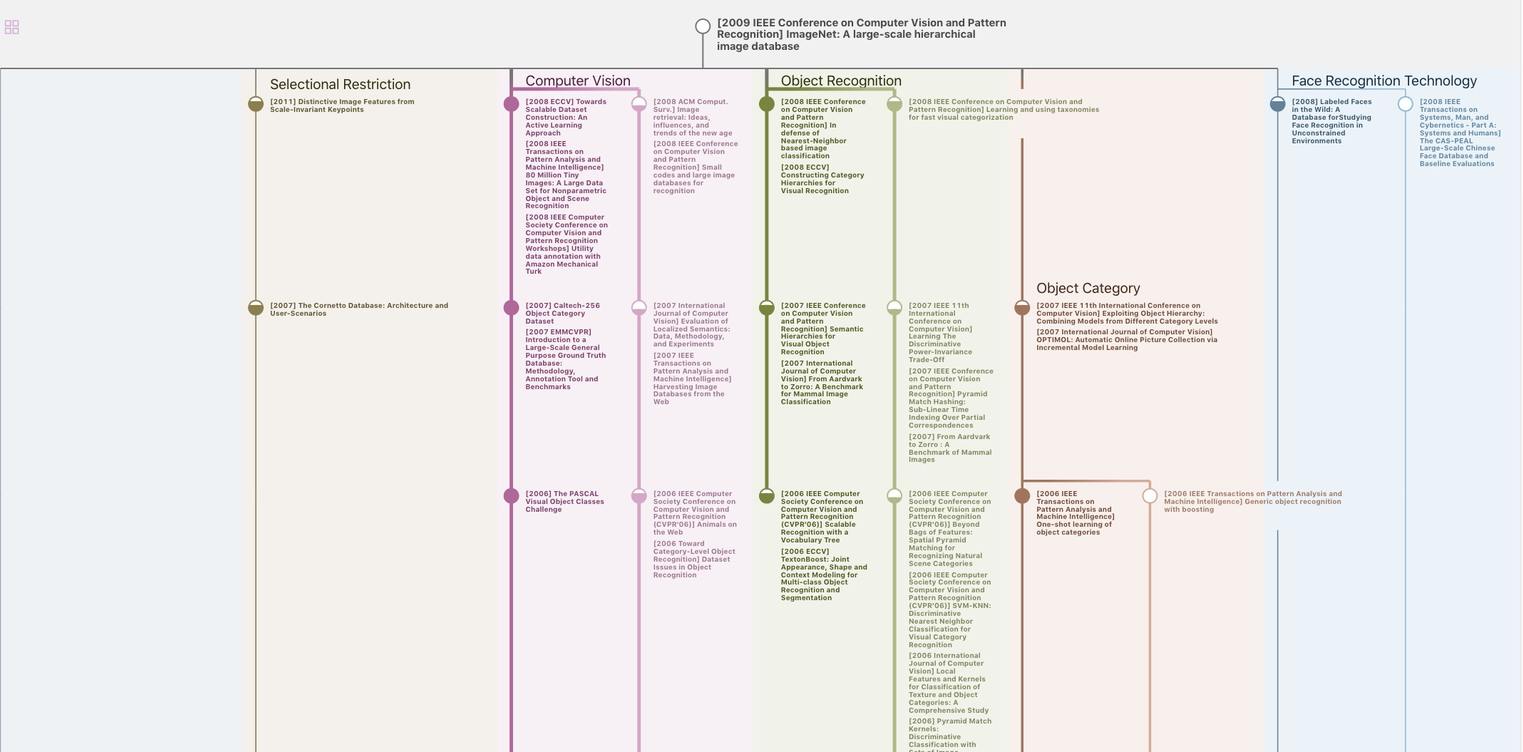
生成溯源树,研究论文发展脉络
Chat Paper
正在生成论文摘要