WNet: W-Shaped Hierarchical Network for Remote-Sensing Image Change Detection.
IEEE Trans. Geosci. Remote. Sens.(2023)
摘要
Change detection (CD) is a hot research topic in the remote-sensing (RS) community. With the increasing availability of high-resolution (HR) RS images, there is a growing demand for CD models with high detection accuracy and generalization ability. In other words, the CD models are expected to work well for various HRRS images. Convolutional neural networks (CNNs) have been dominated in HRRS image CD due to their excellent information extraction and nonlinear fitting capabilities. However, they are not skilled in modeling long-range contexts hidden in HRRS images, which limits their performance in CD tasks more or less. Recently, the Transformer, which is good at extracting global context dependencies, has become popular in the RS community. Nevertheless, detailed local knowledge receives insufficient emphasis in common Transformers. Considering the above discussion, we combine CNNs and Transformers and propose a new W-shaped dual-Siamese branch hierarchical network for HRRS image CD named W-shaped hierarchical network (WNet). WNet first incorporates a Siamese CNN and a Siamese Transformer into a dual-branch encoder to extract multilevel local fine-grained features and global long-range contextual dependencies. Also, we introduce deformable ideas into the Siamese CNN and Transformer to make WNet understand the critical and irregular areas within HRRS images. Second, the difference enhancement module (DEM) is developed and embedded into the encoder to produce the difference feature maps at different levels. Using simple pixel-wise subtraction and channel-wise concatenation, the changes of interest and irrelevant changes can be highlighted and suppressed in a learnable manner. Next, the multilevel difference feature maps are fused stage by stage by CNN-Transformer fusion modules (CTFMs), which are the basic units of the decoder in WNet. In CTFMs, the local, global, and cross-scale clues are taken into account to ensure the integrity of information. Finally, a simple classifier is constructed and added at the top of the decoder to predict the change maps. Positive experimental results counted on four public datasets demonstrate that the proposed WNet is helpful in HRRS image CD tasks. Our source codes are available at https://github.com/TangXu-Group/Remote-Sensing-Image-Change-Detection/tree/main/WNet.
更多查看译文
关键词
remote-sensing remote-sensing,wnet,hierarchical network,detection,w-shaped
AI 理解论文
溯源树
样例
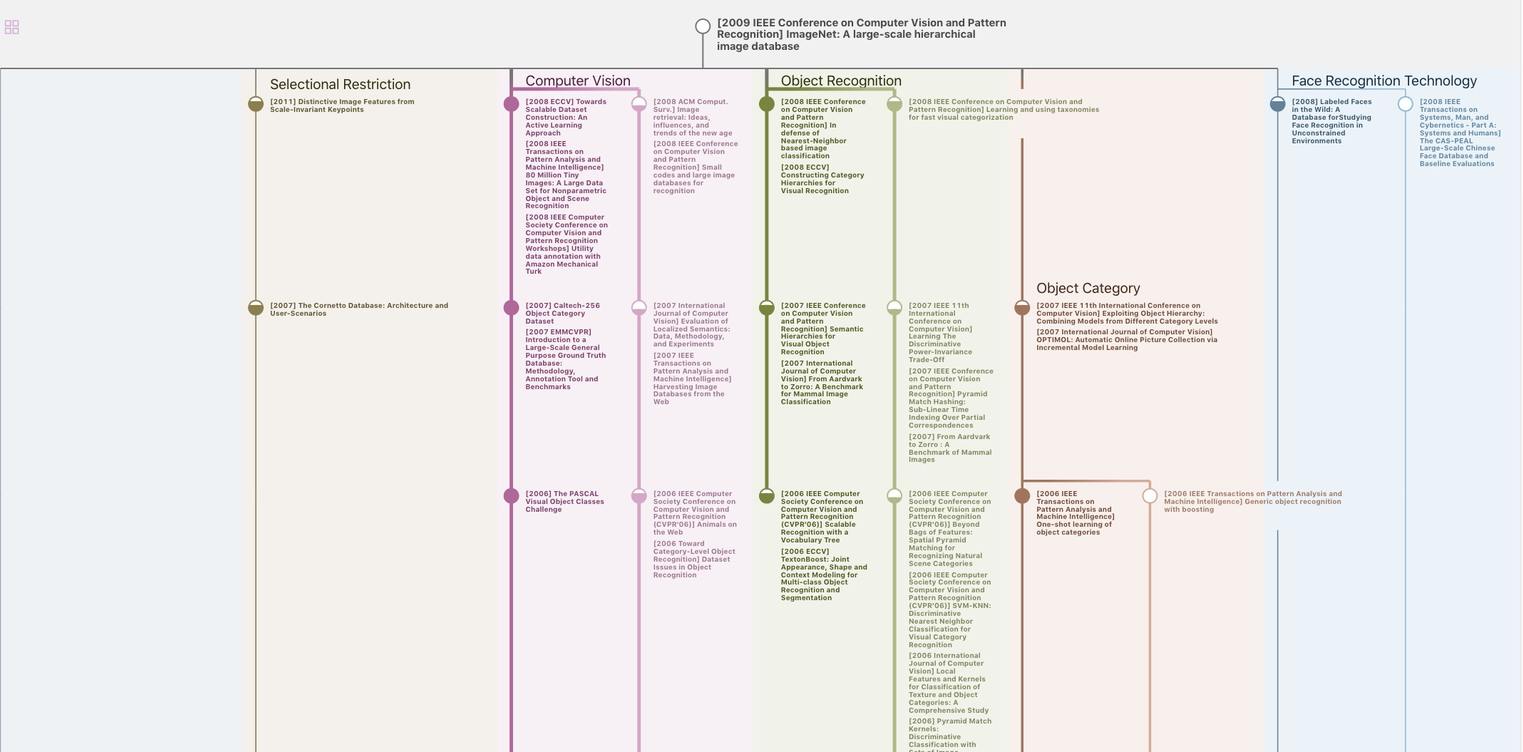
生成溯源树,研究论文发展脉络
Chat Paper
正在生成论文摘要