Boosting Semantic Segmentation of Aerial Images via Decoupled and Multilevel Compaction and Dispersion.
IEEE Trans. Geosci. Remote. Sens.(2023)
摘要
Semantic segmentation is a valuable task in practical applications for aerial images. Nevertheless, the segmentation performance is unsatisfactory due to aerial images' huge intraclass variance and interclass similarity. To solve this problem, we propose an approach to increase the distinction between classes and compact the features of the same class. Specifically, since a single aerial image contains only a small number of categories, which is fatal for previous contrastive learning, we discard InfoNCE loss in contrastive learning and use the simple mean square error (mse) loss that does not require negative samples to decouple the dispersion and compaction operations. Besides, we set up more representative prototypes for classes and extend the prototypes to the whole dataset level, which we call image- and dataset-level prototypes. Based on the calculated prototypes, we propose multilevel intraclass feature compaction (MFC) and multilevel interclass feature dispersion (MFD) to compact the features of the same class and disperse the features of different classes in the latent feature space. More importantly, some measures are proposed to ensure the two do not conflict. MFC and MFD can be applied to any existing segmentation network to improve performance significantly without increasing computational complexity during inference. Moreover, we feed the calculated multilevel prototypes directly into the classifier, thus keeping the feature extraction and classifier consistent. Results on four challenging datasets, Deepglobe, iSAID, Potsdam, and Vaihingen, demonstrate the significant effect of our method, and sufficient ablation studies verify the role of each module.
更多查看译文
关键词
Aerial image,multilevel feature interclass dispersion,multilevel feature intraclass compaction,semantic segmentation
AI 理解论文
溯源树
样例
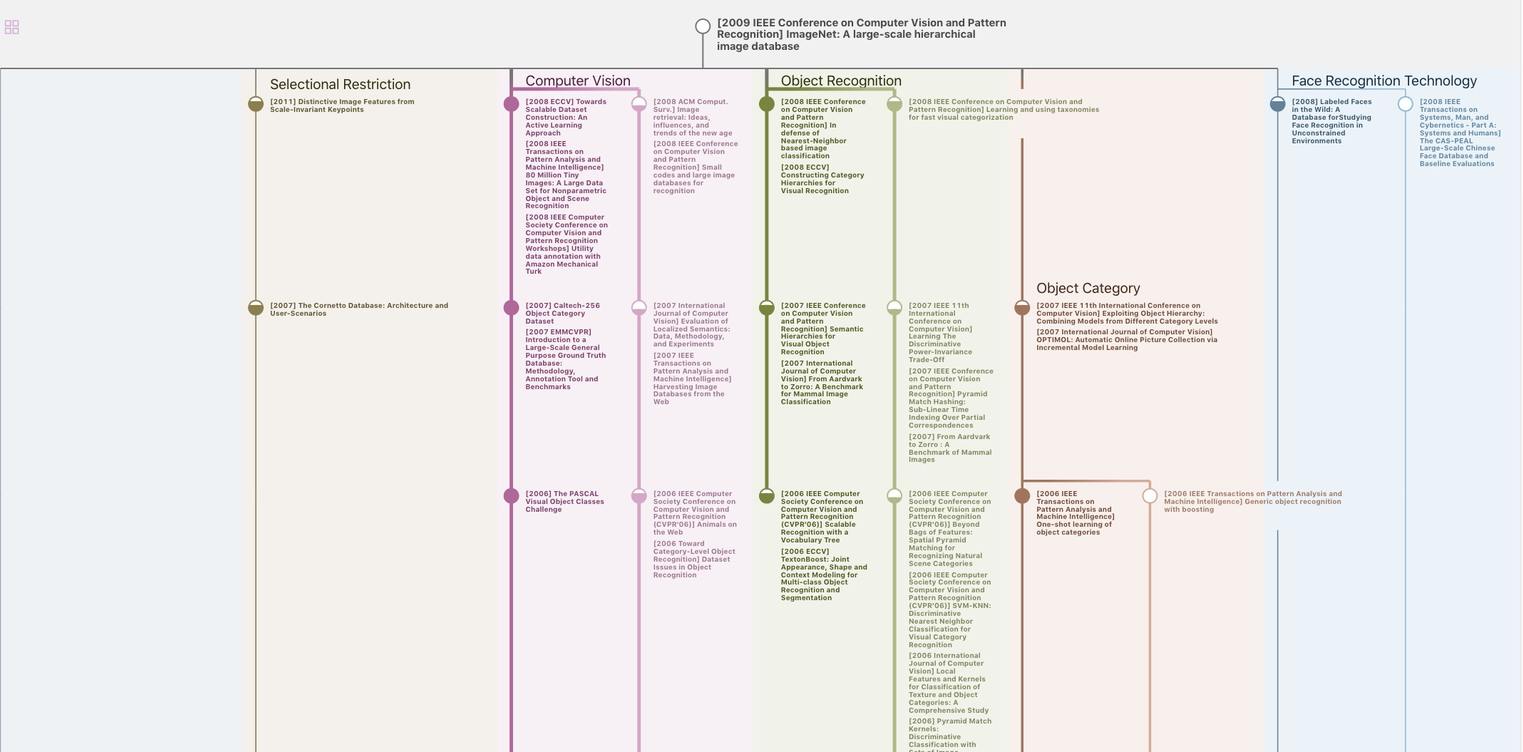
生成溯源树,研究论文发展脉络
Chat Paper
正在生成论文摘要