X-Shaped Interactive Autoencoders With Cross-Modality Mutual Learning for Unsupervised Hyperspectral Image Super-Resolution.
IEEE Trans. Geosci. Remote. Sens.(2023)
摘要
Hyperspectral image super-resolution (HSI-SR) can compensate for the incompleteness of single-sensor imaging and provide desirable products with both high spatial and spectral resolution. Among them, unmixing-inspired networks have drawn considerable attention due to their straightforward unsupervised paradigm. However, most do not fully capture and utilize the multimodal information due to their limited representation ability of constructed networks, hence leaving large room for further improvement. To this end, we propose an X-shaped interactive autoencoder network with cross-modality mutual learning between hyperspectral and multispectral data, XINet for short, to cope with this problem. Generally, it employs a coupled structure equipped with two autoencoders, aiming at deriving latent abundances and corresponding endmembers from input correspondence. Inside the network, a novel X-shaped interactive architecture is designed by coupling two disjointed U-Nets together via a parameter-shared strategy, which not only enables sufficient information flow between two modalities but also leads to informative spatial-spectral features. Considering the complementarity across each modality, a cross-modality mutual learning module (CMMLM) is constructed to further transfer knowledge from one modality to another, allowing for better utilization of multimodal features. Moreover, a joint self-supervised loss is proposed to effectively optimize our proposed XINet, enabling an unsupervised manner without external triplets supervision. Extensive experiments, including super-resolved results in four datasets, robustness analysis, and extension to other applications, are conducted, and the superiority of our method is demonstrated.
更多查看译文
关键词
Hyperspectral image (HSI),spectral unmixing,super-resolution,unsupervised learning
AI 理解论文
溯源树
样例
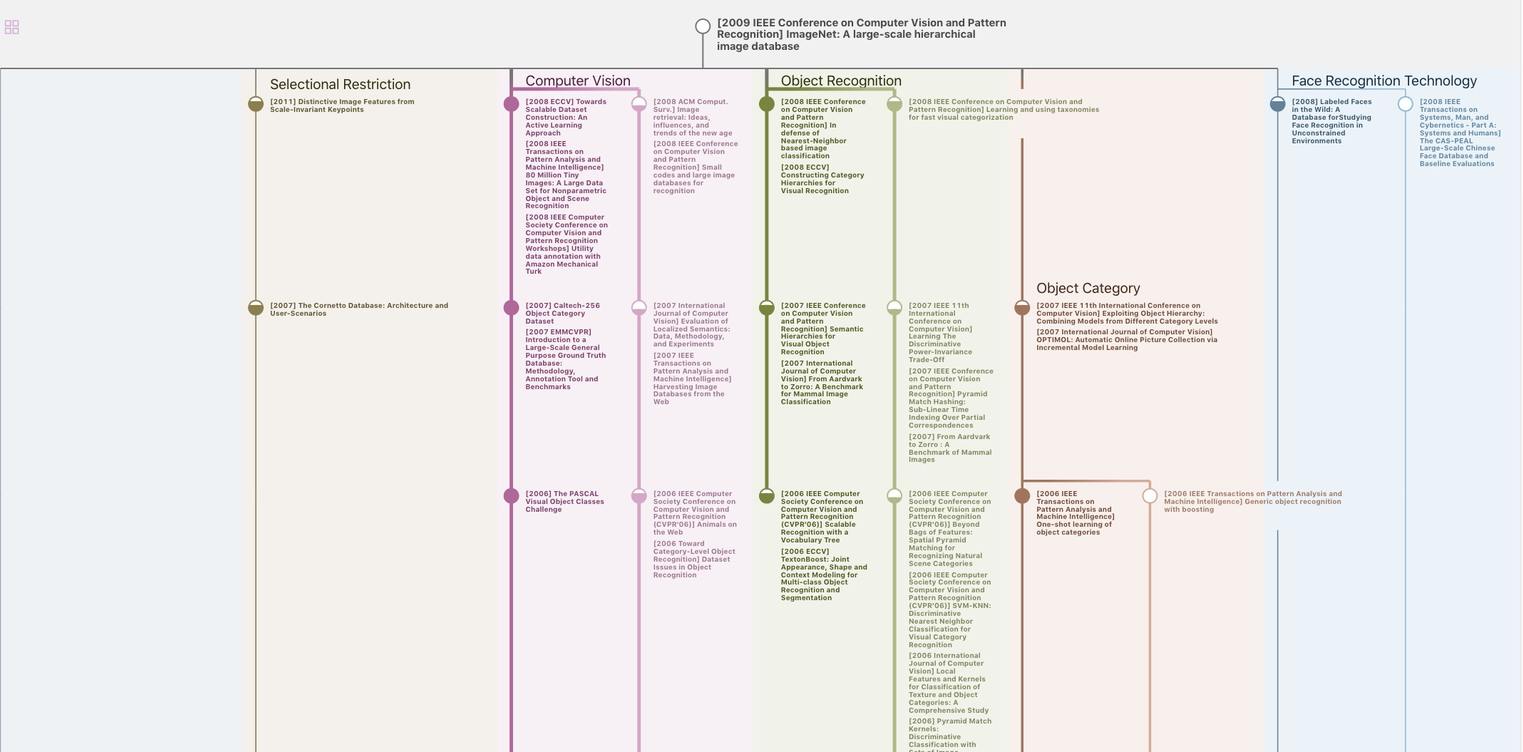
生成溯源树,研究论文发展脉络
Chat Paper
正在生成论文摘要