Seismic Data Reconstruction Based on Multiscale Attention Deep Learning
IEEE Trans. Geosci. Remote. Sens.(2023)
摘要
Seismic data reconstruction is always an essential step in the field of seismic data processing. Effective reconstruction methods can obtain high-density information at low-cost and also recover missing seismic data. Due to the strong feature extraction ability, convolutional neural network (CNN) has shown remarkable performance in numerous fields of data processing and been gradually applied to seismic data reconstruction. However, most of the CNN-based methods applied to seismic data reconstruction only consider features in single scale or just utilize simple interactions between different scales, which are likely to result in performance degradation when facing complex and extremely incomplete seismic data. To further promote the performance of CNN-based methods in seismic data reconstruction, a novel multiscale enhanced attention network (MSEA-Net) is proposed based on the self-enhanced scheme. In general, MSEA-Net has a multiscale architecture which can significantly improve the processing accuracy by fusing the potential features in different-resolution seismic data. From the basis, a parallel sparse residual (PSR) block is designed and applied in MSEA-Net to enhance processing efficiency and avoid overfitting issues. In addition, a dense spatial attention (DSA) block is also introduced to the network to further reinforce the effective features, thereby strengthening the reconstruction performance. Experimental results demonstrate that our proposed network can effectively reconstruct incomplete seismic data including regular missing data, irregular missing data, and even consecutively missing data with big gap, which is superior to existing interpolation methods including commonly used U-Net.
更多查看译文
关键词
Convolutional neural network (CNN),seismic data reconstruction,seismic exploration,signal processing
AI 理解论文
溯源树
样例
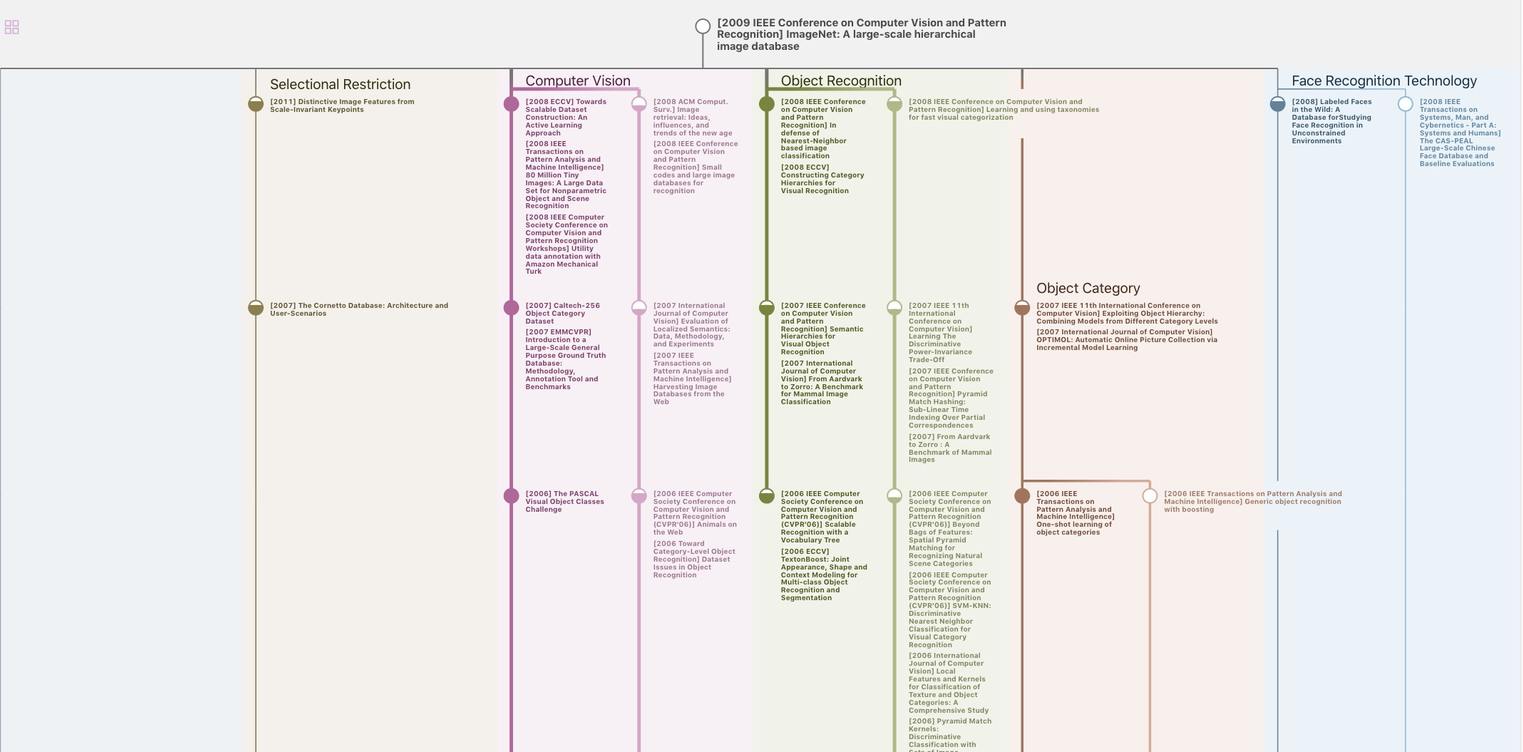
生成溯源树,研究论文发展脉络
Chat Paper
正在生成论文摘要