Learning Deep Multiscale Local Dissimilarity Prior for Pansharpening
IEEE Trans. Geosci. Remote. Sens.(2023)
摘要
Various deep neural networks (DNNs) have been constructed to inject the spatial information of the panchromatic (PAN) image into the low spatial resolution multispectral (LR MS) image. However, most of them ignore the local dissimilarity (LD) prior between MS and PAN images, which has a negative influence on the fused image. Considering the above-mentioned issues, we propose a deep multiscale LD network (DMLD-Net) to learn the LD prior at different scales and enhance the spatial and spectral information in the fused image better. Specifically, we first synthesize a downsampled PAN image from the original PAN image to match the scale of the LR MS image. Then, an LD metric is designed to calculate the dissimilarity map between the two images in feature space. According to the learned dissimilarity map, we use an LD-guided attention block (LDGAB) to suppress the impact of LD, which filters out the dissimilar information in the features of the PAN image. To learn the LD prior between MS and PAN images sufficiently, the multiscale architecture is considered and we infer the dissimilar maps hierarchically and inject filtered features into the LR MS image progressively. Finally, the fused image is generated by a reconstruction block. Through the LD learning at different scales, reasonable spatial information is extracted from the PAN image, by which the distortions in the fused image caused by LD can be reduced efficiently. Extensive experiments are conducted on the GeoEye-1 and WorldView-2 datasets, and the results demonstrate the effectiveness of the proposed DMLD-Net in terms of spatial and spectral preservation. The code is available at https://github.com/RSMagneto/DMLD-Net.
更多查看译文
关键词
Deep multiscale network,local dissimilarity (LD),multispectral image,panchromatic (PAN) image,pansharpening (PNN),remote sensing image fusion
AI 理解论文
溯源树
样例
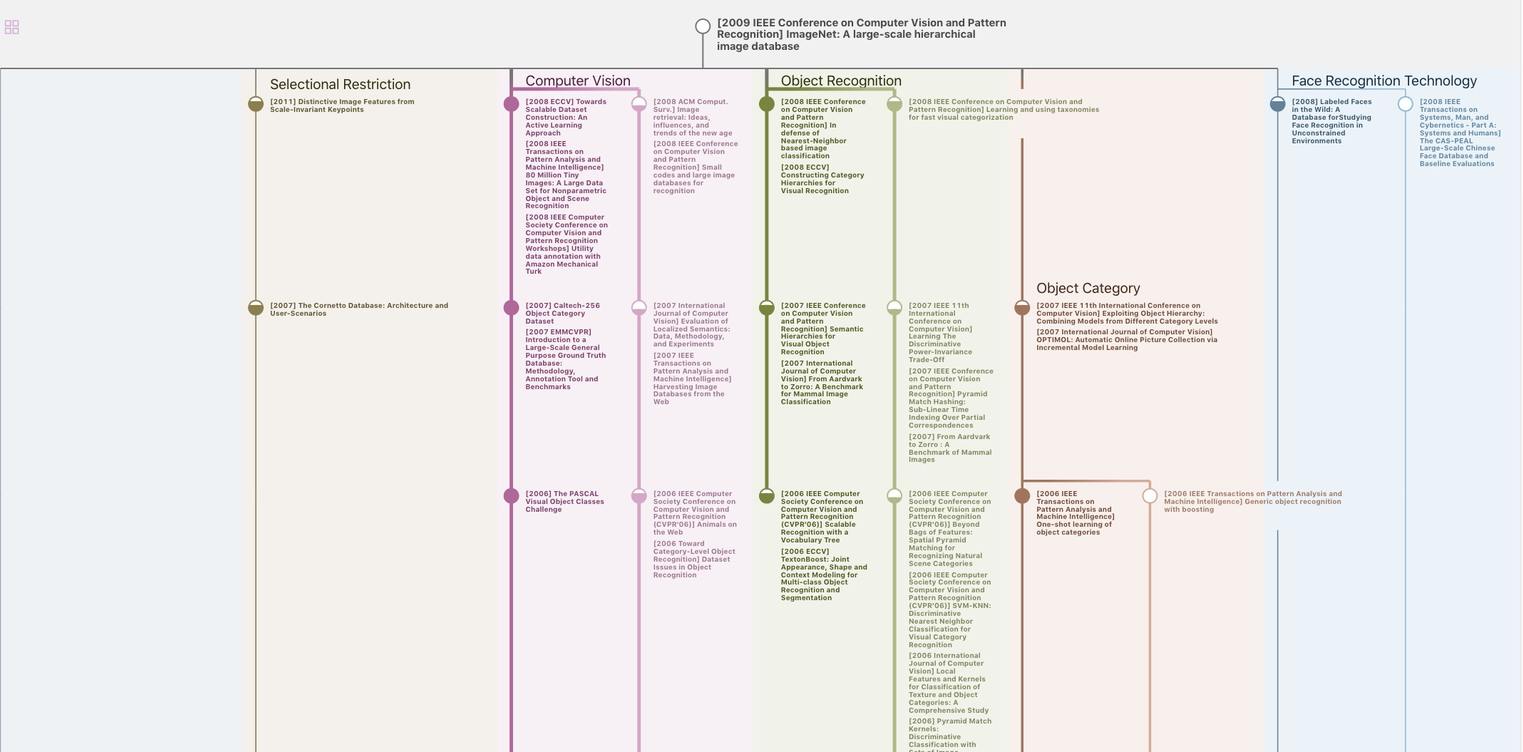
生成溯源树,研究论文发展脉络
Chat Paper
正在生成论文摘要